Mask-Guided Attention Network And Occlusion-Sensitive Hard Example Mining For Occluded Pedestrian Detection
IEEE TRANSACTIONS ON IMAGE PROCESSING(2021)
摘要
Pedestrian detection relying on deep convolution neural networks has made significant progress. Though promising results have been achieved on standard pedestrians, the performance on heavily occluded pedestrians remains far from satisfactory. The main culprits are intra-class occlusions involving other pedestrians and inter-class occlusions caused by other objects, such as cars and bicycles. These result in a multitude of occlusion patterns. We propose an approach for occluded pedestrian detection with the following contributions. First, we introduce a novel mask-guided attention network that fits naturally into popular pedestrian detection pipelines. Our attention network emphasizes on visible pedestrian regions while suppressing the occluded ones by modulating full body features. Second, we propose the occlusion-sensitive hard example mining method and occlusion-sensitive loss that mines hard samples according to the occlusion level and assigns higher weights to the detection errors occurring at highly occluded pedestrians. Third, we empirically demonstrate that weak box-based segmentation annotations provide reasonable approximation to their dense pixel-wise counterparts. Experiments are performed on CityPersons, Caltech and ETH datasets. Our approach sets a new state-of-the-art on all three datasets. Our approach obtains an absolute gain of 10.3% in log-average miss rate, compared with the best reported results on the heavily occluded HO pedestrian set of the CityPersons test set. Code and models are available at: https://github.com/Leotju/MGAN.
更多查看译文
关键词
Detectors, Standards, Feature extraction, Proposals, Computer architecture, Benchmark testing, Task analysis, Pedestrian detection, attention, convolutional neural networks, hard example mining
AI 理解论文
溯源树
样例
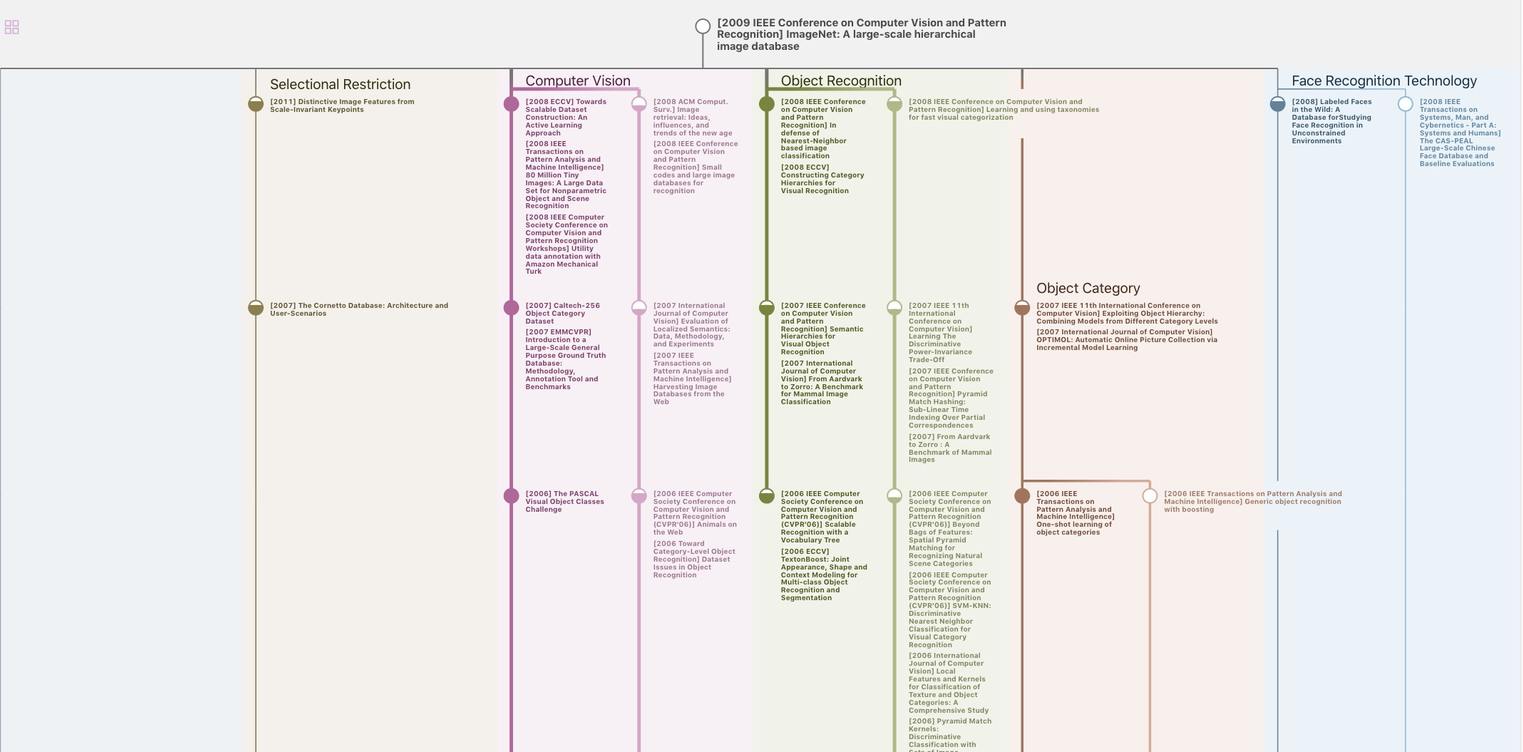
生成溯源树,研究论文发展脉络
Chat Paper
正在生成论文摘要