Accurate prediction of the signaling pathway activity elicited by variants of uncertain significance (VUS) and their drug sensitivity using deep convolutional neural networks on florescent microscopy images of cells
CANCER RESEARCH(2019)
摘要
Sequencing based methods to tailor the treatment of cancer patients are at the cutting edge of personalized medicine and have remarkably advanced in the past few years. However, a large number of mutations that are found when sequencing tumors are poorly characterized in the literature and are thus labeled as VUS. Because of their uncertain significance, it is not possible to assign them a function and hence are overlooked when designing treatment regimens, and this can have deleterious effects. Here we report the development of a Deep Convolutional Neural Network (DCNN) platform trained using Deep Learning algorithms, that is able to accurately predict the activity elicited by specific mutations. The DCNN is based on a high-throughput platform to predict the activation of signaling pathways elicited by the specific mutations via changes to the intra-cellular localization of fluorescently labeled protein reporters. For our training set we used images of 6 billion cells expressing either WT or mutations with known activity of the analyzed genes. After training, the network was presented with images of cells transfected with VUS that the network has never seen before. We show that the network was able to correctly predict the level of activity of a large number of VUS in KRAS, BRAF and cKIT and on multiple signaling pathways, specifically the MAPK and JAK-STAT pathways. These findings were further confirmed by both biochemical assays as well as literature search. Furthermore, images of cells expressing known mutations of BRAF and cKIT and treated with increasing doses of the targeted treatments Vemurafenib and Imatinib, respectively, were analyzed by the network and we were able to generate high resolution dose response curves in specific signaling pathways. Lastly, we present the predicted dose response curves in specific signaling pathways of a variety of VUS which have never been characterized before and show that different VUS respond differently to the tested drugs. Overall, we provide evidence that this novel tool enables robust and highly accurate calculation of activity profiles of a wide range of genes and signaling pathways. Each mutation is highly specific in the magnitude of activity it elicits. The high degree of resolution allows the investigation of the efficacy of different targeted therapies on a wide range of mutations. Citation Format: Lior Zimmerman, Arie Aizenman, Yutti Daitsh, Zohar Barbash, Gabi Tarcic, Michael Vidne. Accurate prediction of the signaling pathway activity elicited by variants of uncertain significance (VUS) and their drug sensitivity using deep convolutional neural networks on florescent microscopy images of cells [abstract]. In: Proceedings of the American Association for Cancer Research Annual Meeting 2019; 2019 Mar 29-Apr 3; Atlanta, GA. Philadelphia (PA): AACR; Cancer Res 2019;79(13 Suppl):Abstract nr 2447.
更多查看译文
AI 理解论文
溯源树
样例
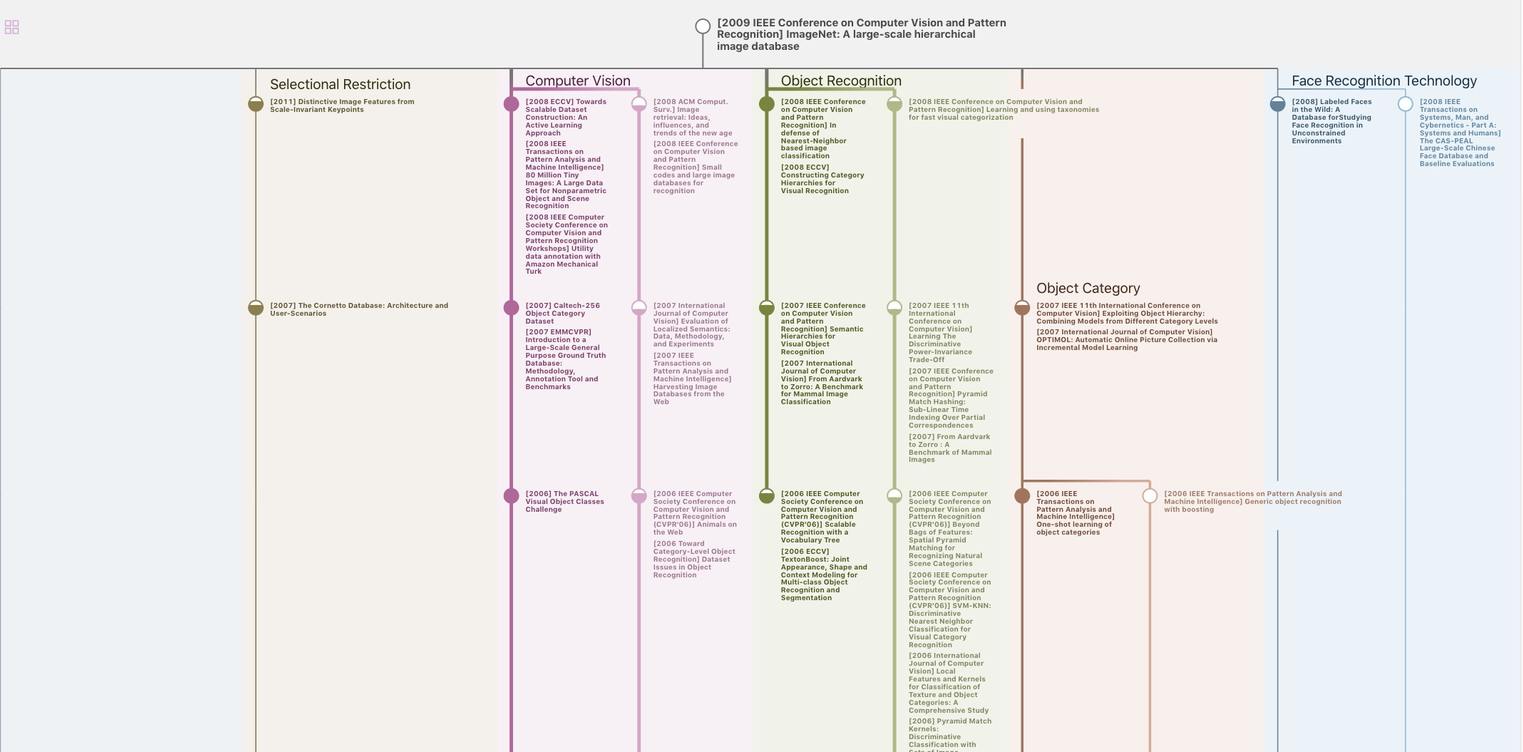
生成溯源树,研究论文发展脉络
Chat Paper
正在生成论文摘要