Dynamic Clustering in Federated Learning
IEEE INTERNATIONAL CONFERENCE ON COMMUNICATIONS (ICC 2021)(2021)
摘要
In the resource management of wireless networks, Federated Learning has been used to predict handovers. However, non-independent and identically distributed data degrade the accuracy performance of such predictions. To overcome the problem, Federated Learning can leverage data clustering algorithms and build a machine learning model for each cluster. However, traditional data clustering algorithms, when applied to the handover prediction, exhibit three main limitations: the risk of data privacy breach, the fixed shape of clusters, and the non-adaptive number of clusters. To overcome these limitations, in this paper, we propose a three-phased data clustering algorithm, namely: generative adversarial network-based clustering, cluster calibration, and cluster division. We show that the generative adversarial network-based clustering preserves privacy. The cluster calibration deals with dynamic environments by modifying clusters. Moreover, the divisive clustering explores the different number of clusters by repeatedly selecting and dividing a cluster into multiple clusters. A baseline algorithm and our algorithm are tested on a time series forecasting task. We show that our algorithm improves the performance of forecasting models, including cellular network handover, by 43%.
更多查看译文
关键词
clustering, Federated Learning, GAN, non-HD, handover prediction
AI 理解论文
溯源树
样例
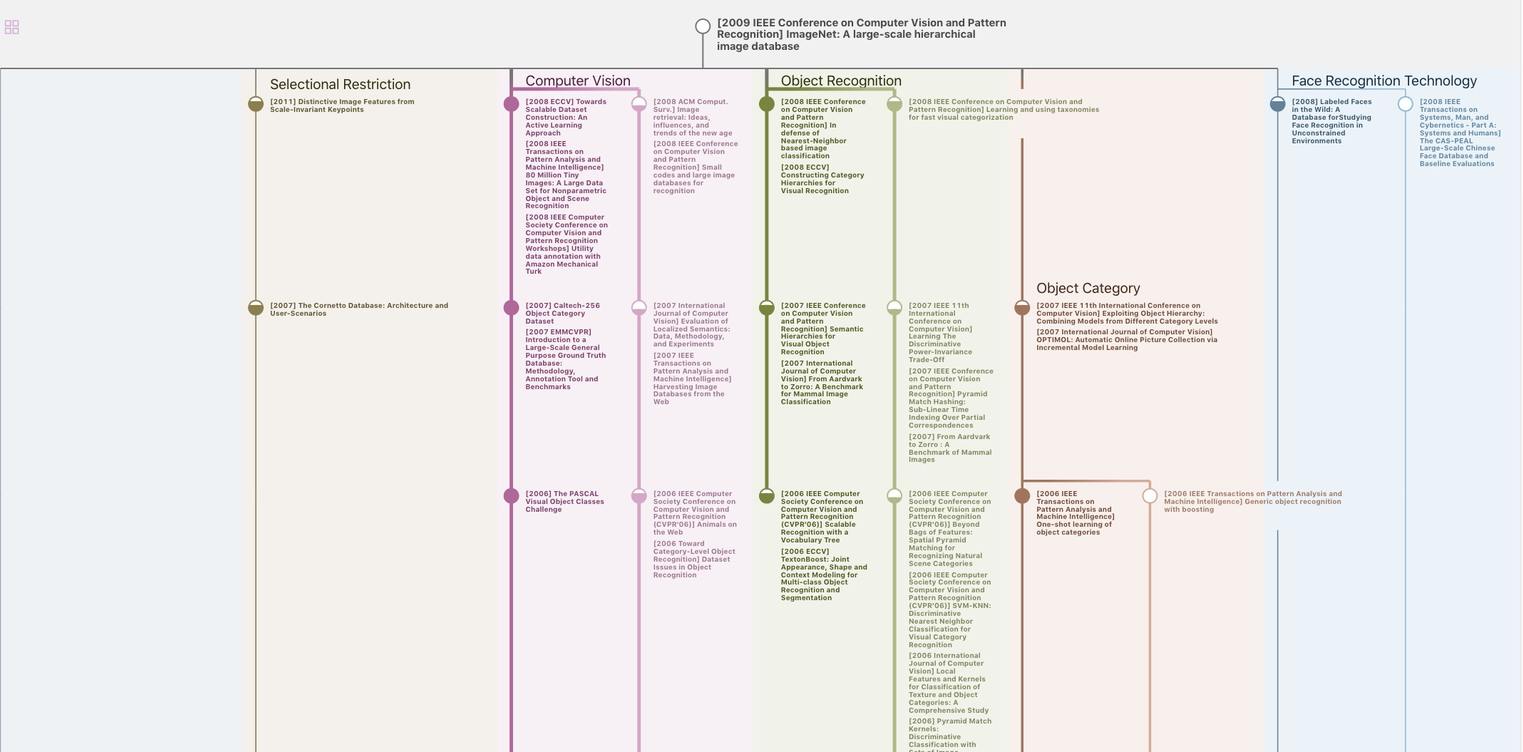
生成溯源树,研究论文发展脉络
Chat Paper
正在生成论文摘要