A Differential Evolution Algorithm With Adaptive Niching and K -Means Operation for Data Clustering
IEEE Transactions on Cybernetics(2022)
摘要
Clustering, as an important part of data mining, is inherently a challenging problem. This article proposes a differential evolution algorithm with adaptive niching and
${k}$
-means operation (denoted as DE_ANS_AKO) for partitional data clustering. Within the proposed algorithm, an adaptive niching scheme, which can dynamically adjust the size of each niche in the population, is devised and integrated to prevent premature convergence of evolutionary search, thus appropriately searching the space to identify the optimal or near-optimal solution. Furthermore, to improve the search efficiency, an adaptive
${k}$
-means operation has been designed and employed at the niche level of population. The performance of the proposed algorithm has been evaluated on synthetic as well as real datasets and compared with related methods. The experimental results reveal that the proposed algorithm is able to reliably and efficiently deliver high quality clustering solutions and generally outperforms related methods implemented for comparisons.
更多查看译文
关键词
Algorithms,Cluster Analysis,Computer Simulation,Data Mining
AI 理解论文
溯源树
样例
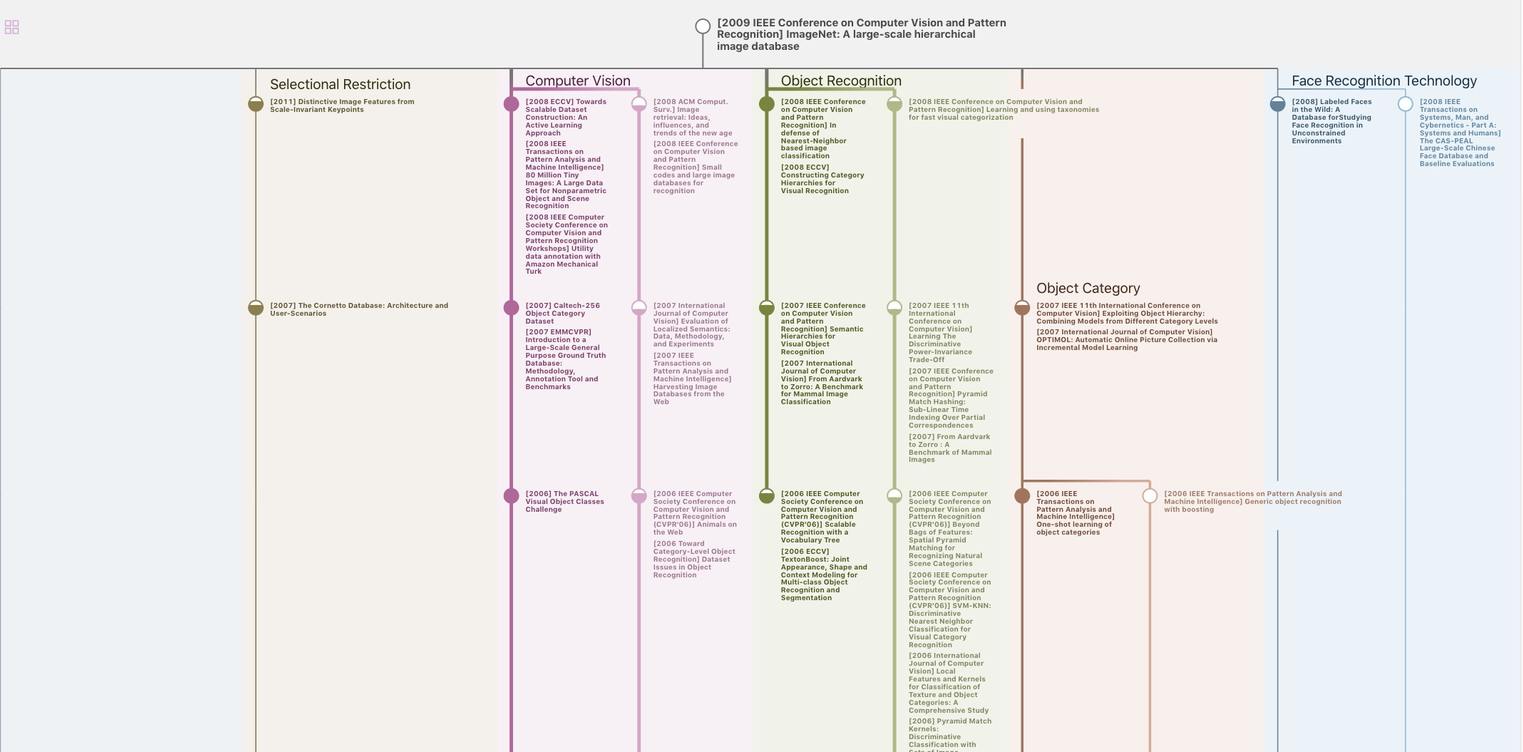
生成溯源树,研究论文发展脉络
Chat Paper
正在生成论文摘要