High-dimensional Canonical Forest
JOURNAL OF STATISTICAL COMPUTATION AND SIMULATION(2017)
摘要
Recently, a new ensemble classification method named Canonical Forest (CF) has been proposed by Chen et al. [Canonical forest. Comput Stat. 2014; 29: 849-867]. CF has been proven to give consistently good results in many data sets and comparable to other widely used classification ensemble methods. However, CF requires an adopting feature reduction method before classifying high-dimensional data. Here, we extend CF to a high-dimensional classifier by incorporating a random feature subspace algorithm [Ho TK. The random subspace method for constructing decision forests. IEEE Trans Pattern Anal Mach Intell. 1998; 20: 832-844]. This extended algorithm is called HDCF (high-dimensional CF) as it is specifically designed for high-dimensional data. We conducted an experiment using three data sets - gene imprinting, oestrogen, and leukaemia - to compare the performance of HDCF with several popular and successful classification methods on high-dimensional data sets, including Random Forest [Breiman L. Random forest. Mach Learn. 2001; 45: 5-32], CERP [Ahn H, et al. Classification by ensembles from random partitions of high-dimensional data. Comput Stat Data Anal. 2007; 51: 6166-6179], and support vector machines [Vapnik V. The nature of statistical learning theory. New York: Springer; 1995]. Besides the classification accuracy, we also investigated the balance between sensitivity and specificity for all these four classification methods.
更多查看译文
关键词
Canonical Forest,canonical linear discriminant analysis,classification,ensemble,high-dimensional data,Random Subspace
AI 理解论文
溯源树
样例
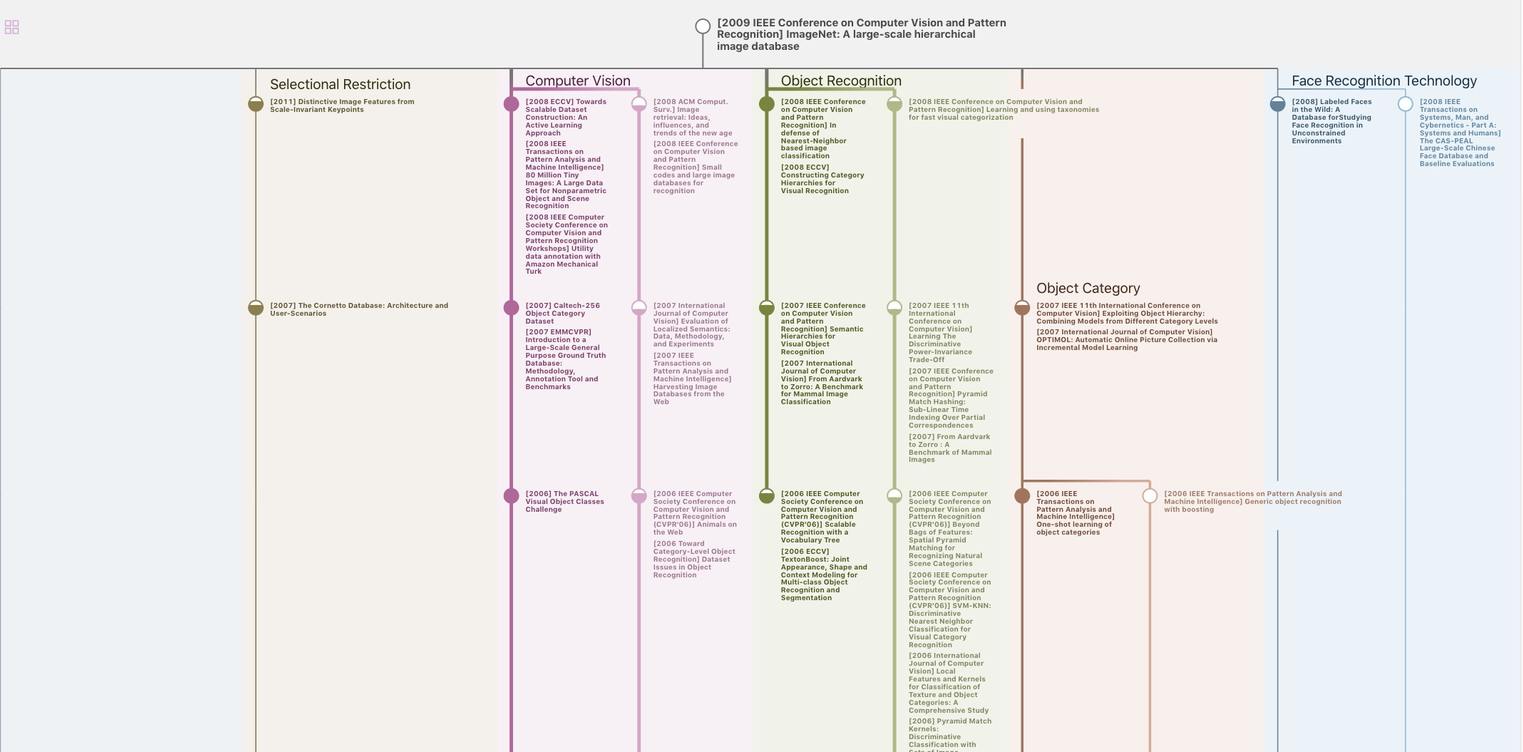
生成溯源树,研究论文发展脉络
Chat Paper
正在生成论文摘要