Neural fidelity warping for efficient robot morphology design
2021 IEEE INTERNATIONAL CONFERENCE ON ROBOTICS AND AUTOMATION (ICRA 2021)(2021)
摘要
We consider the problem of optimizing a robot morphology to achieve the best performance for a target task, under computational resource limitations. The evaluation process for each morphological design involves learning a controller for the design, which can consume substantial time and computational resources. To address the challenge of expensive robot morphology evaluation, we present a continuous multi-fidelity Bayesian Optimization framework that efficiently utilizes computational resources via low-fidelity evaluations. We identify the problem of non-stationarity over fidelity space. Our proposed fidelity warping mechanism can learn representations of learning epochs and tasks to model non-stationary covariances between continuous fidelity evaluations which prove challenging for off-the-shelf stationary kernels. Various experiments demonstrate that our method can utilize the low-fidelity evaluations to efficiently search for the optimal robot morphology, outperforming state-of-the-art methods.
更多查看译文
关键词
neural fidelity,morphology,robot
AI 理解论文
溯源树
样例
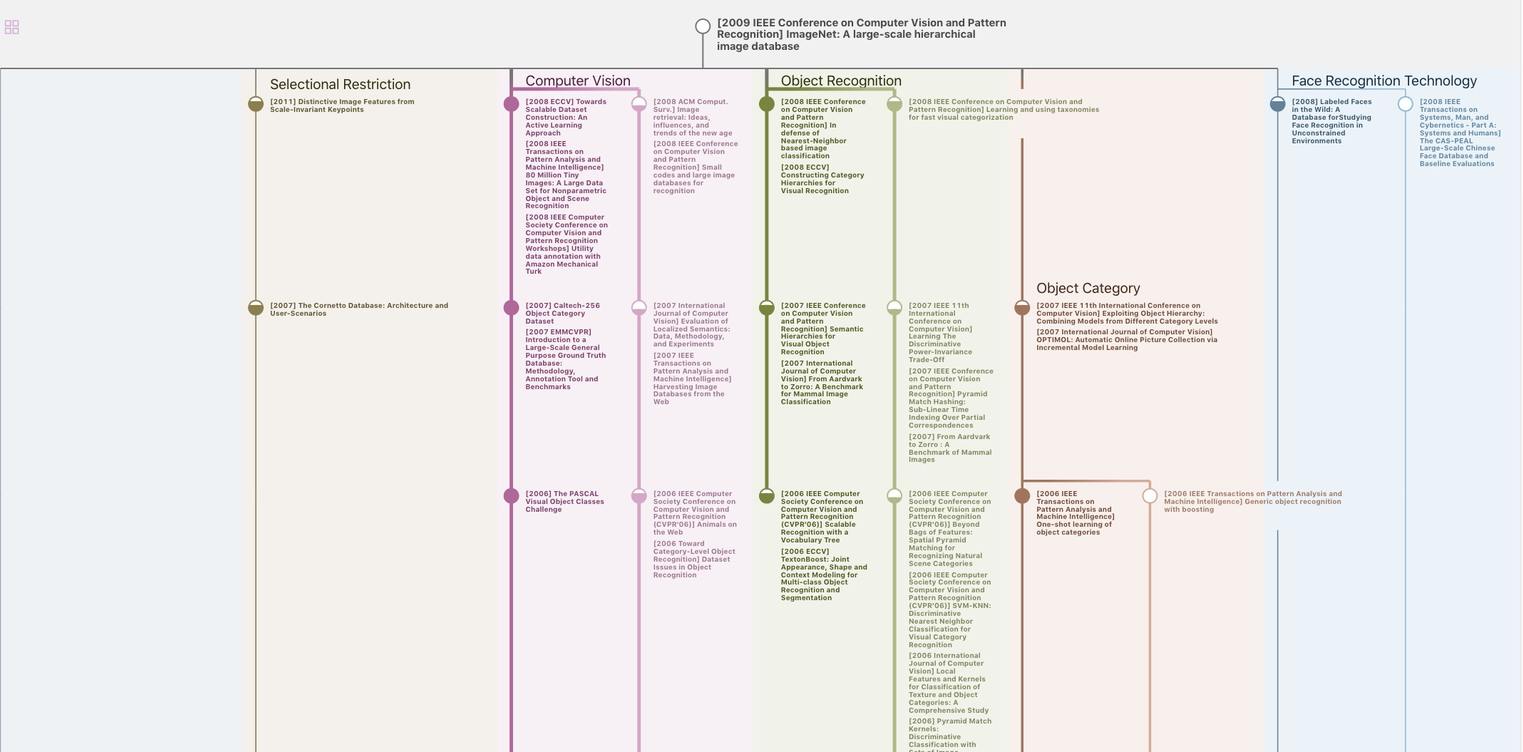
生成溯源树,研究论文发展脉络
Chat Paper
正在生成论文摘要