Towards Communication-Efficient and Attack-Resistant Federated Edge Learning for Industrial Internet of Things
ACM Transactions on Internet Technology(2022)
摘要
AbstractFederated Edge Learning (FEL) allows edge nodes to train a global deep learning model collaboratively for edge computing in the Industrial Internet of Things (IIoT), which significantly promotes the development of Industrial 4.0. However, FEL faces two critical challenges: communication overhead and data privacy. FEL suffers from expensive communication overhead when training large-scale multi-node models. Furthermore, due to the vulnerability of FEL to gradient leakage and label-flipping attacks, the training process of the global model is easily compromised by adversaries. To address these challenges, we propose a communication-efficient and privacy-enhanced asynchronous FEL framework for edge computing in IIoT. First, we introduce an asynchronous model update scheme to reduce the computation time that edge nodes wait for global model aggregation. Second, we propose an asynchronous local differential privacy mechanism, which improves communication efficiency and mitigates gradient leakage attacks by adding well-designed noise to the gradients of edge nodes. Third, we design a cloud-side malicious node detection mechanism to detect malicious nodes by testing the local model quality. Such a mechanism can avoid malicious nodes participating in training to mitigate label-flipping attacks. Extensive experimental studies on two real-world datasets demonstrate that the proposed framework can not only improve communication efficiency but also mitigate malicious attacks while its accuracy is comparable to traditional FEL frameworks.
更多查看译文
关键词
Federated edge learning, edge intelligence, local differential privacy, gradient leakage attack, poisoning attack
AI 理解论文
溯源树
样例
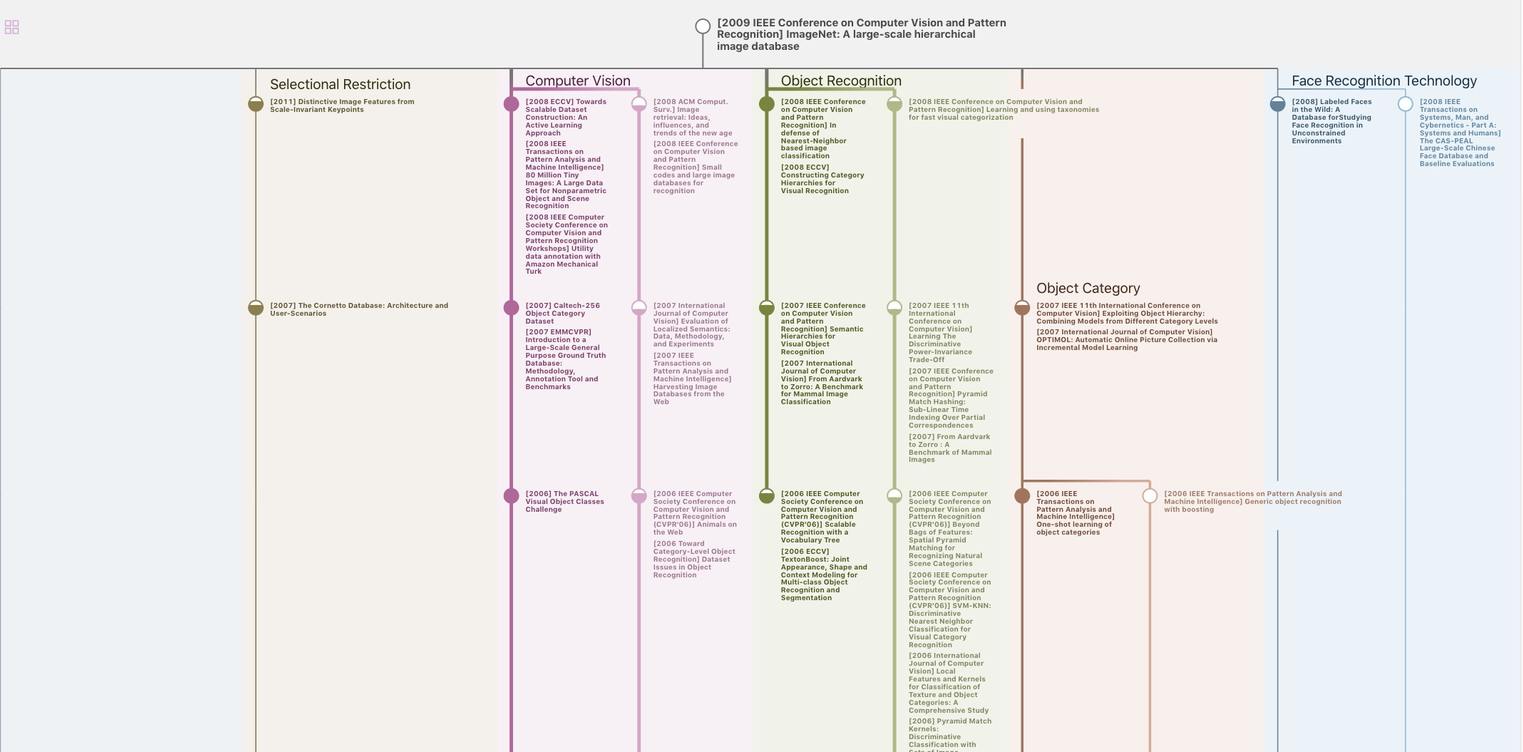
生成溯源树,研究论文发展脉络
Chat Paper
正在生成论文摘要