BotMoE: Twitter Bot Detection with Community-Aware Mixtures of Modal-Specific Experts
PROCEEDINGS OF THE 46TH INTERNATIONAL ACM SIGIR CONFERENCE ON RESEARCH AND DEVELOPMENT IN INFORMATION RETRIEVAL, SIGIR 2023(2023)
摘要
Twitter bot detection has become a crucial task in efforts to combat online misinformation, mitigate election interference, and curb malicious propaganda. However, advanced Twitter bots often attempt to mimic the characteristics of genuine users through feature manipulation and disguise themselves to fit in diverse user communities, posing challenges for existing Twitter bot detection models. To this end, we propose BotMoE(1), a Twitter bot detection framework that jointly utilizes multiple user information modalities (metadata, textual content, network structure) to improve the detection of deceptive bots. Furthermore, BotMoE incorporates a community-aware Mixture-of-Experts (MoE) layer to improve domain generalization and adapt to different Twitter communities. Specifically, BotMoE constructs modal-specific encoders for metadata features, textual content, and graph structure, which jointly model Twitter users from three modal-specific perspectives. We then employ a community-aware MoE layer to automatically assign users to different communities and leverage the corresponding expert networks. Finally, user representations from metadata, text, and graph perspectives are fused with an expert fusion layer, combining all three modalities while measuring the consistency of user information. Extensive experiments demonstrate that BotMoE significantly advances the state-of-the-art on three Twitter bot detection benchmarks. Studies also confirm that BotMoE captures advanced and evasive bots, alleviates the reliance on training data, and better generalizes to new and previously unseen user communities.
更多查看译文
关键词
Twitter Bot Detection,Mixture-of-Experts,Social Network Analysis
AI 理解论文
溯源树
样例
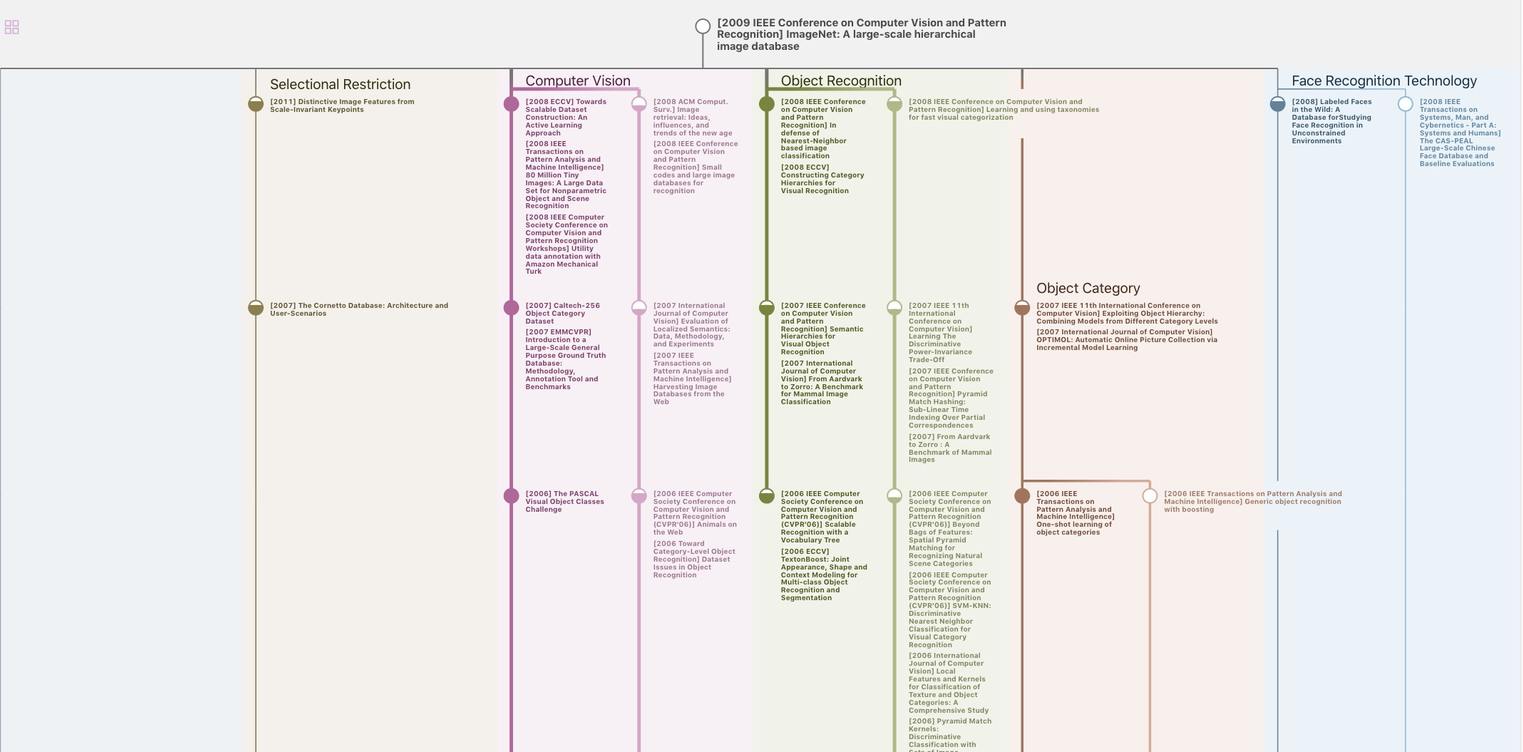
生成溯源树,研究论文发展脉络
Chat Paper
正在生成论文摘要