Forecasting the Number of Out-Of-Hospital Cardiac Arrest in the Young Using Generalized Additive Model
CIRCULATION(2019)
摘要
Introduction: Accurate forecasting could help in resource planning and evaluation of intervention efforts to reduce out-of-hospital cardiac arrest. Hypothesis: Generalized additive model can rapidly and accurately forecast the number of OHCA in the young (1-35 years old) when compared to other auto regressive moving average (ARIMA) based models. Methods: Data were obtained from CARES in Chicago from May 2013 to December 2017. Monthly forecasts of the number of OHCA were performed using a generative additive model framework using the open source software Prophet and R. The first 50 months served as training and the last 6 months served as testing. Results: Figure 1 shows the distribution of the number of cases over the 3-year study period showing yearly seasonality and upward trend. Figure 2 shows the forecast of the number of arrest (middle line) along with the actual number of arrest (dots). The shaded band represents the 95% confidence interval of the prediction. Figure 3 show the comparison of the 6-month forecast and actual data. This model has low root mean square error score when compared with other ARIMA based models (2 vs. 4). Conclusion: Accurate time series forecasting of the number of OHCA arrests could be achieved. Time series analysis and forecasting are essential tools in evaluating the effectiveness of intervention efforts to reduce OHCA as they allow for causality hypothesis testing.
更多查看译文
AI 理解论文
溯源树
样例
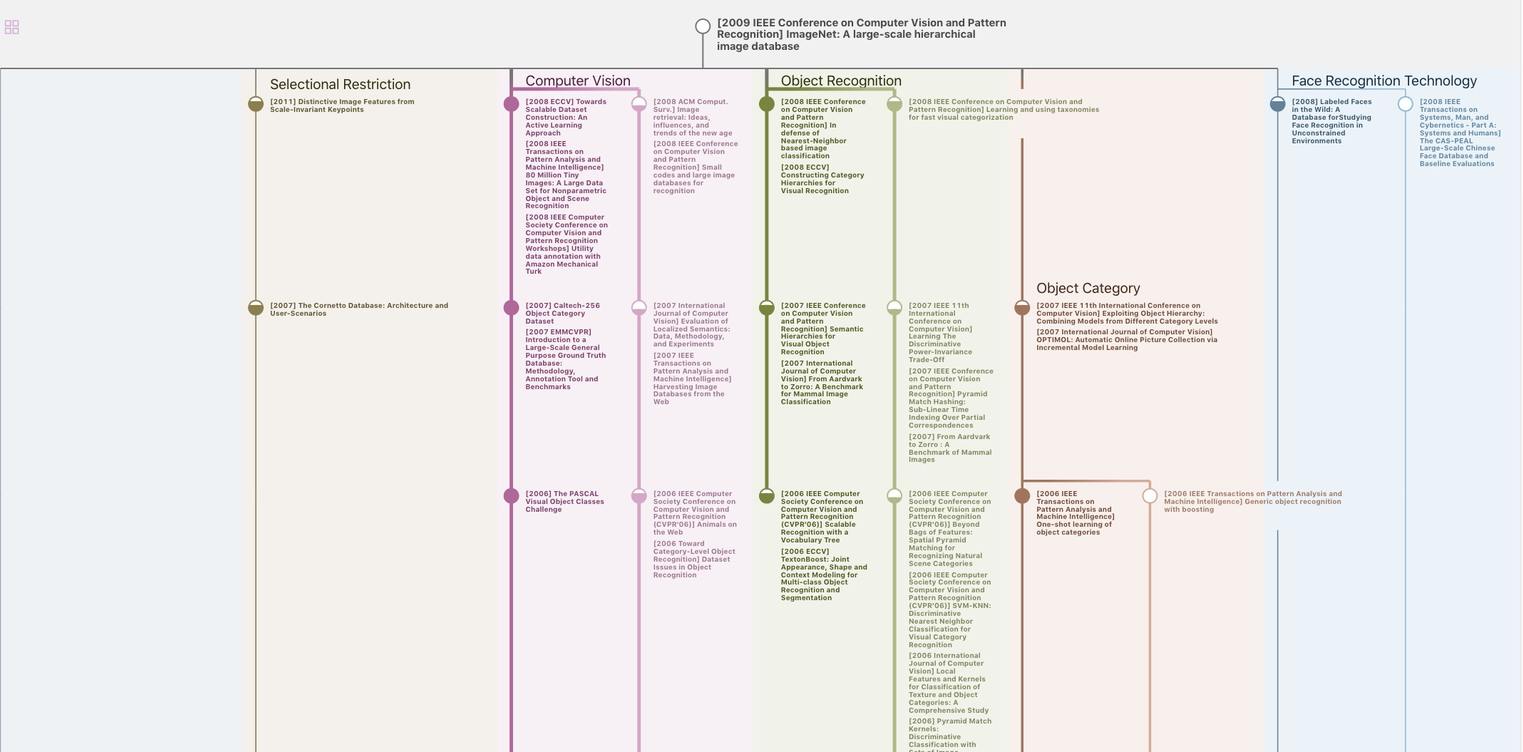
生成溯源树,研究论文发展脉络
Chat Paper
正在生成论文摘要