HYPERBOLIC RELAXATION OF k-LOCALLY POSITIVE SEMIDEFINITE MATRICES
SIAM JOURNAL ON OPTIMIZATION(2022)
摘要
A successful computational approach for solving large-scale positive semidefinite (PSD) programs is to enforce PSD-ness on only a collection of submatrices. For our study, we let Sn,k be the convex cone of n x n symmetric matrices where all k x k principal submatrices matrices, we study eigenvalues of k-locally PSD matrices. The key insight in this paper is that there is a convex cone H(enk) so that if X E Sn,k, then the vector of eigenvalues of X is contained in H(enk ). The cone H(enk) is the hyperbolicity cone of the elementary symmetric polynomial en \prod k (where enk (x) = \sum Sc[n]:ISI=k iES xi) with respect to the all ones vector. Using this insight, we are able to improve previously known upper bounds on the Frobenius distance between matrices in Sn,k and PSD matrices. We also study the quality of the convex relaxation H(enk ). We first show that this relaxation is tight for the case of k = n-1, that is, for every vector in H(enn-1) there exists a matrix in Sn,n-1 whose eigenvalues are equal to the components of the vector. We then prove a structure theorem on nonsingular matrices in Sn,k all of whose k x k principal minors are zero, which we believe is of independent interest. This result shows shows that for 1 < k < n - 1 ``large parts"" of the boundary of H(enk) do not intersect with the eigenvalues of matrices in Sn,k.
更多查看译文
关键词
hyperbolicity cone,positive semidefinite matrix,eigenvalue bounds
AI 理解论文
溯源树
样例
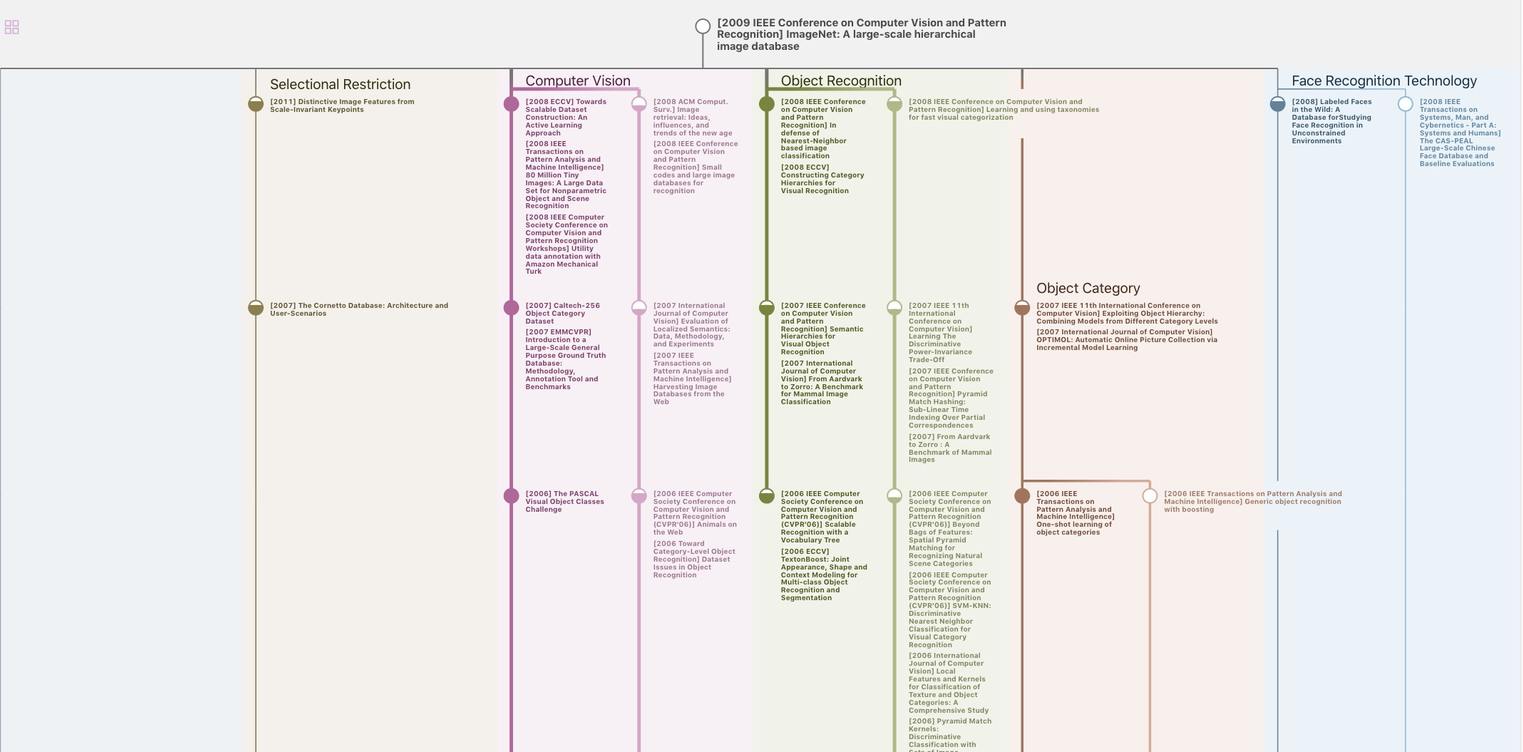
生成溯源树,研究论文发展脉络
Chat Paper
正在生成论文摘要