Deep Learning and Video Quality Analysis
Proceedings of SPIE(2019)
摘要
For more than 30 years, the video coding industry has been using mean-squared error-based PSNR as a measure of video quality, despite evidence of its inadequacy. Moreover, in the encoder, SAD is used instead of MSE to save multiplications. We quantify how these measures are inadequately correlated to subjective scores and obtain new measures that correlate much better. We focus on the problem of full-reference assessment of video degraded only by coding and scaling errors, such as experienced by streaming services, and put aside issues of transmission, such as timing jitters, rebufferings, etc. We begin with the Video Multi-Assessment Fusion (VMAF) algorithm introduced by Netflix. Results with up to 97% correlation accuracy to subjective scores are reported on two Netflix datasets, using a neural network model.
更多查看译文
关键词
video quality analysis,video coding,VMAF,deep learning
AI 理解论文
溯源树
样例
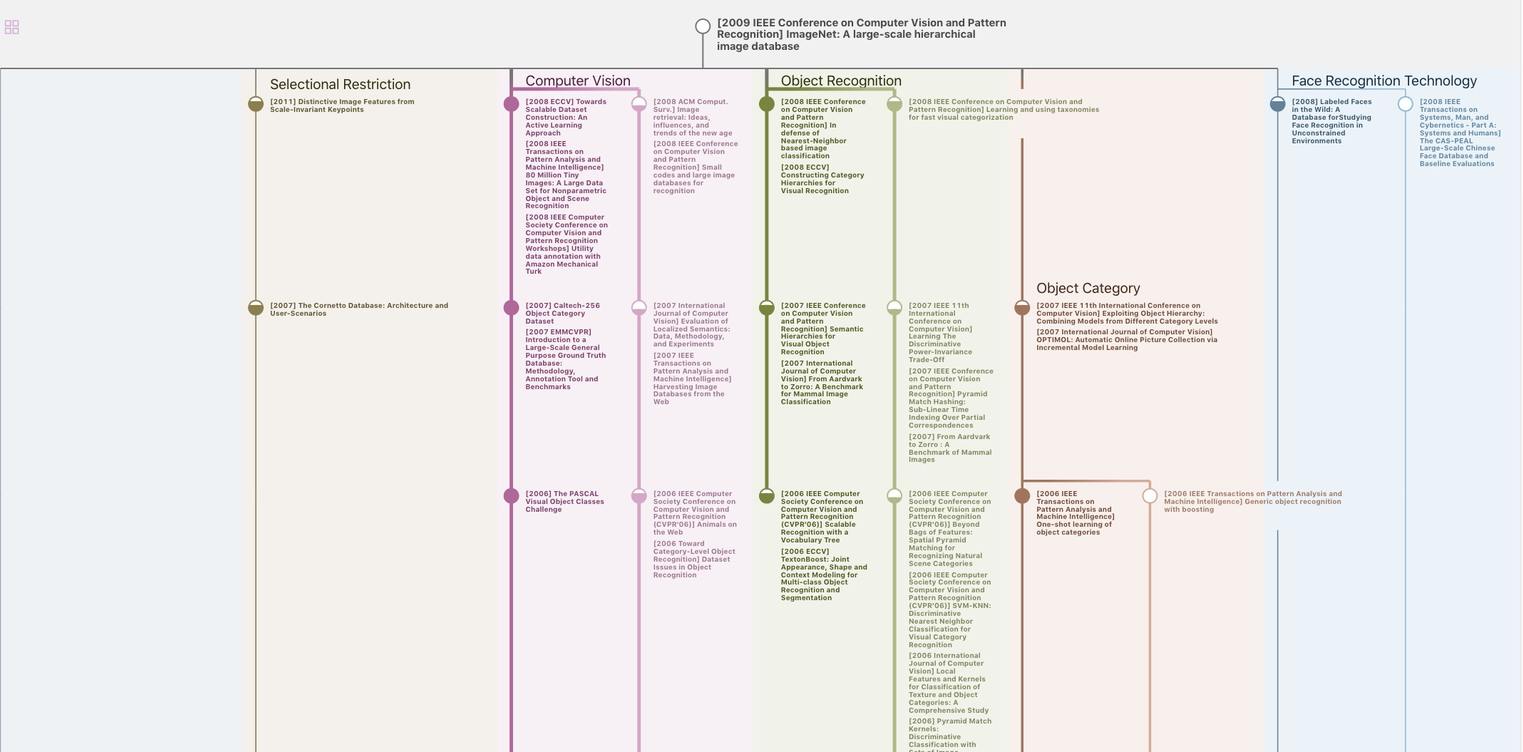
生成溯源树,研究论文发展脉络
Chat Paper
正在生成论文摘要