Generalizable brain network markers of major depressive disorder across multiple imaging sites.
PLOS BIOLOGY(2020)
摘要
Many studies have highlighted the difficulty inherent to the clinical application of fundamental neuroscience knowledge based on machine learning techniques. It is difficult to generalize machine learning brain markers to the data acquired from independent imaging sites, mainly due to large site differences in functional magnetic resonance imaging. We address the difficulty of finding a generalizable marker of major depressive disorder (MDD) that would distinguish patients from healthy controls based on resting-state functional connectivity patterns. For the discovery dataset with 713 participants from 4 imaging sites, we removed site differences using our recently developed harmonization method and developed a machine learning MDD classifier. The classifier achieved an approximately 70% generalization accuracy for an independent validation dataset with 521 participants from 5 different imaging sites. The successful generalization to a perfectly independent dataset acquired from multiple imaging sites is novel and ensures scientific reproducibility and clinical applicability.
更多查看译文
关键词
generalizable brain network markers,major depressive disorder,depressive disorder,imaging
AI 理解论文
溯源树
样例
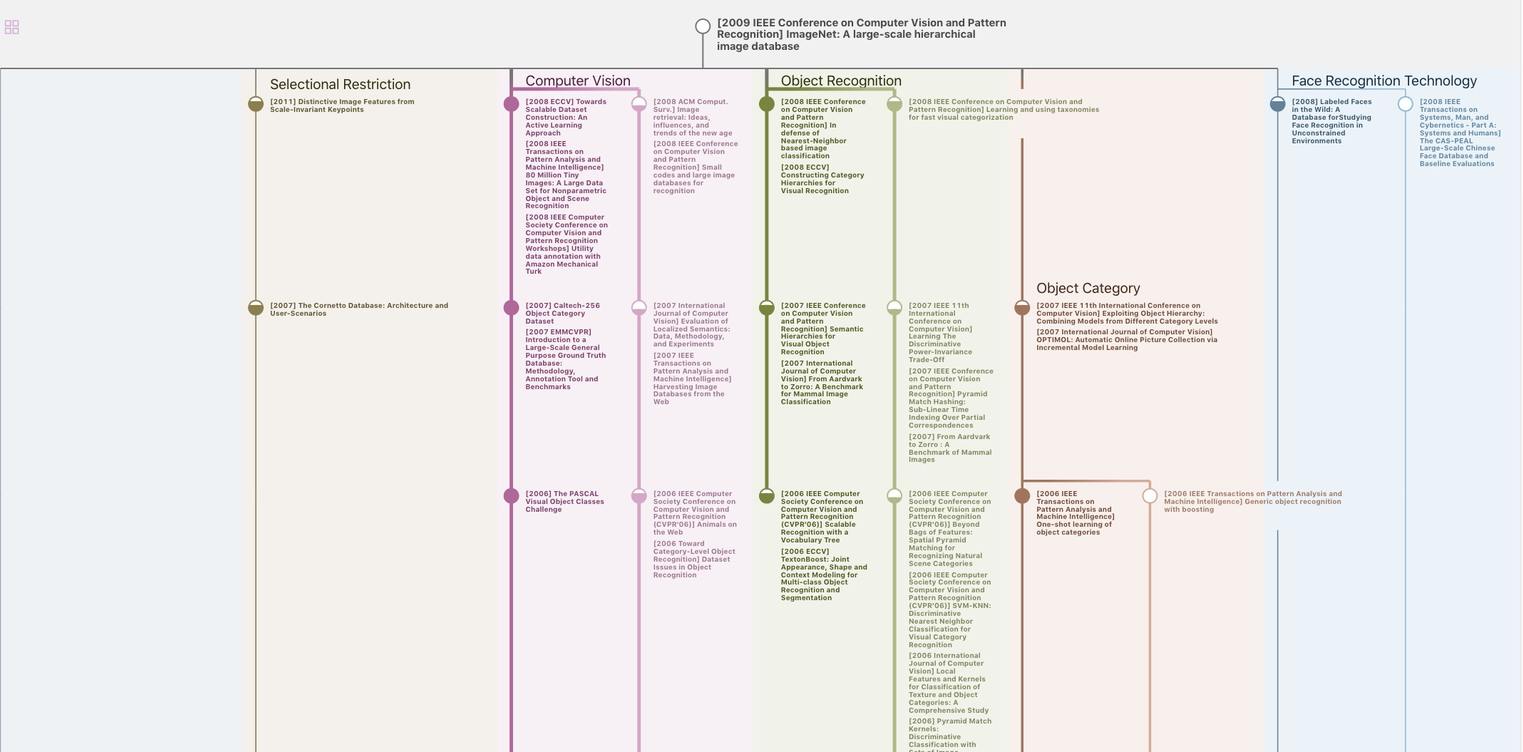
生成溯源树,研究论文发展脉络
Chat Paper
正在生成论文摘要