Deep Efficient End-To-End Reconstruction (Deer) Network For Few-View Breast Ct Image Reconstruction
IEEE ACCESS(2020)
摘要
Breast CT provides image volumes with isotropic resolution in high contrast, enabling detection of small calcification (down to a few hundred microns in size) and subtle density differences. Since breast is sensitive to x-ray radiation, dose reduction of breast CT is an important topic, and for this purpose, few-view scanning is a main approach. In this article, we propose a Deep Efficient End-to-end Reconstruction (DEER) network for few-view breast CT image reconstruction. The major merits of our network include high dose efficiency, excellent image quality, and low model complexity. By the design, the proposed network can learn the reconstruction process with as few as O(N) parameters, where N is the side length of an image to be reconstructed, which represents orders of magnitude improvements relative to the state-of-the-art deep-learning-based reconstruction methods that map raw data to tomographic images directly. Also, validated on a cone-beam breast CT dataset prepared by Koning Corporation on a commercial scanner, our method demonstrates a competitive performance over the state-of-the-art reconstruction networks in terms of image quality. The source code of this paper is available at: https://github.com/HuidongXie/DEER.
更多查看译文
关键词
Breast CT, deep learning, few-view CT, low-dose CT, X-ray CT
AI 理解论文
溯源树
样例
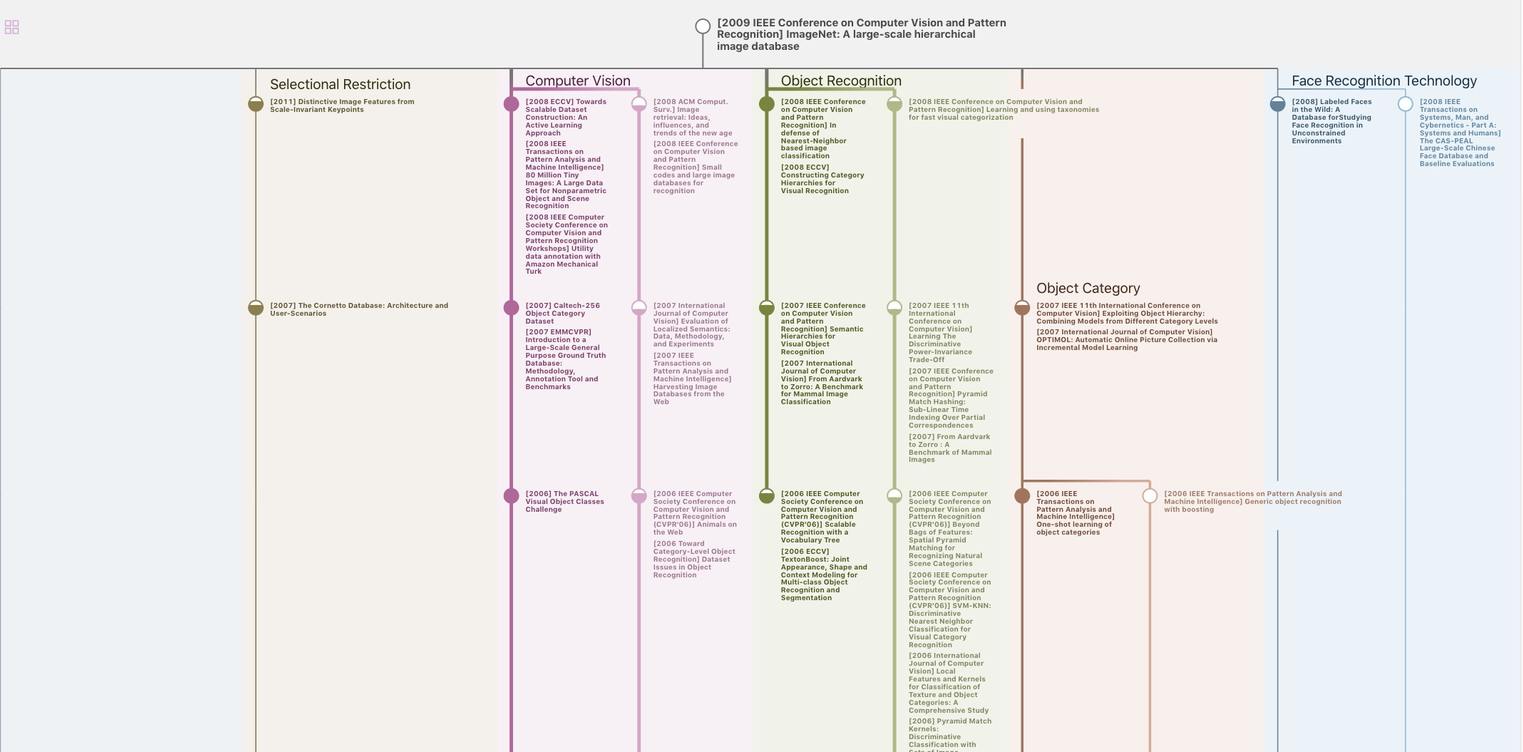
生成溯源树,研究论文发展脉络
Chat Paper
正在生成论文摘要