An Empirical Study of Explainable AI Techniques on Deep Learning Models For Time Series Tasks
arxiv(2020)
摘要
Decision explanations of machine learning black-box models are often generated by applying Explainable AI (XAI) techniques. However, many proposed XAI methods produce unverified outputs. Evaluation and verification are usually achieved with a visual interpretation by humans on individual images or text. In this preregistration, we propose an empirical study and benchmark framework to apply attribution methods for neural networks developed for images and text data on time series. We present a methodology to automatically evaluate and rank attribution techniques on time series using perturbation methods to identify reliable approaches.
更多查看译文
关键词
explainable explainable techniques,deep learning models
AI 理解论文
溯源树
样例
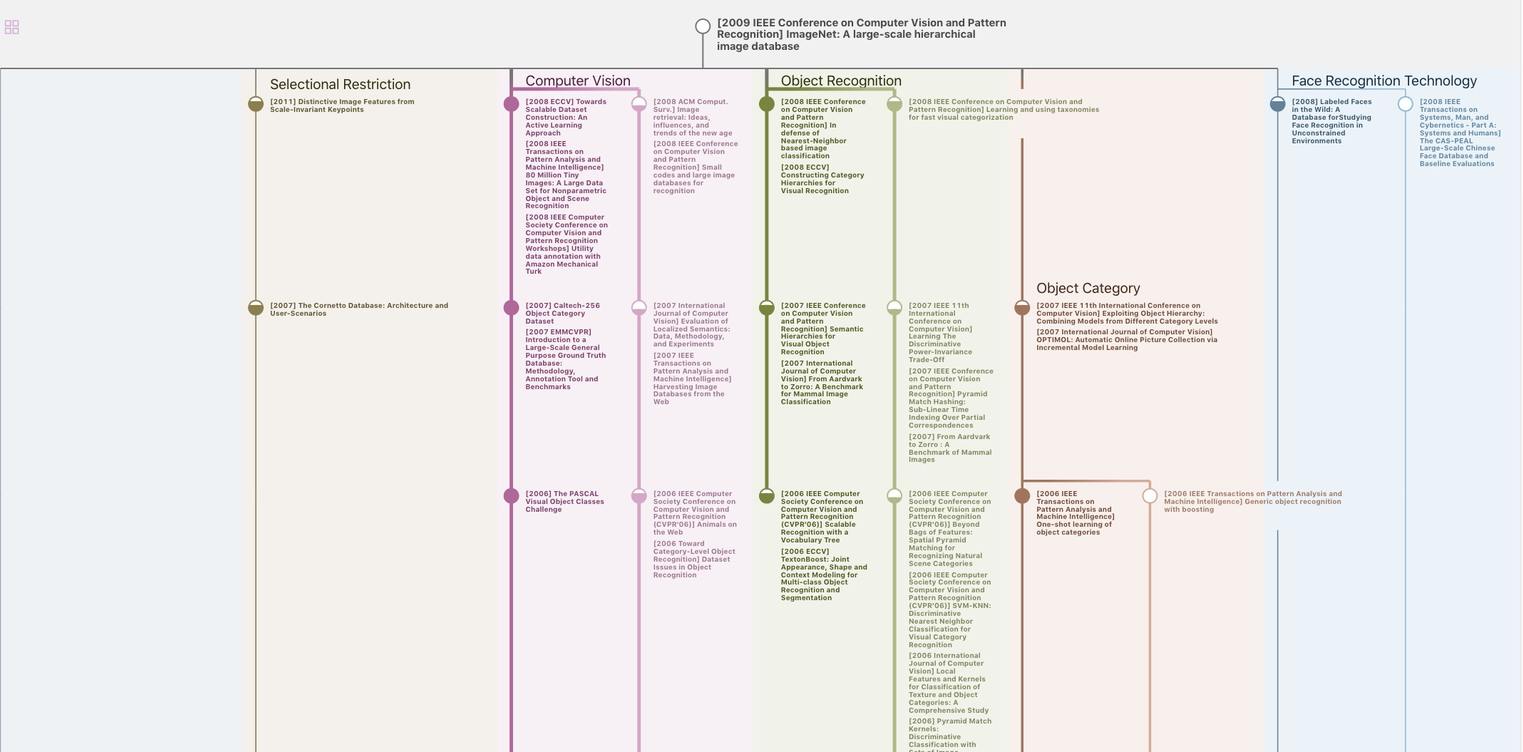
生成溯源树,研究论文发展脉络
Chat Paper
正在生成论文摘要