Theme-Based Partitioning Approach to Decision Tree: An Extended Experimental Analysis
Lecture Notes in Electrical Engineering(2019)
摘要
Decision tree is a well-established technique for classification in data mining and machine learning. Induction of a decision tree using high-dimensional dataset may lower the performance of the decision tree in terms of classification rate and stability. Vertical partitioning is a novel paradigm to avoid these issues; it divides the features of a dataset into subsets and creates a subset-based classifier ensemble. In our previous work, we proposed a theme-based decision tree classifier ensemble using vertical partitioning for teacher recruitment modelling. In this paper, we extend our previous work in terms of exhaustive experimental analysis to address both high-dimensionality and instability issues of decision tree using five standard datasets. The performance of the theme-based method is evaluated using classification rate, standard deviation and misclassification rate. Our experimental analysis confirms the superiority of the theme-based approach over traditional decision tree approaches.
更多查看译文
关键词
Decision tree,Vertical partitioning,High dimensionality and instability
AI 理解论文
溯源树
样例
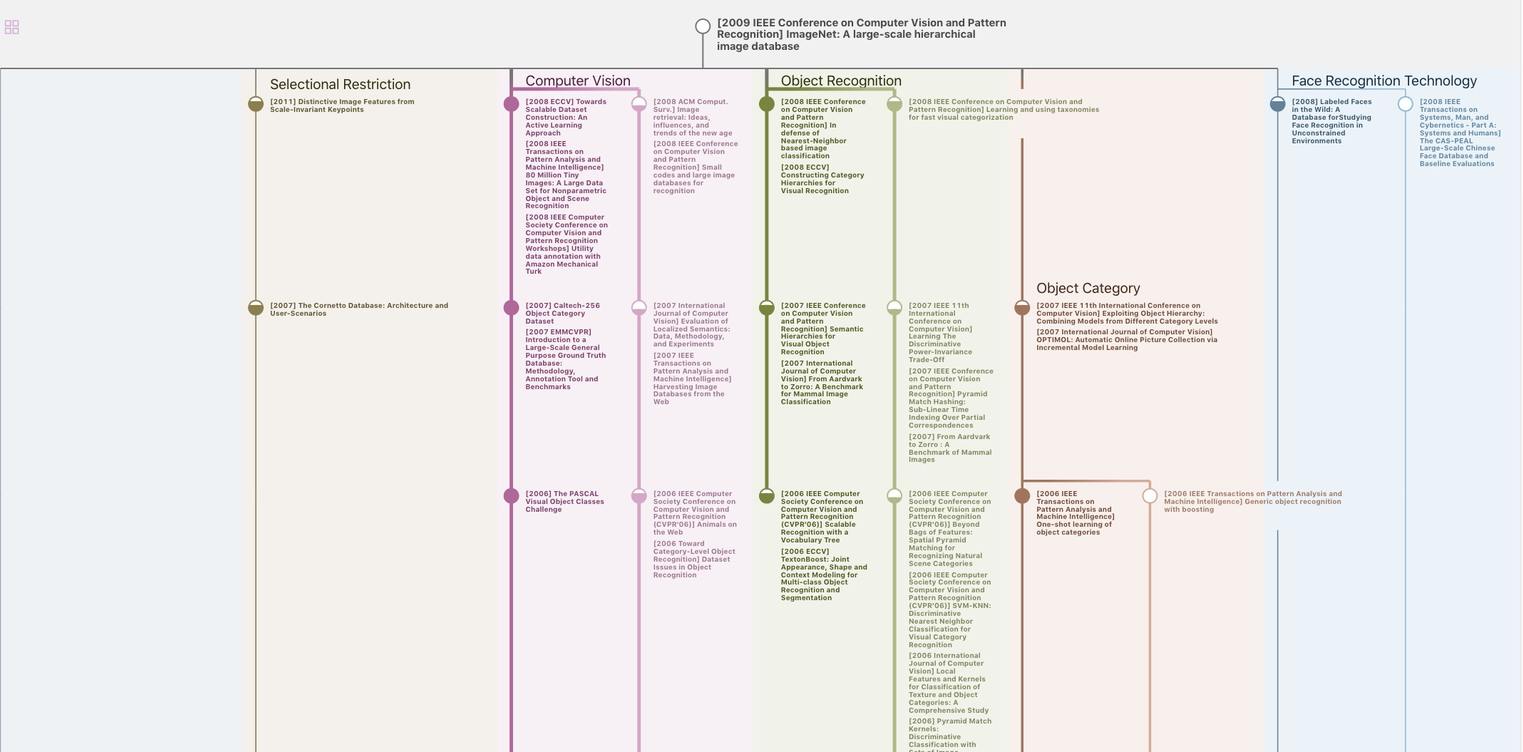
生成溯源树,研究论文发展脉络
Chat Paper
正在生成论文摘要