Reducing Textural Bias Improves Robustness of Deep Segmentation CNNs
arxiv(2020)
摘要
Despite current advances in deep learning, domain shift remains a common problem in medical imaging settings. Recent findings on natural images suggest that deep neural models can show a textural bias when carrying out image classification tasks, which goes against the common understanding of convolutional neural networks (CNNs) recognising objects through increasingly complex representations of shape. This study draws inspiration from recent findings on natural images and aims to investigate ways in which addressing the textural bias phenomenon could be used to bring up the robustness and transferability of deep segmentation models when applied to three-dimensional (3D) medical data. To achieve this, publicly available MRI scans from the Developing Human Connectome Project are used to investigate ways in which simulating textural noise can help train robust models in a complex segmentation task. Our findings illustrate how applying specific types of textural filters prior to training the models can increase their ability to segment scans corrupted by previously unseen noise.
更多查看译文
关键词
Textural bias, Domain shift, Robustness, Segmentation
AI 理解论文
溯源树
样例
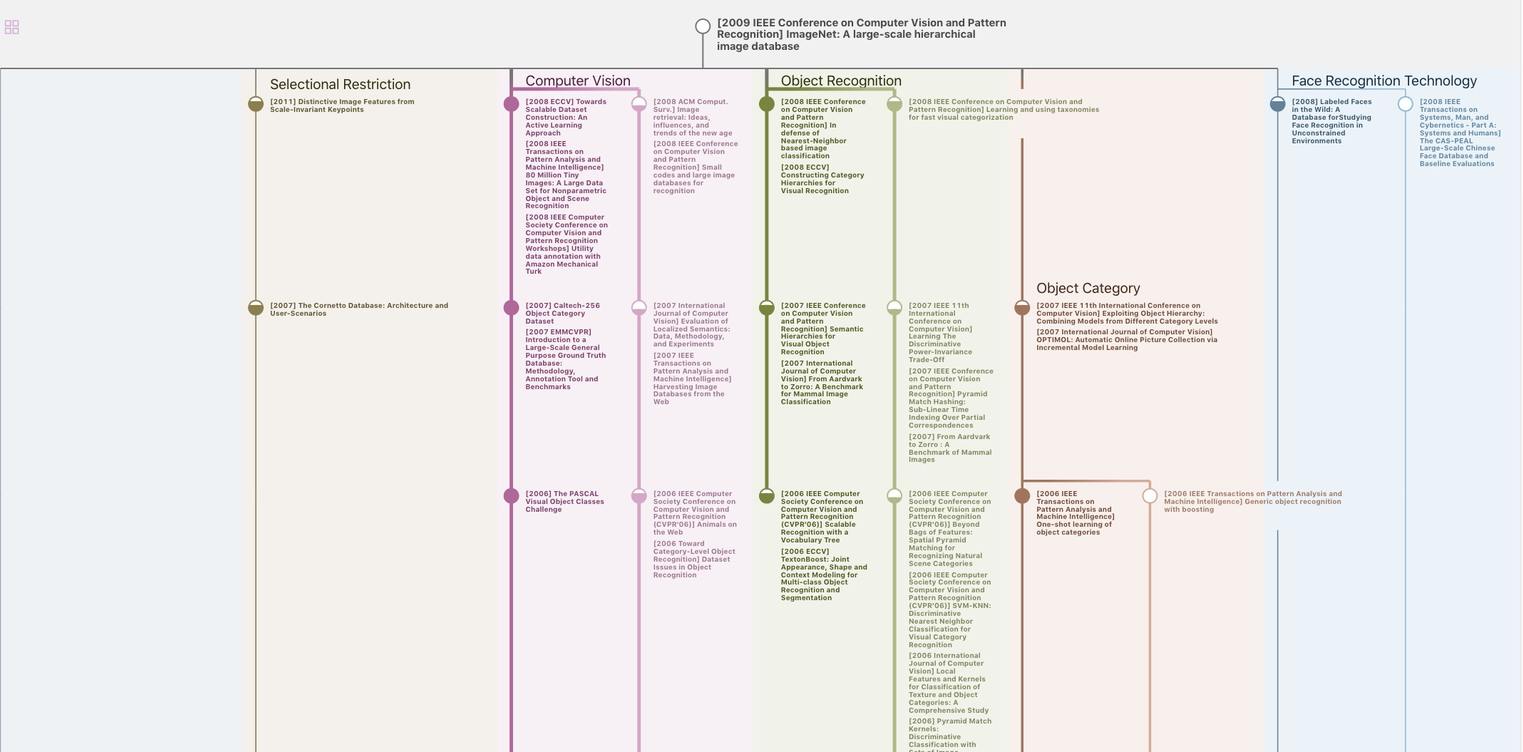
生成溯源树,研究论文发展脉络
Chat Paper
正在生成论文摘要