SE-Dual Path Networks combined with a Navigator for Fine-Grained Classification
Proceedings of SPIE(2019)
摘要
Recognizing fine-grained categories is difficult due to the challenges of discriminative region localization and fine-grained feature learning. To handle this circumstance, we propose a novel model termed SDN-Net for SE-DPN-Navigator Networks, which consists of DPN (Dual Path Networks), SE-blocks (Squeeze-and-Excitation Blocks) and a Navigator. DPN shares common features while maintaining the flexibility to explore new features. Moreover, we add SE-blocks into DPN to make up the SE-DPN which acts as a feature extractor of the proposed model, SE-blocks helps the model learn to use global information to selectively emphasize informative features and suppress less useful ones. We also use a Navigator to help the model to detect most informative regions without extra bounding box/part annotations. Our model can be trained end-to-end. With the great cooperation between these three components, we achieve state-of-the-art performance on two publicly available fine-grained recognition datasets (CUB-200-2001 and Stanford Cars). Besides, We have done ablation studies and confirmed the effectiveness of each components in the proposed model.
更多查看译文
关键词
Navigator,fine-grained,Squeeze-and-Excitation,Dual Path Networks
AI 理解论文
溯源树
样例
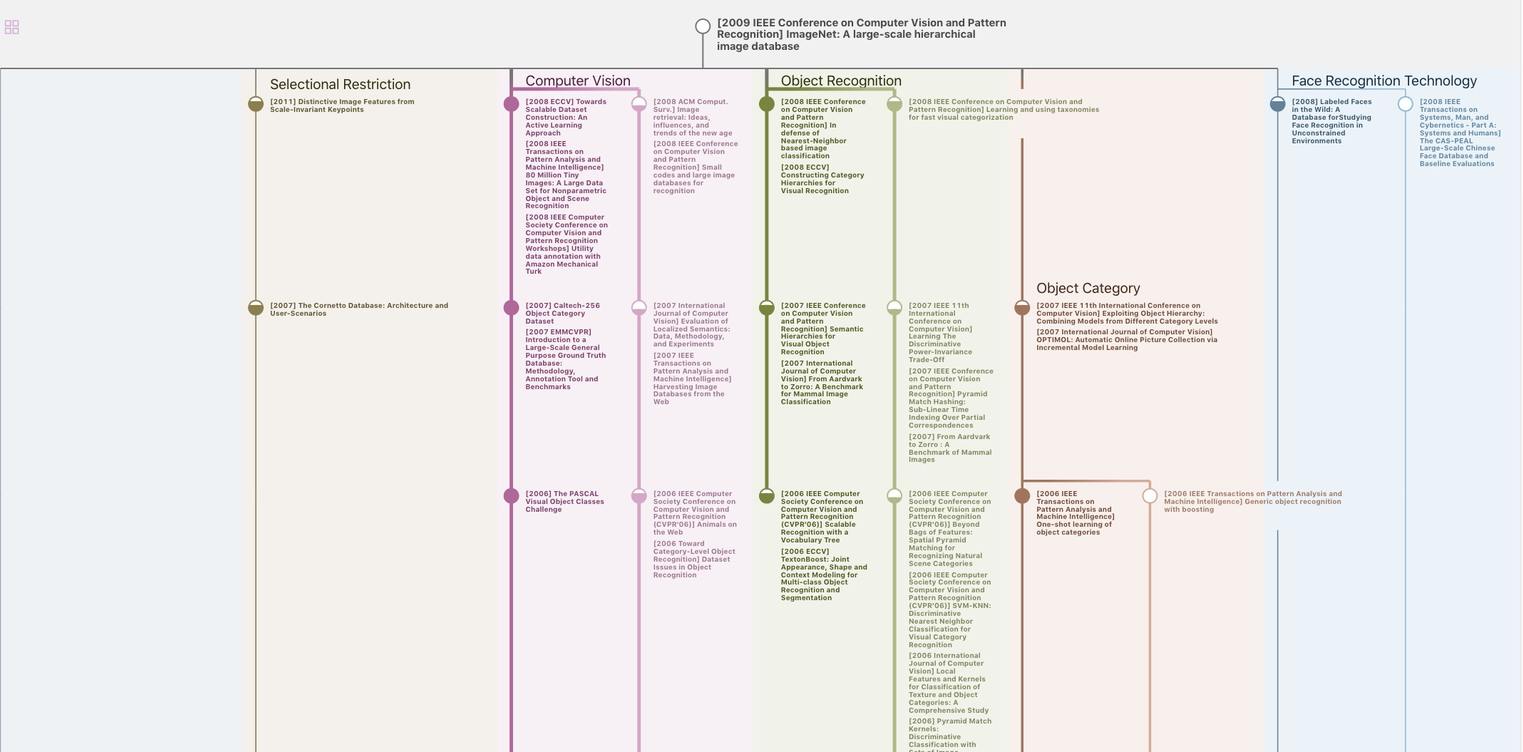
生成溯源树,研究论文发展脉络
Chat Paper
正在生成论文摘要