ML Health Monitor: Taking the Pulse of Machine Learning Algorithms in Production
Proceedings of SPIE(2019)
摘要
Bringing the research advances in Machine Learning (ML) to production is necessary for businesses to gain value from ML. A key challenge of production ML is the monitoring and management of real-time prediction quality. This is complicated by the variability of live production data, the absence of real-time labels and the non-determinism posed by ML techniques themselves. We define ML Health as the real time assessment of ML prediction quality and present an approach to monitoring and improving ML Health. Specifically, a complete solution to monitor and manage ML Health within a realistic full production ML lifecycle. We describe a number of ML Health techniques and assess their efficacy via publicly available datasets. Our solution handles production realities such as scale, heterogeneity and distributed runtimes. We present what we believe is the first solution to production ML Health explored at both an empirical and complete system implementation level.
更多查看译文
关键词
machine learning operations,prediction confidence,model performance,data deviation,ML Health,diagnostics,data drift,model drift,real time,ML lifecycle management,production ML
AI 理解论文
溯源树
样例
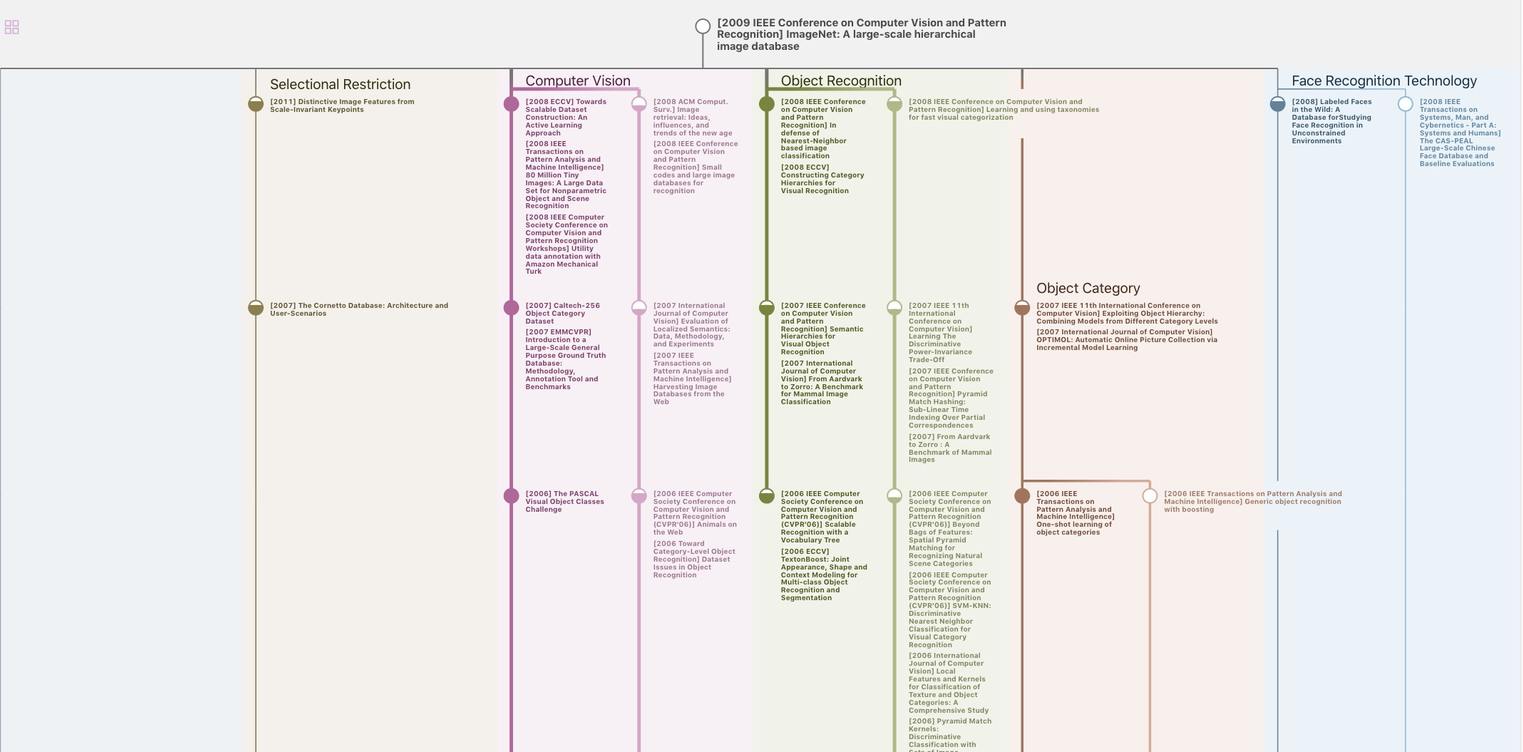
生成溯源树,研究论文发展脉络
Chat Paper
正在生成论文摘要