End-to-End Video Instance Segmentation with Transformers
2021 IEEE/CVF CONFERENCE ON COMPUTER VISION AND PATTERN RECOGNITION, CVPR 2021(2021)
摘要
Video instance segmentation (VIS) is the task that requires simultaneously classifying, segmenting and tracking object instances of interest in video. Recent methods typically develop sophisticated pipelines to tackle this task. Here, we propose a new video instance segmentation framework built upon Transformers, termed VisTR, which views the VIS task as a direct end-to-end parallel sequence decoding/prediction problem. Given a video clip consisting of multiple image frames as input, VisTR outputs the sequence of masks for each instance in the video in order directly. At the core is a new, effective instance sequence matching and segmentation strategy, which supervises and segments instances at the sequence level as a whole. VisTR frames the instance segmentation and tracking in the same perspective of similarity learning, thus considerably simplifying the overall pipeline and is significantly different from existing approaches. Without bells and whistles, VisTR achieves the highest speed among all existing VIS models, and achieves the best result among methods using single model on the YouTube-VIS dataset. For the first time, we demonstrate a much simpler and faster video instance segmentation framework built upon Transformers, achieving competitive accuracy. We hope that VisTR can motivate future research for more video understanding tasks. Code is available at: https://git . io/VisTR
更多查看译文
关键词
end-to-end video instance segmentation,Transformers,termed VisTR,VIS task,direct end-to-end,video clip,multiple image frames,effective instance sequence matching,segmentation strategy,supervises,segments instances,sequence level,VisTR frames,tracking,simpler video instance segmentation framework,faster video instance segmentation framework,video understanding tasks
AI 理解论文
溯源树
样例
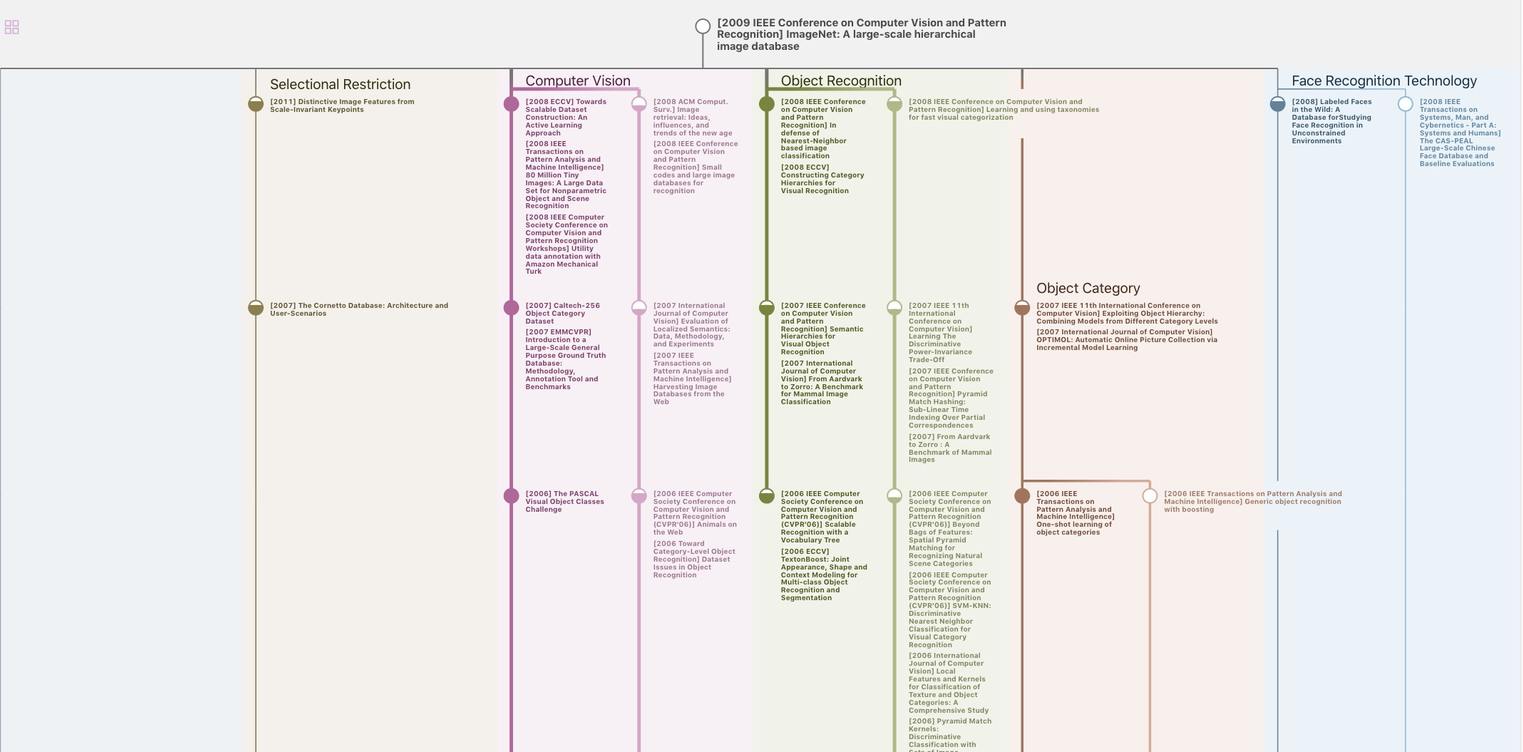
生成溯源树,研究论文发展脉络
Chat Paper
正在生成论文摘要