Frame-Level Specaugment for Deep Convolutional Neural Networks in Hybrid ASR Systems
2021 IEEE Spoken Language Technology Workshop (SLT)(2021)
摘要
Inspired by SpecAugment - a data augmentation method for end-to-end ASR systems, we propose a frame-level SpecAugment method (f-SpecAugment) to improve the performance of deep convolutional neural networks (CNN) for hybrid HMM based ASR systems. Similar to the utterance level SpecAugment, f-SpecAugment performs three transformations: time warping, frequency masking, and time masking. Instead of applying the transformations at the utterance level, f-SpecAugment applies them to each convolution window independently during training. We demonstrate that f-SpecAugment is more effective than the utterance level SpecAugment for deep CNN based hybrid models. We evaluate the proposed f-SpecAugment on 50-layer Self-Normalizing Deep CNN (SNDCNN) acoustic models trained with up to 25000 hours of training data. We observe f-SpecAugment reduces WER by 0.5-4.5% relatively across different ASR tasks for four languages. As the benefits of augmentation techniques tend to diminish as training data size increases, the large scale training reported is important in understanding the effectiveness of f-SpecAugment. Our experiments demonstrate that even with 25k training data, f-SpecAugment is still effective. We also demonstrate that f-SpecAugment has benefits approximately equivalent to doubling the amount of training data for deep CNNs.
更多查看译文
关键词
speech recognition,frame-level SpecAugment,SNDCNN,data augmentation,hybrid ASR system
AI 理解论文
溯源树
样例
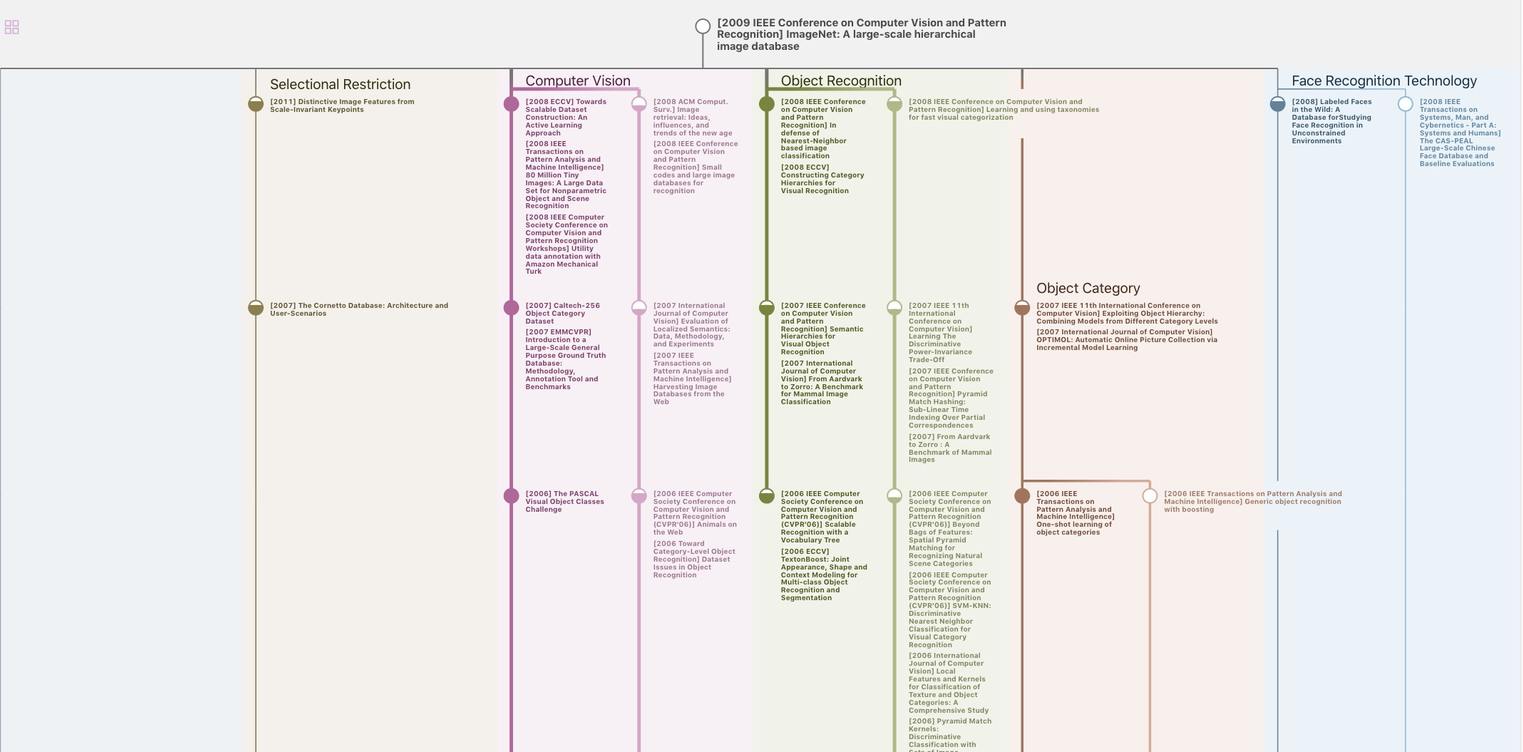
生成溯源树,研究论文发展脉络
Chat Paper
正在生成论文摘要