Improved Gaussian Inverse Wishart Probability Hypothesis Density for Extended Target Tracking
JOURNAL OF ELECTRONICS & INFORMATION TECHNOLOGY(2018)
摘要
Assumed that extension and measurement number of Extended Targets (ET) are respectively modeled as ellipse and Poisson, a Gaussian Inverse Wishart Probability Hypothesis Density (GIW-PHD) filter can estimate kinematic and extension states. However, for the number of spatially close targets and the extensions of non-ellipsoidal and occluded targets, the results estimated by this filter are not accurate enough. In view of these problems, an improved GIW-PHD filter is proposed in this paper. Firstly, assumed that target extension is modeled as a reference ellipse of the same size, a modified Random Matrix (RM) method is obtained by devising a new scatter matrix. Then, combining the improved RM method with the ET-PHD based on a measurement number multi-Bernoulli model, the improved GIW-PHD filter is obtained. Simulated and experimental results show that, compared with the traditional GIW-PHD, the improved GIW-PHD filter can obtain more accurate estimates in target number and the extensions of ellipsoidal and non-ellipsoidal targets with large measurement number and extensions.
更多查看译文
关键词
Extended target tracking,Gaussian Inverse Wishart Probability Hypothesis Density (GIW-PHD),Random Matrix (RM),Multi-Bernoulli distribution
AI 理解论文
溯源树
样例
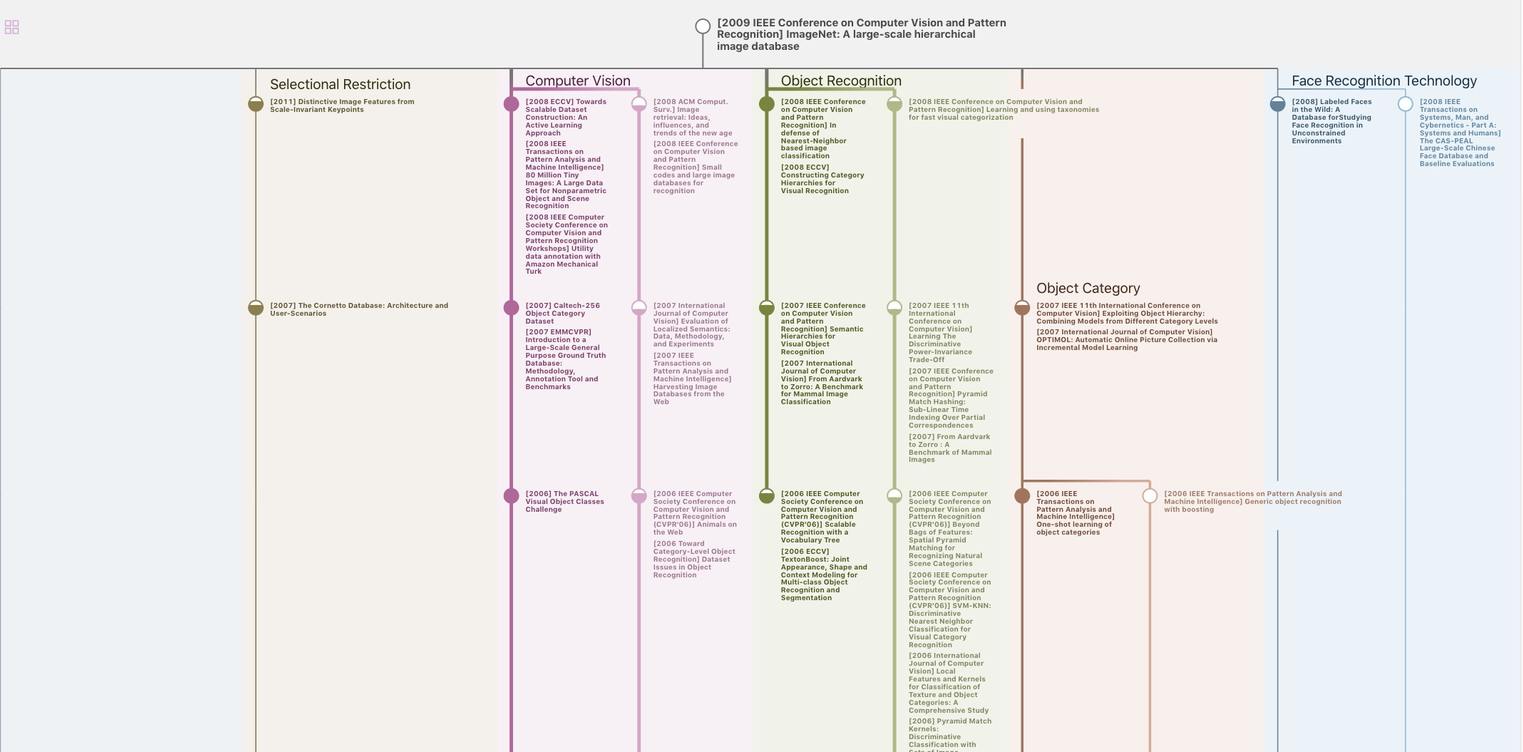
生成溯源树,研究论文发展脉络
Chat Paper
正在生成论文摘要