DeepCrawl: Deep Reinforcement Learning for Turn-based Strategy Games
arxiv(2020)
摘要
In this paper we introduce DeepCrawl, a fully-playable Roguelike prototype for iOS and Android in which all agents are controlled by policy networks trained using Deep Reinforcement Learning (DRL). Our aim is to understand whether recent advances in DRL can be used to develop convincing behavioral models for non-player characters in videogames. We begin with an analysis of requirements that such an AI system should satisfy in order to be practically applicable in video game development, and identify the elements of the DRL model used in the DeepCrawl prototype. The successes and limitations of DeepCrawl are documented through a series of playability tests performed on the final game. We believe that the techniques we propose offer insight into innovative new avenues for the development of behaviors for non-player characters in video games, as they offer the potential to overcome critical issues with
更多查看译文
关键词
deepcrawl reinforcement learning,strategy,games,turn-based
AI 理解论文
溯源树
样例
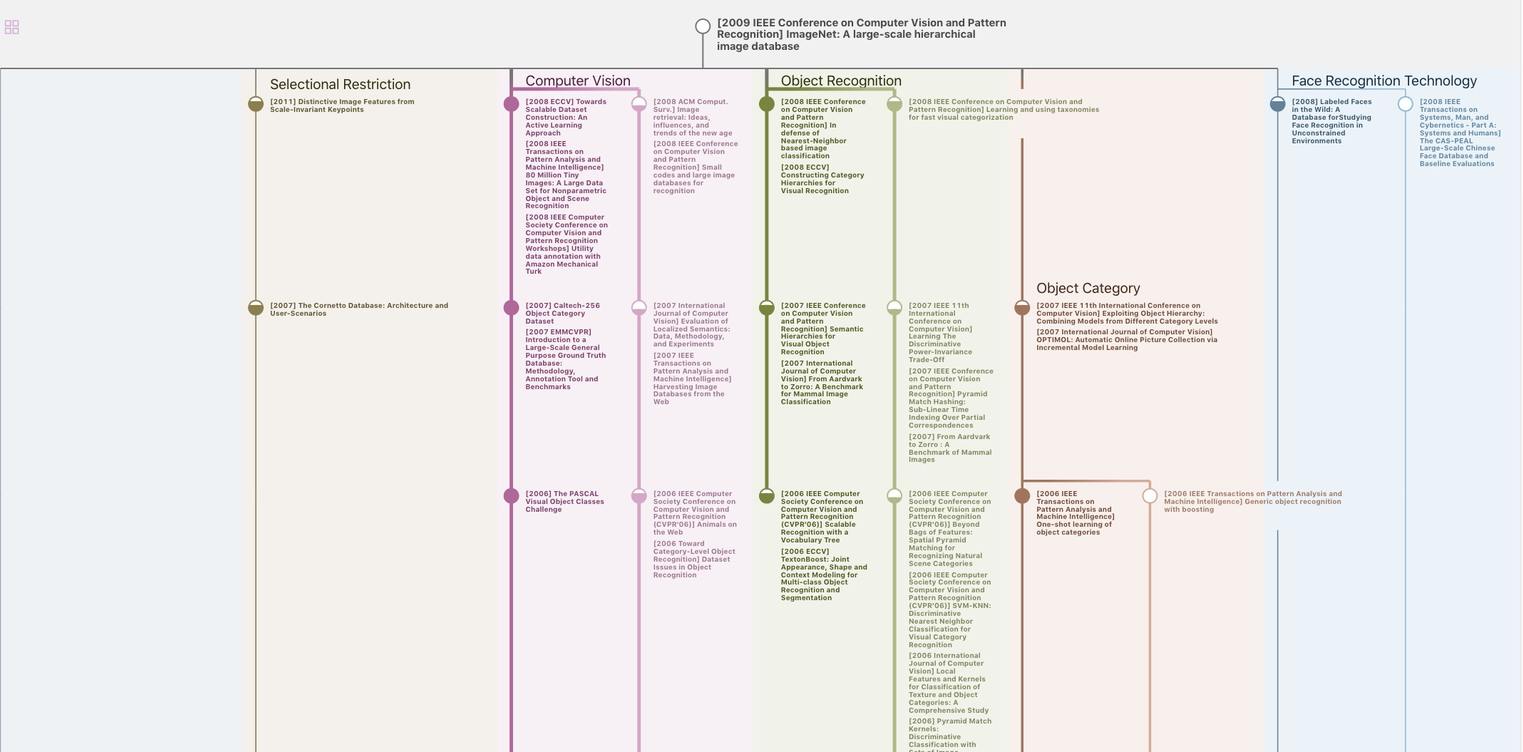
生成溯源树,研究论文发展脉络
Chat Paper
正在生成论文摘要