Photovoltaic power station identification using refined encoder-decoder network with channel attention and chained residual dilated convolutions
JOURNAL OF APPLIED REMOTE SENSING(2020)
摘要
The quantity and distribution of photovoltaic power stations play an important role in policymaking related to clean energy. High-resolution satellite imagery with a wide field of view enables photovoltaic power station monitoring at low cost. The separability varies greatly due to distinct backgrounds in different regions, which makes photovoltaic power station identification a challenging task. We propose an end-to-end semantic segmentation network to identify photovoltaic power stations under multiple complex backgrounds in Gaofen 1 imageries. Our network adopts the encoder-decoder architecture in which three modules between the encoder and decoder are added, i.e., feature refinement residual module (FRRM), chained dilation attention module (CDAM), and global channel attention module (GCAM). FRRM uses the structure of residual connection to refine the features from each stage of the encoder. CDAM consisting of chained residual dilated convolutions with different dilation rates and channel attention can enlarge the receptive field without reducing image resolution and select useful features. GCAM utilizes high-level features containing rich semantic information as a guide to select more discriminative low-level features. Experiments demonstrate the effectiveness of each module and the ability of the proposed network to improve photovoltaic power station identification under complex backgrounds. (C) 2020 Society of Photo-Optical Instrumentation Engineers (SPIE)
更多查看译文
关键词
photovoltaic power station,identification,remote sensing,semantic segmentation,encoder-decoder,channel attention
AI 理解论文
溯源树
样例
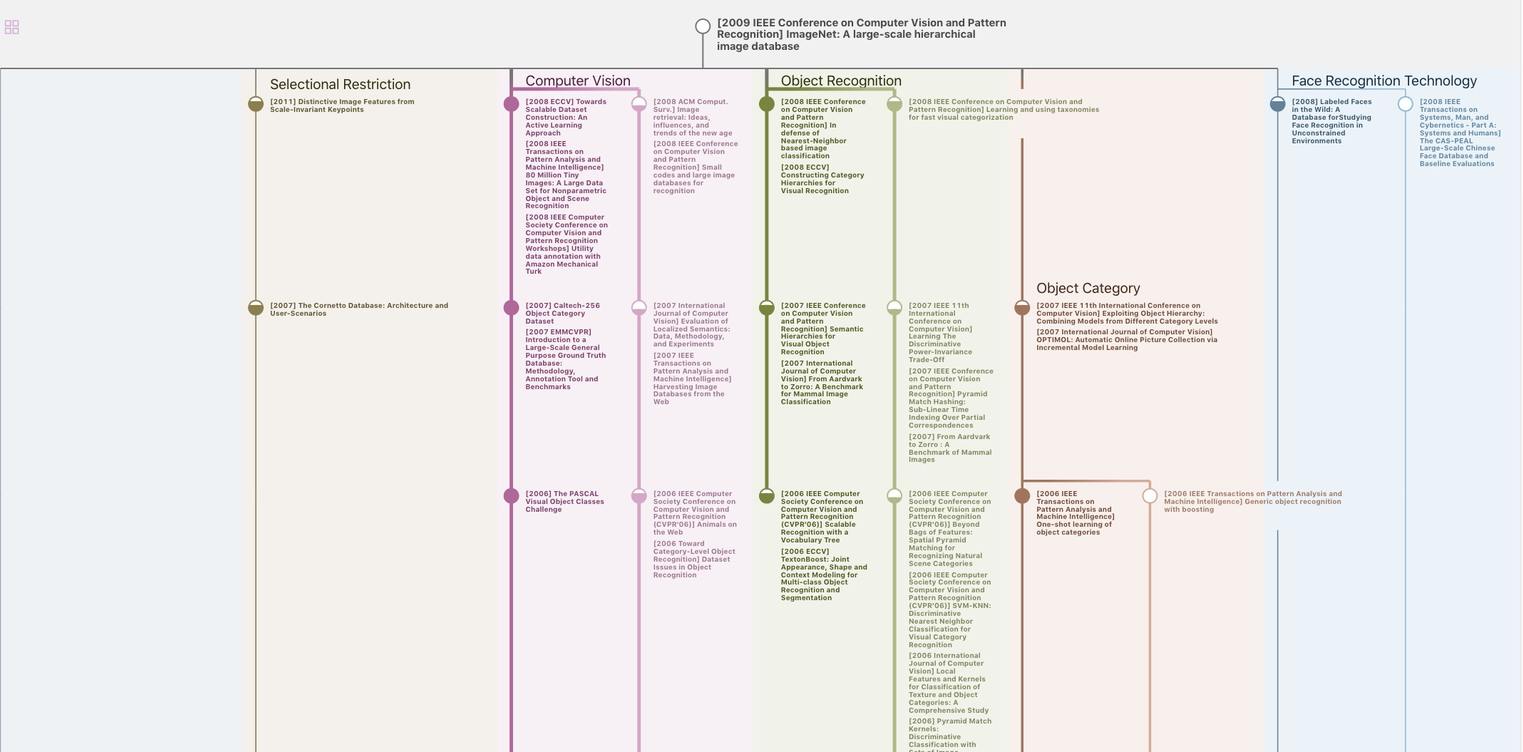
生成溯源树,研究论文发展脉络
Chat Paper
正在生成论文摘要