A Differential Evolution-Based Consistency Improvement Method in AHP With an Optimal Allocation of Information Granularity
IEEE Transactions on Cybernetics(2022)
摘要
In the analytic hierarchy process (AHP), the reciprocal matrix is generated based on the pairwise comparisons completed among all the alternatives or attributes under consideration. To ensure reliability and validity of the decision solution, a certain modification of entries of the matrix is usually needed to improve the consistency of the reciprocal matrix. This study aims to present a consistency improvement method by admitting some level of information granularity in the evaluation process. This gives rise to a granular rather than numeric matrix of pairwise comparisons. First, with a given average level of information granularity, we present an optimal granularity model that is characterized by maximal consistency. One can maximize the consistency degree by invoking a process of allocation of information granularity across the corresponding modifications of the reciprocal matrix. Based on the optimal granularity model, an interactive consistency improvement process is presented with the involvement of the decision maker. Then, an adaptive differential evolution algorithm is applied to optimize entries of the modified reciprocal matrix. Detailed experiments along with a thorough comparative analysis are completed to demonstrate the effectiveness of the proposed method.
更多查看译文
关键词
Algorithms,Analytic Hierarchy Process,Reproducibility of Results
AI 理解论文
溯源树
样例
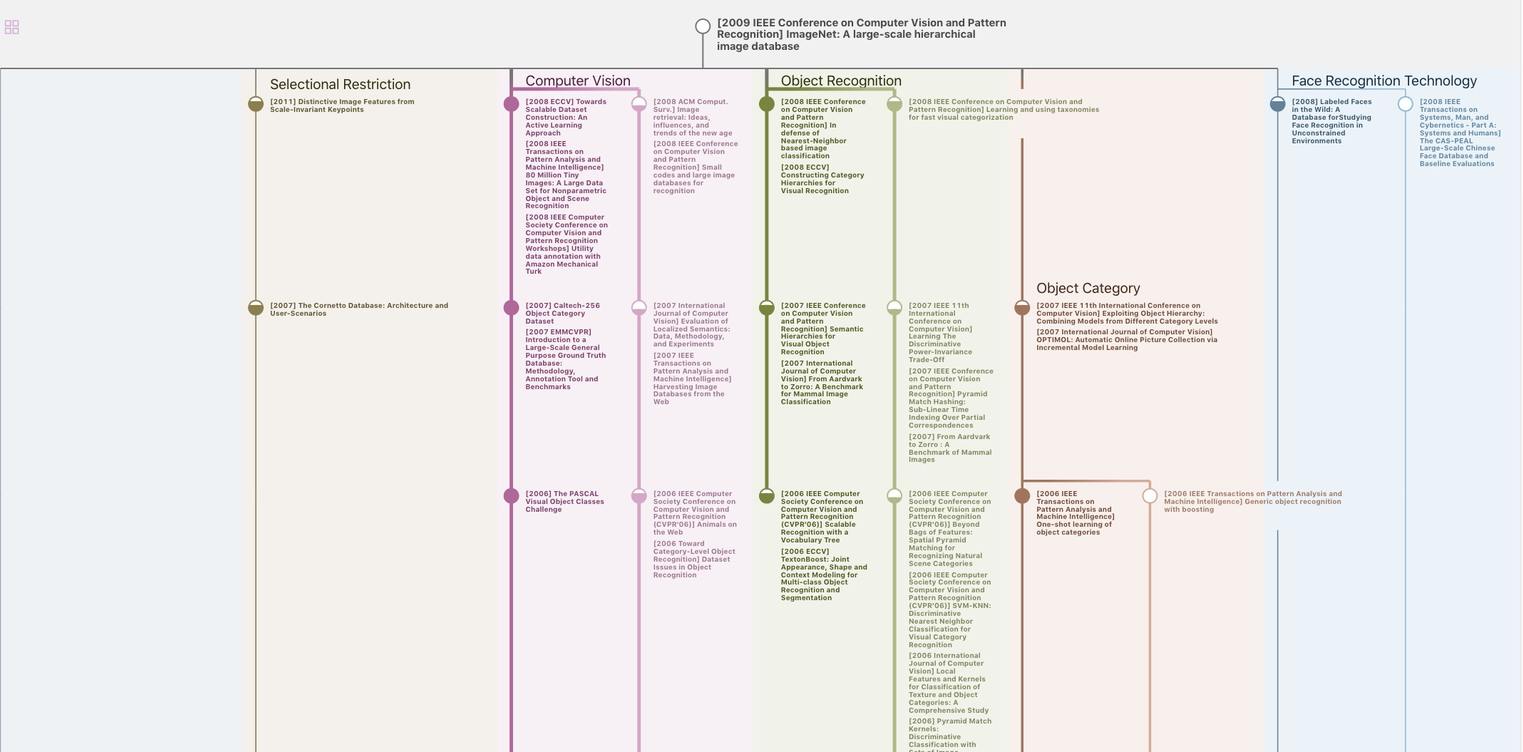
生成溯源树,研究论文发展脉络
Chat Paper
正在生成论文摘要