Approximate Midpoint Policy Iteration for Linear Quadratic Control
L4DC(2021)
摘要
We present a midpoint policy iteration algorithm to solve linear quadratic optimal control problems in both model-based and model-free settings. The algorithm is a variation of Newton's method, and we show that in the model-based setting it achieves cubic convergence, which is superior to standard policy iteration and policy gradient algorithms that achieve quadratic and linear convergence, respectively. We also demonstrate that the algorithm can be approximately implemented without knowledge of the dynamics model by using least-squares estimates of the state-action value function from trajectory data, from which policy improvements can be obtained. With sufficient trajectory data, the policy iterates converge cubically to approximately optimal policies, and this occurs with the same available sample budget as the approximate standard policy iteration. Numerical experiments demonstrate effectiveness of the proposed algorithms.
更多查看译文
关键词
control,iteration
AI 理解论文
溯源树
样例
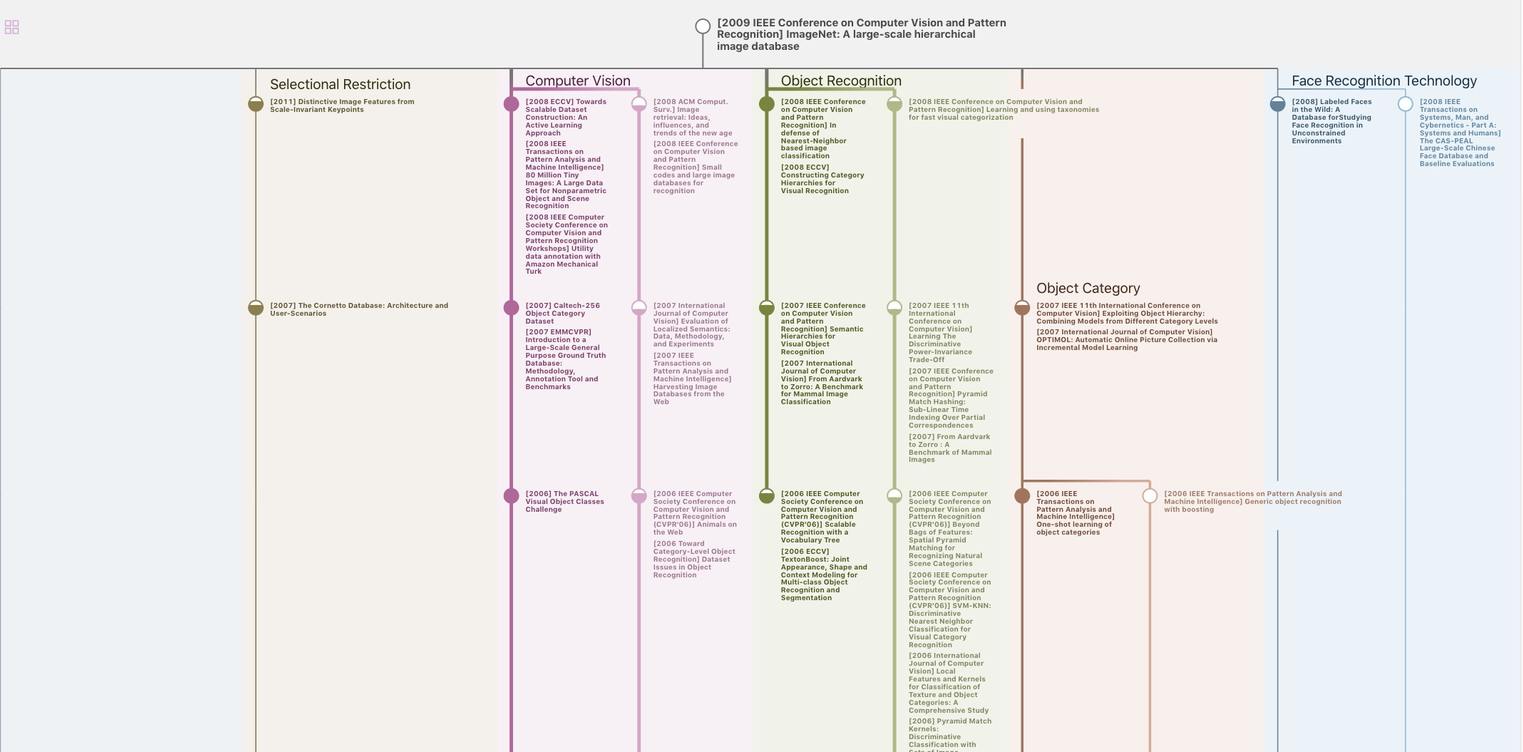
生成溯源树,研究论文发展脉络
Chat Paper
正在生成论文摘要