Attention-Gating For Improved Radio Galaxy Classification
MONTHLY NOTICES OF THE ROYAL ASTRONOMICAL SOCIETY(2021)
摘要
In this work, we introduce attention as a state-of-the-art mechanism for classification of radio galaxies, using convolutional neural networks. We present an attention-based model that performs on par with previous classifiers while using more than 50 per cent fewer parameters than the next smallest classic CNN application in this field. We demonstrate quantitatively how the selection of normalization and aggregation methods used in attention-gating can affect the output of individual models, and show that the resulting attention maps can be used to interpret the classification choices made by the model. We observe that the salient regions identified by the our model align well with the regions an expert human classifier would attend to make equivalent classifications. We show that while the selection of normalization and aggregation may only minimally affect the performance of individual models, it can significantly affect the interpretability of the respective attention maps and by selecting a model which aligns well with how astronomers classify radio sources by eye, a user can employ the model in a more effective manner.
更多查看译文
关键词
methods: statistical, techniques: image processing, radio continuum: galaxies
AI 理解论文
溯源树
样例
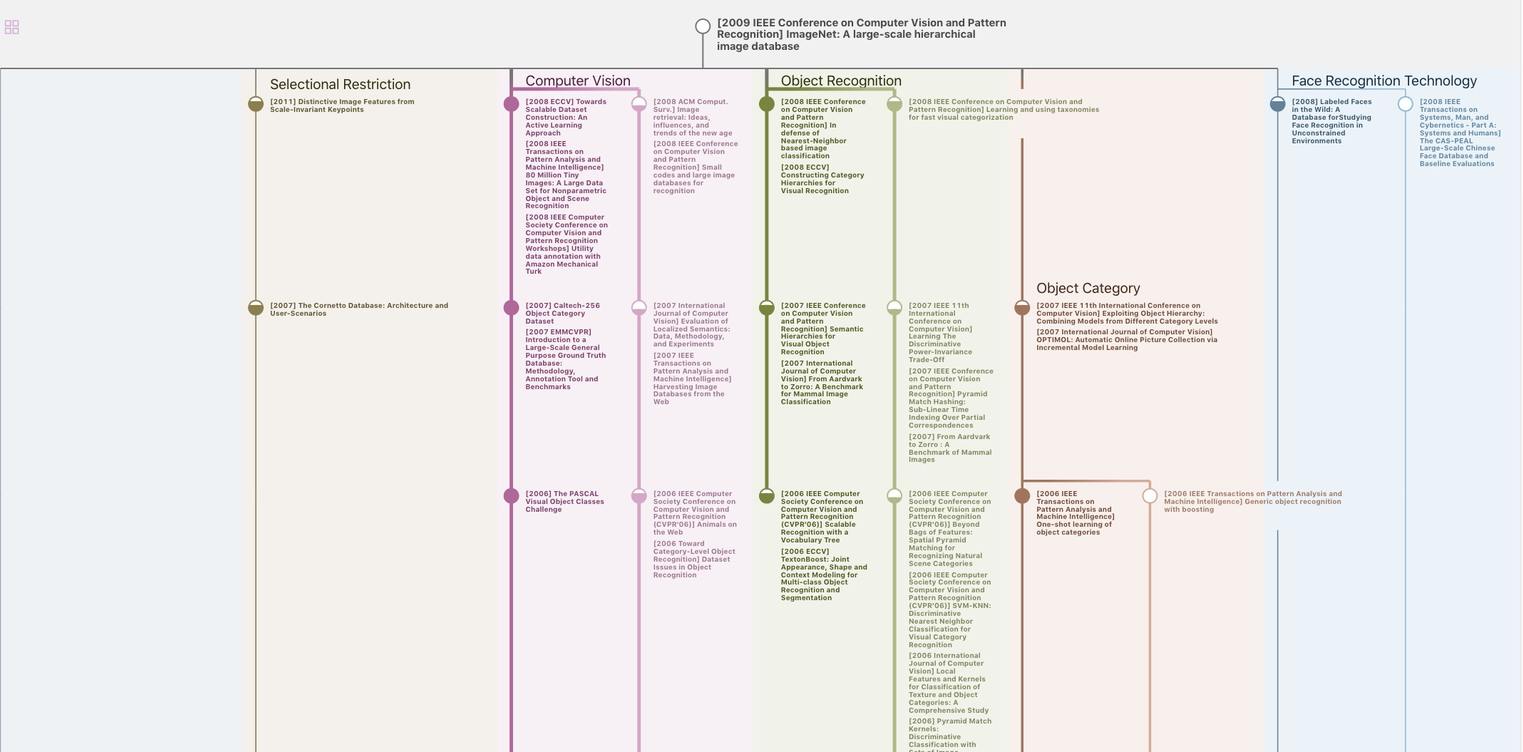
生成溯源树,研究论文发展脉络
Chat Paper
正在生成论文摘要