Discriminatory Expressions to Improve Model Comprehensibility in Short Documents.
International Conferences on Pattern Recognition and Artificial Intelligence (ICPRAI)(2022)
摘要
Social Networking Sites (SNS) are one of the most important ways of communication. In particular, microblogging sites are being used as analysis avenues due to their peculiarities (promptness, short texts...). There are countless researches that use SNS in novel manners, but machine learning has focused mainly in classification performance rather than interpretability and/or other goodness metrics. Thus, state-of-the-art models are black boxes that should not be used to solve problems that may have a social impact. When the problem requires transparency, it is necessary to build interpretable pipelines. Although the classifier may be interpretable, resulting models are too complex to be considered comprehensible, making it impossible for humans to understand the actual decisions. This paper presents a feature selection mechanism that is able to improve comprehensibility by using less but more meaningful features while achieving good performance in microblogging contexts where interpretability is mandatory. Moreover, we present a ranking method to evaluate features in terms of statistical relevance and bias. We conducted exhaustive tests with five different datasets in order to evaluate classification performance, generalisation capacity and complexity of the model. Results show that our proposal is better and the most stable one in terms of accuracy, generalisation and comprehensibility.
更多查看译文
关键词
model comprehensibility,expressions
AI 理解论文
溯源树
样例
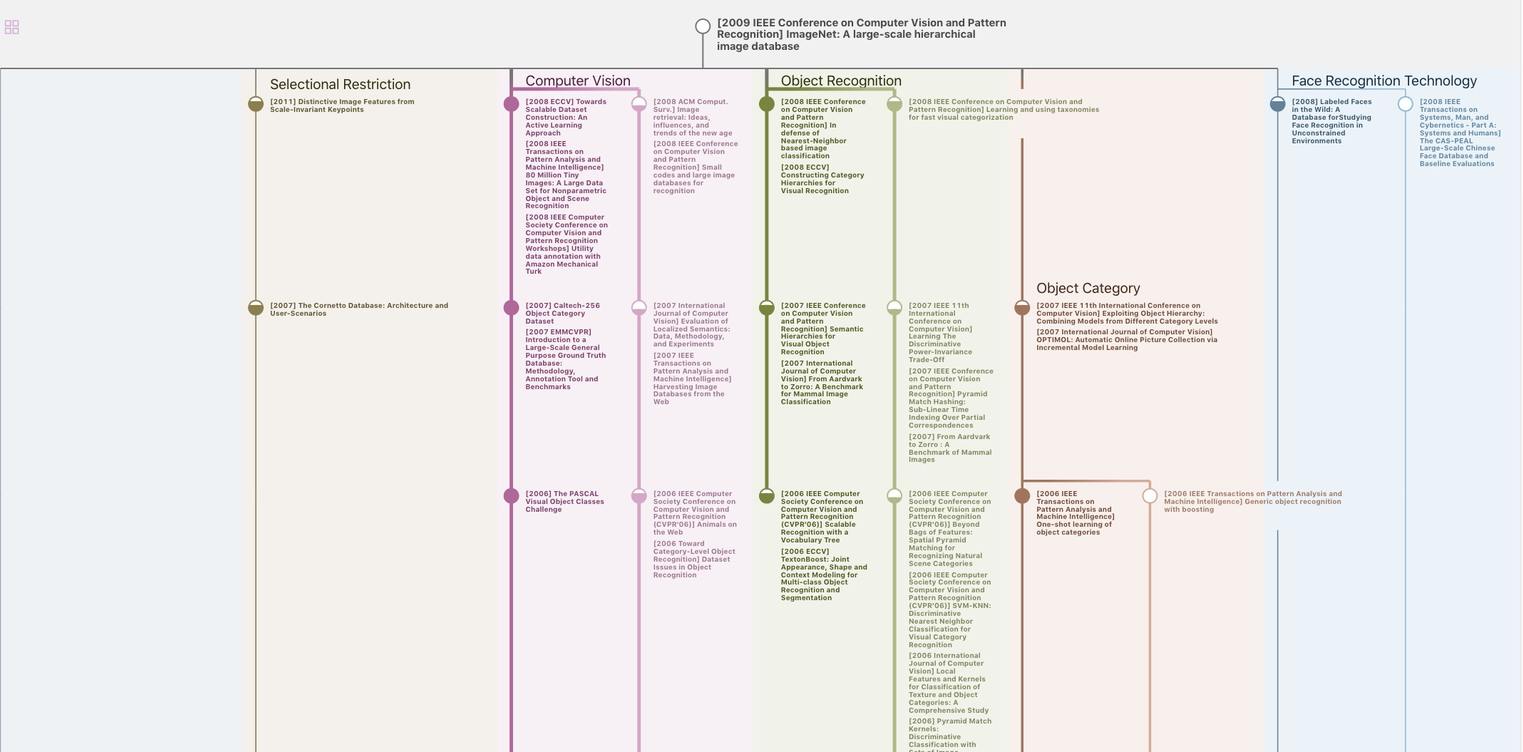
生成溯源树,研究论文发展脉络
Chat Paper
正在生成论文摘要