Vibration-Based Support Vector Machine for Structural Health Monitoring
Lecture Notes in Civil Engineering(2018)
摘要
There are ever-increasing interests to determine effectively structural diagnosis and conditional assessment for structural health monitoring (SHM). Although research has been extensively conducted in the conventional physical-based vibration analysis, advancement in sensor technologies and complexity in structural systems post great challenges in the effectiveness of these techniques. Alternatively, various data-driven based machine learning techniques are recently emerging tools to data clarification. In this study, a new vibration-based machine learning was proposed for condition assessment and damage detection in SHM. Three vibration-based feature extraction methods, autoregressive, vector autoregressive and singular value decomposition methods, were used as damage sensitive features. A kernel function based support vector machine was used to facilitate the identification between damaged and undamaged cases. A benchmark with varying environment and operational conditions in the literature was selected to verify the effectiveness of the proposed methods. The results showed that three feature methods could effectively map damage features in a high dimensional feature space, thereby dramatically improving the effectiveness and accuracy of data classification. Moreover, comparisons of results revealed that the singular value decomposition methods exhibit higher sensitivity to damage states as compared to other two approaches.
更多查看译文
关键词
Vibration,Machine learning,Damage feature extraction,Structural health monitoring,Damage detection
AI 理解论文
溯源树
样例
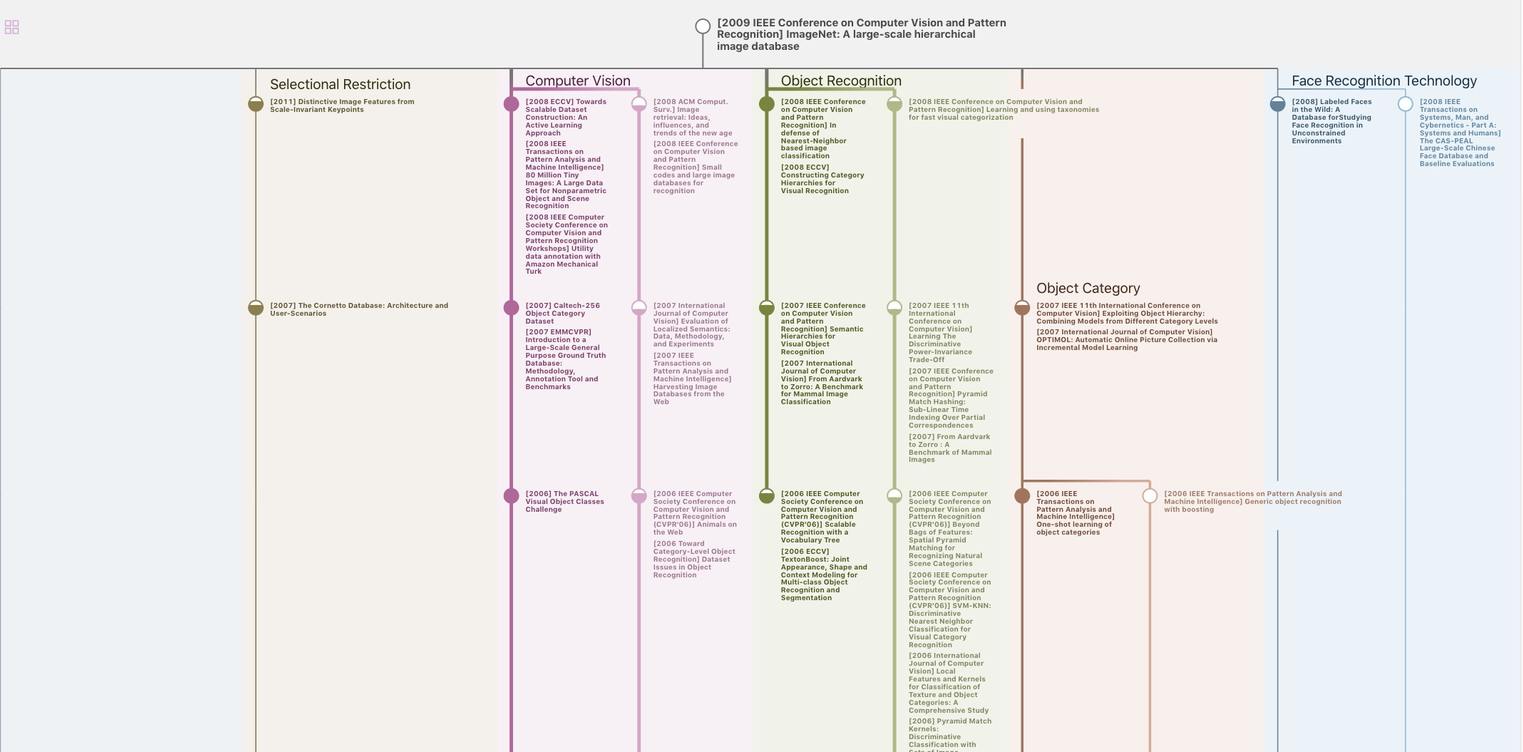
生成溯源树,研究论文发展脉络
Chat Paper
正在生成论文摘要