Robust And Private Learning Of Halfspaces
24TH INTERNATIONAL CONFERENCE ON ARTIFICIAL INTELLIGENCE AND STATISTICS (AISTATS)(2021)
摘要
In this work, we study the trade-off between differential privacy and adversarial robustness under L-2-perturbations in the context of learning halfspaces. We prove nearly tight bounds on the sample complexity of robust private learning of halfspaces for a large regime of parameters. A highlight of our results is that robust and private learning is harder than robust or private learning alone. We complement our theoretical analysis with experimental results on the MNIST and USPS datasets, for a learning algorithm that is both differentially private and adversarially robust.
更多查看译文
关键词
private learning
AI 理解论文
溯源树
样例
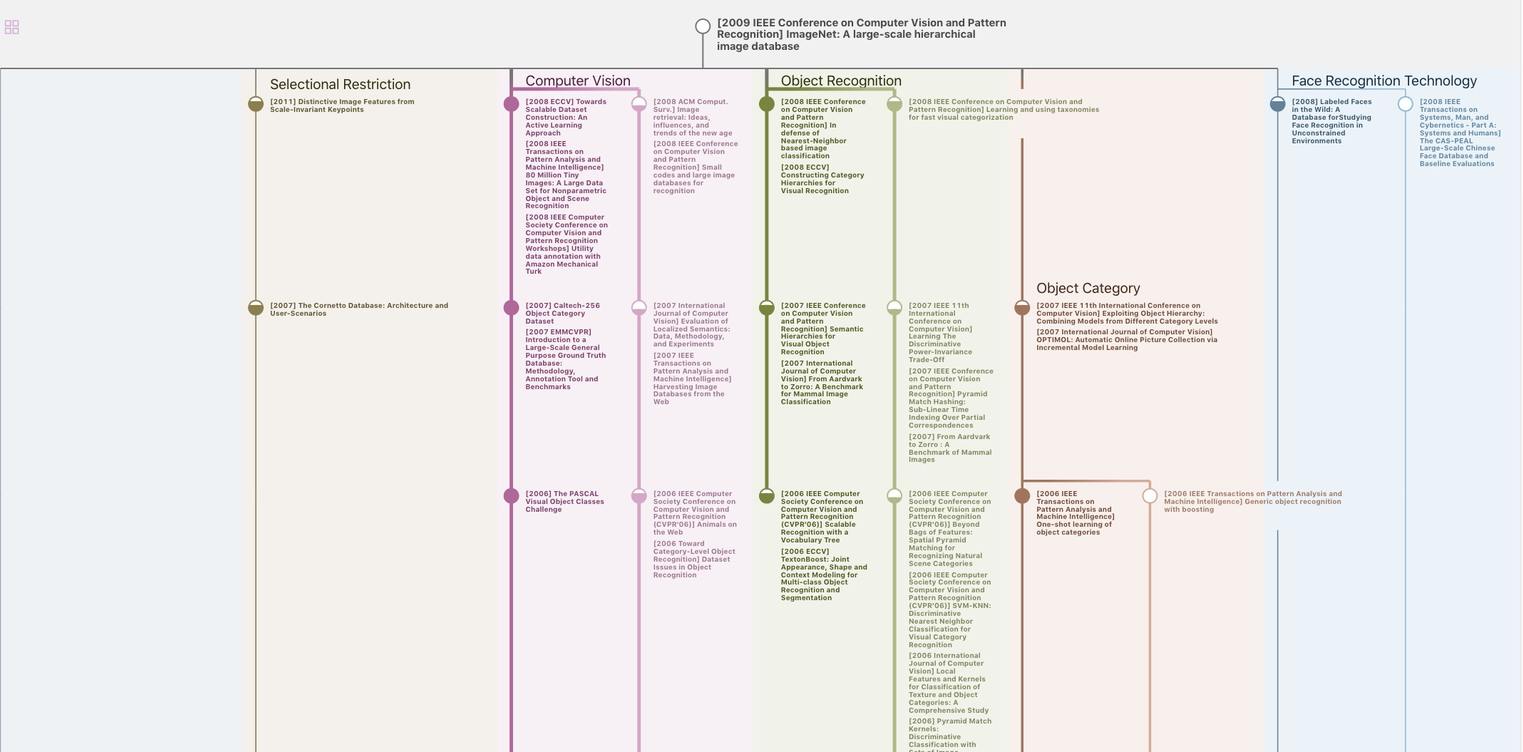
生成溯源树,研究论文发展脉络
Chat Paper
正在生成论文摘要