Robust sparse Bayesian infinite factor models
Computational Statistics(2022)
摘要
Most of previous works and applications of Bayesian factor model have assumed the normal likelihood regardless of its validity. We propose a Bayesian factor model for heavy-tailed high-dimensional data based on multivariate Student- t likelihood to obtain better covariance estimation. We use multiplicative gamma process shrinkage prior and factor number adaptation scheme proposed in Bhattacharya and Dunson [Biometrika 98(2):291–306, 2011]. Since a naive Gibbs sampler for the proposed model suffers from slow mixing, we propose a Markov Chain Monte Carlo algorithm where fast mixing of Hamiltonian Monte Carlo is exploited for some parameters in the proposed model. Simulation results illustrate the gain in performance of covariance estimation for heavy-tailed high-dimensional data. We also provide a theoretical result that the posterior of the proposed model is weakly consistent under reasonable conditions. We conclude the paper with the application of the proposed factor model on breast cancer metastasis prediction given DNA signature data of cancer cells.
更多查看译文
关键词
Bayesian modeling, Covariance estimation, Factor model, Multiplicative gamma process prior, Multivariate t-distribution
AI 理解论文
溯源树
样例
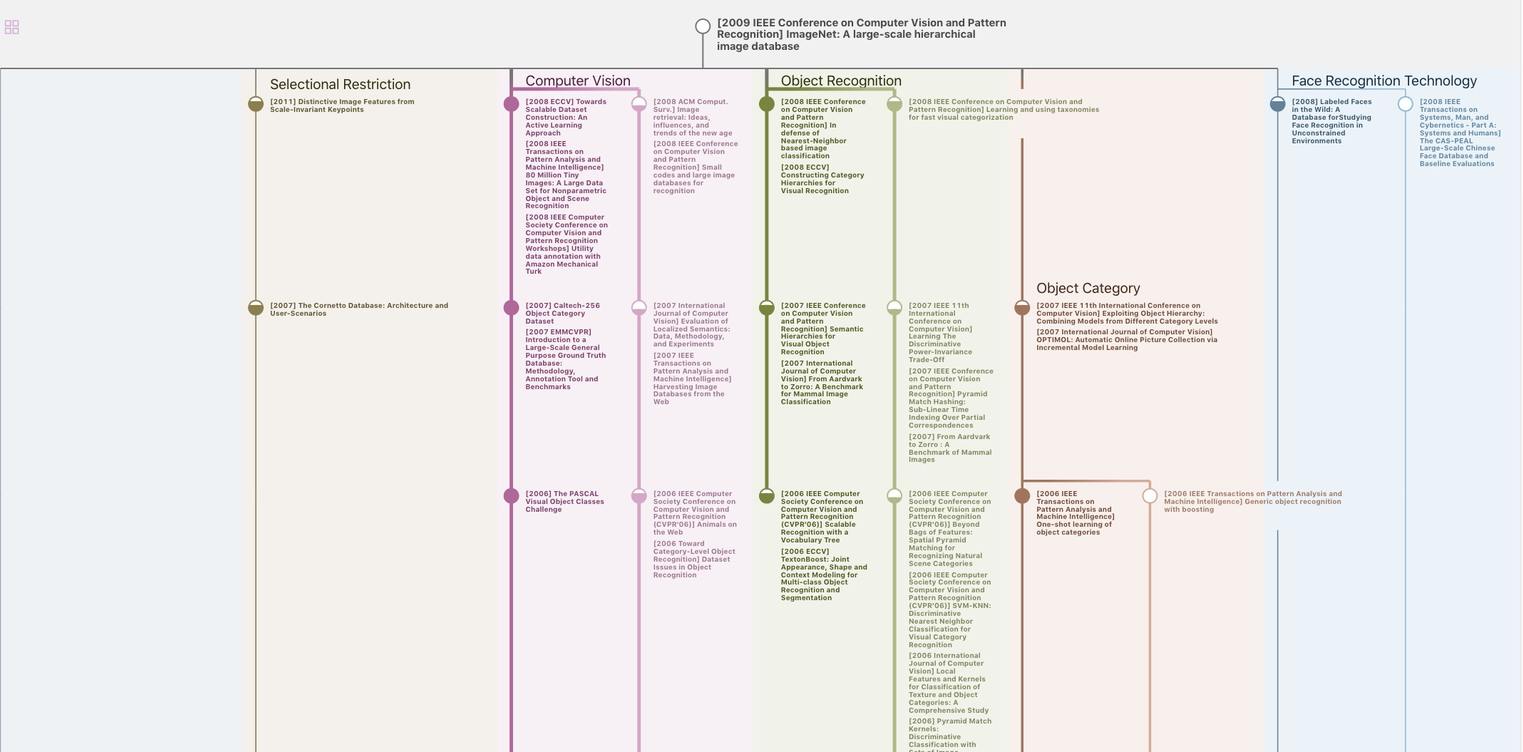
生成溯源树,研究论文发展脉络
Chat Paper
正在生成论文摘要