AIR QUALITY PROGNOSIS USING ARTIFICIAL NEURAL NETWORKS MODELING IN THE URBAN ENVIRONMENT OF VOLOS, CENTRAL GREECE
FRESENIUS ENVIRONMENTAL BULLETIN(2014)
摘要
It is well known that natural and anthropogenic emissions of ambient pollutants affect air quality and as a consequence the public health. Various epidemiological studies have identified particulate matter (PM10) and surface ozone (O-3) as key air pollutants triggering adverse health effects on humans. The objective of this study is the prognosis, one day ahead, of air quality in the Volos urban area, a medium sized city at the eastern seaboard of Central Greece, using Artificial Neural Networks (ANNs). For that purpose, two ANN forecasting models were used. The first ANN model was appropriately developed to forecast the next day mean daily PM10 concentration, while the second one to forecast the next day maximum daily surface ozone's 8-hour moving average concentration. Both ANN models were trained using values for the mean daily relative humidity (%), the mean daily air temperature (degrees C), the mean daily wind speed (m/s), the mean daily PM10 concentration (mu g/m(3)) and the maximum daily surface ozone's 8-hour moving average concentration (mu g/m(3)). Meteorological and air quality data were acquired from the Volos air pollution-monitoring station with fully automated analyzers installed by the Hellenic Ministry of the Environment, Energy and Climate Change, covering the time period 2001-2009. Results indicate that ANN modeling is a promising tool at an operational planning level for State bodies in order to forecast air pollution and protect public health. The coefficient of determination was found to be 0.476 in the case of PM10 prognosis while the same coefficient was 0.856 for O-3 prognosis. Furthermore, the forecasting index of agreement was found to be 0.777 for PM10 and 0.958 for O-3, which indicates that the forecasting values for concentrations are very close to the observed concentrations. Overall, the statistical analysis showed that the predictive ability of the proposed ANN forecasting models is very good at a significant statistical level of p<0.01.
更多查看译文
关键词
Air quality,prognosis,ANN modeling,Volos,Greece
AI 理解论文
溯源树
样例
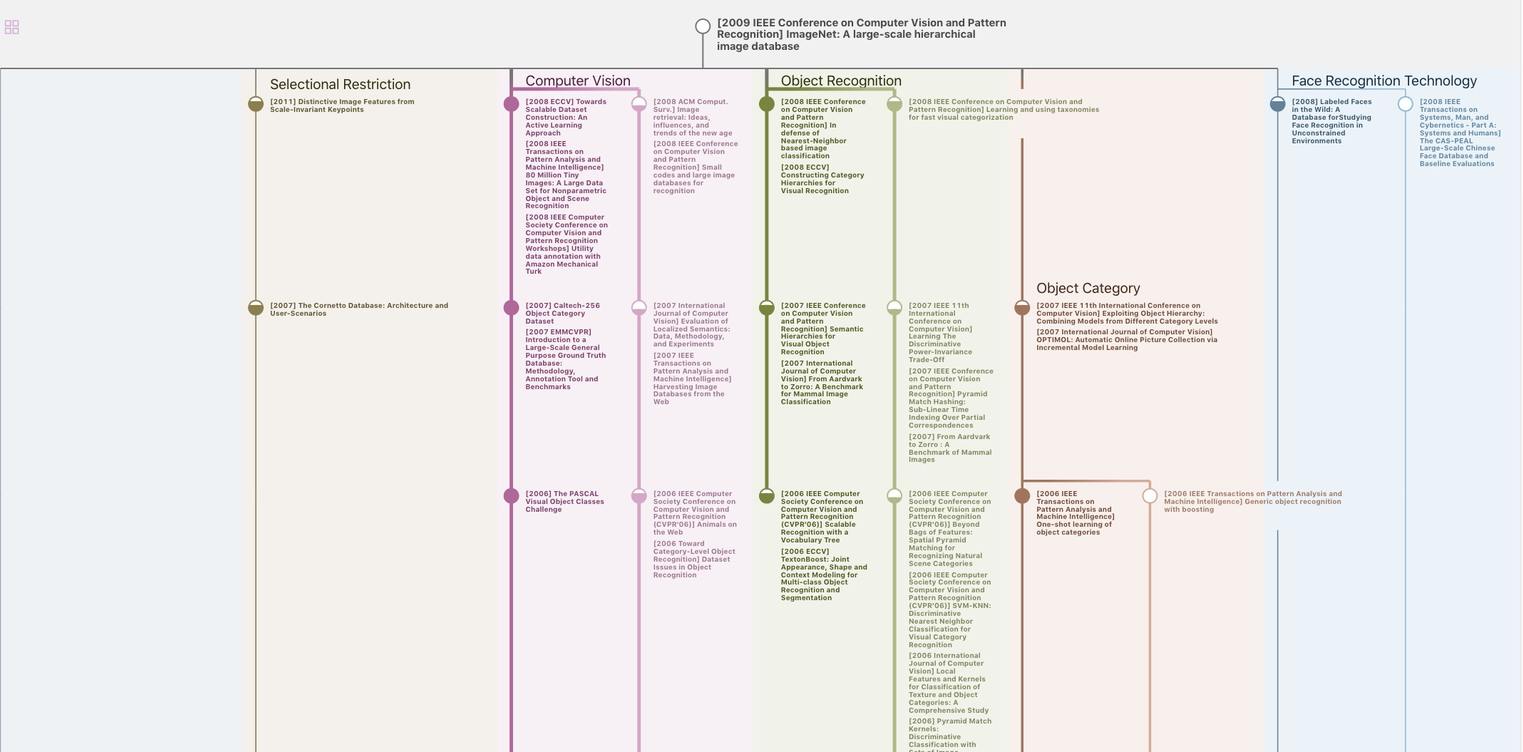
生成溯源树,研究论文发展脉络
Chat Paper
正在生成论文摘要