Robustness of model predictions under extension.
International Conference on Uncertainty in Artificial Intelligence(2022)
摘要
Mathematical models of the real world are simplified representations of complex systems. A caveat to using mathematical models is that predicted causal effects and conditional independences may not be robust under model extensions, limiting applicability of such models. In this work, we consider conditions under which qualitative model predictions are preserved when two models are combined. Under mild assumptions, we show how to use the technique of causal ordering to efficiently assess the robustness of qualitative model predictions. We also characterize a large class of model extensions that preserve qualitative model predictions. For dynamical systems at equilibrium, we demonstrate how novel insights help to select appropriate model extensions and to reason about the presence of feedback loops. We illustrate our ideas with a viral infection model with immune responses.
更多查看译文
关键词
model predictions,extension
AI 理解论文
溯源树
样例
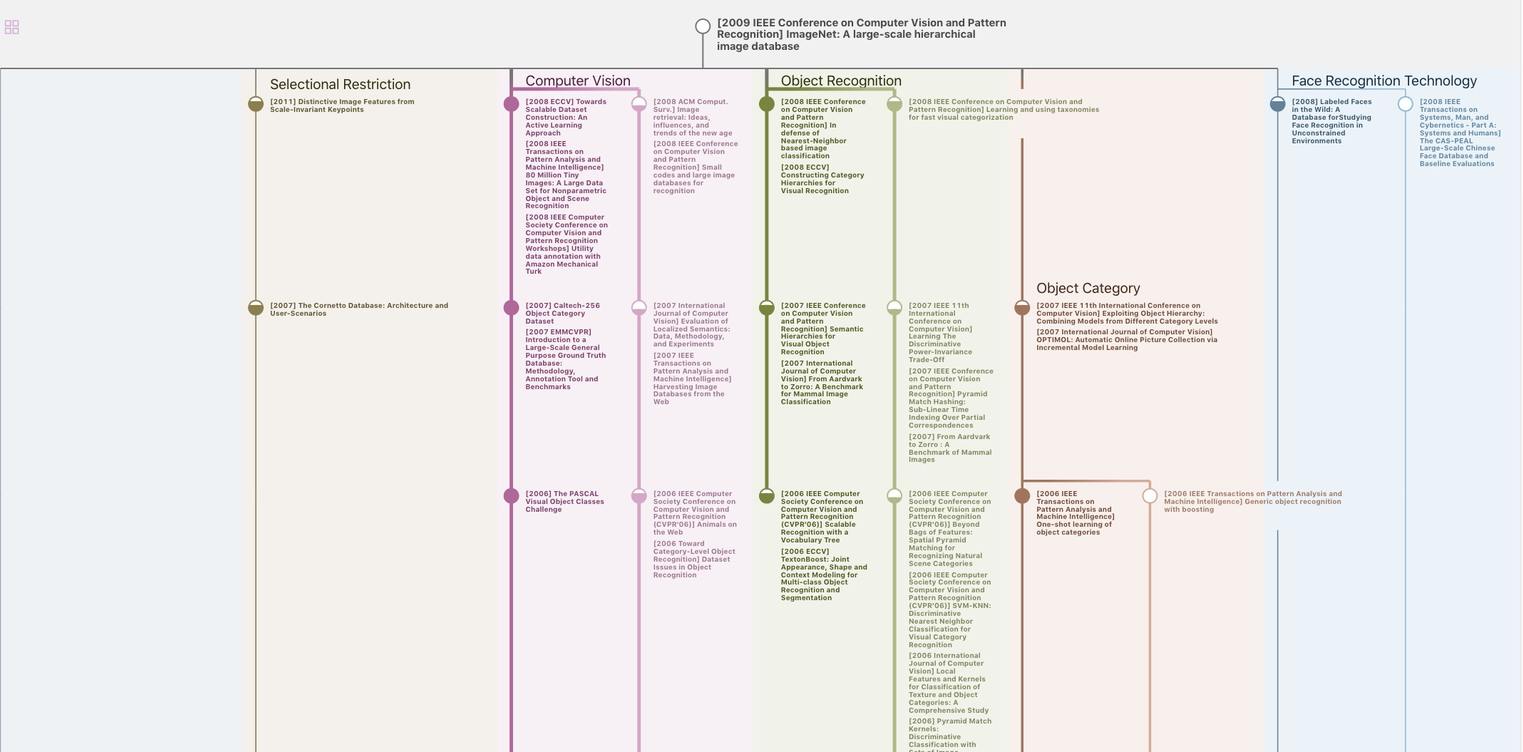
生成溯源树,研究论文发展脉络
Chat Paper
正在生成论文摘要