Perceptual Error Optimization for Monte Carlo Rendering
ACM Transactions on Graphics(2022)
摘要
AbstractSynthesizing realistic images involves computing high-dimensional light-transport integrals. In practice, these integrals are numerically estimated via Monte Carlo integration. The error of this estimation manifests itself as conspicuous aliasing or noise. To ameliorate such artifacts and improve image fidelity, we propose a perception-oriented framework to optimize the error of Monte Carlo rendering. We leverage models based on human perception from the halftoning literature. The result is an optimization problem whose solution distributes the error as visually pleasing blue noise in image space. To find solutions, we present a set of algorithms that provide varying trade-offs between quality and speed, showing substantial improvements over prior state of the art. We perform evaluations using quantitative and error metrics and provide extensive supplemental material to demonstrate the perceptual improvements achieved by our methods.
更多查看译文
关键词
Monte Carlo, rendering, sampling, perceptual error, blue noise, halftoning, dithering, error diffusion
AI 理解论文
溯源树
样例
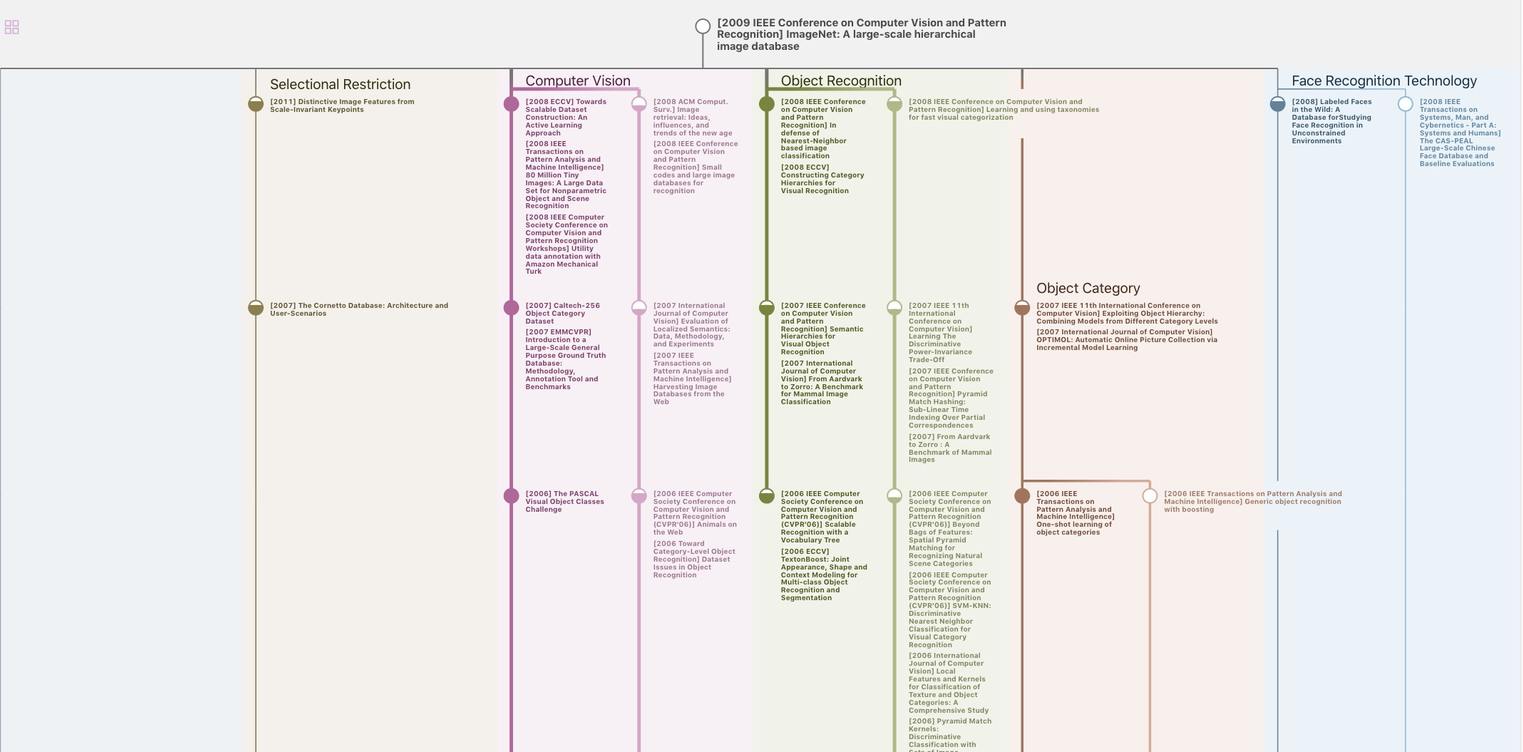
生成溯源树,研究论文发展脉络
Chat Paper
正在生成论文摘要