Prediction of early blight severity in tomato (Solanum lycopersicum) by machine learning technique
INDIAN JOURNAL OF AGRICULTURAL SCIENCES(2019)
摘要
Study of scenario and weather based prediction of severity of early blight (Alternaria solani Ell. & Mart) on tomato (Solanum lycopersicum L.) for five Indian states, viz. Rajendranagar (Telangana), Bengaluru (Karnataka), Rahuri (Maharashtra), Raipur (Chhattisgarh) and Ludhiana (Punjab) was made using advanced statistical method of support vector regression (SVR) with its accuracy compared with conventional multiple linear regression (MLR) model. Comparisons of early blight severity for mean and maximum severity levels across seasons for each location was carried out using Duncan's Multiple Range Test (DMRT). Early blight mean and maximum severity levels were in order. Bengaluru (KA) > Rajendranagar (Ts) > Rahuri (MH) > Raipur (CG) > Ludhiana (PB). Ludhiana (PB) had nil incidence during 2015 and not greater than 5% of either mean or maximum severity in any season. Both minimum temperature and morning relative humidity of one and two lagged weeks had negative and positive influence respectively, on mean and maximum severity of early blight at Rajendranagar (TS), Bengaluru (KA) and Rahuri (MH), which had higher blight severity over Raipur (CG) and Ludhiana (PB). MLR indicated 22-56% and 21-61% of variability with respect to mean and maximum severity of early blight due to weather factors that varied with locations. SVR predicted early blight severity nearer to actual values over MLR in terms of goodness of fit as well as Root Mean Square Error (RMSE).
更多查看译文
关键词
Early blight,MLR,SVR,Tomato,Weather
AI 理解论文
溯源树
样例
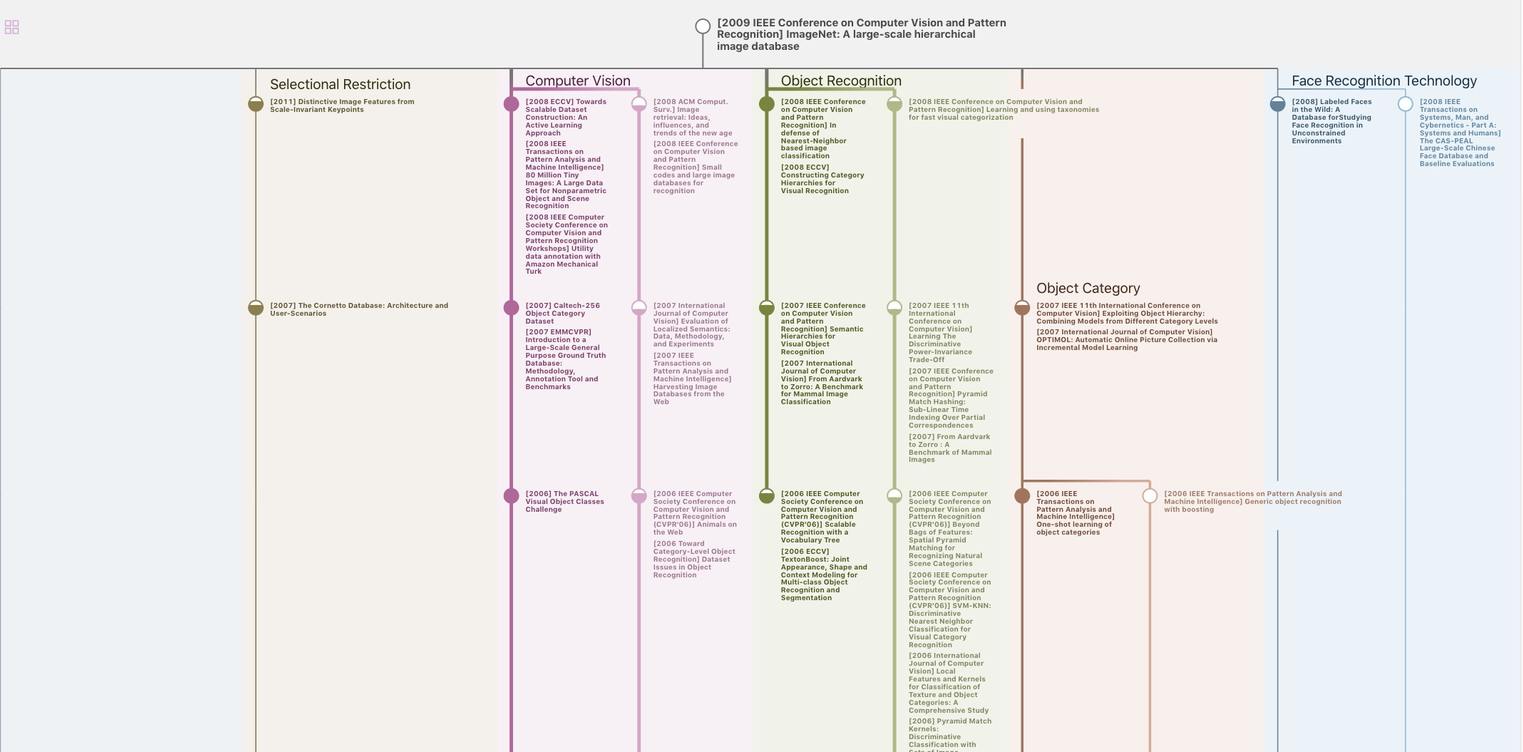
生成溯源树,研究论文发展脉络
Chat Paper
正在生成论文摘要