Adaptively weighted group Lasso for semiparametric quantile regression models
BERNOULLI(2019)
摘要
We propose an adaptively weighted group Lasso procedure for simultaneous variable selection and structure identification for varying coefficient quantile regression models and additive quantile regression models with ultra-high dimensional covariates. Under a strong sparsity condition, we establish selection consistency of the proposed Lasso procedure when the weights therein satisfy a set of general conditions. This consistency result, however, is reliant on a suitable choice of the tuning parameter for the Lasso penalty, which can be hard to make in practice. To alleviate this difficulty, we suggest a BIC-type criterion, which we call high-dimensional information criterion (HDIC), and show that the proposed Lasso procedure with the tuning parameter determined by HDIC still achieves selection consistency. Our simulation studies support strongly our theoretical findings.
更多查看译文
关键词
additive models,B-spline,high-dimensional information criteria,Lasso,structure identification,varying coefficient models
AI 理解论文
溯源树
样例
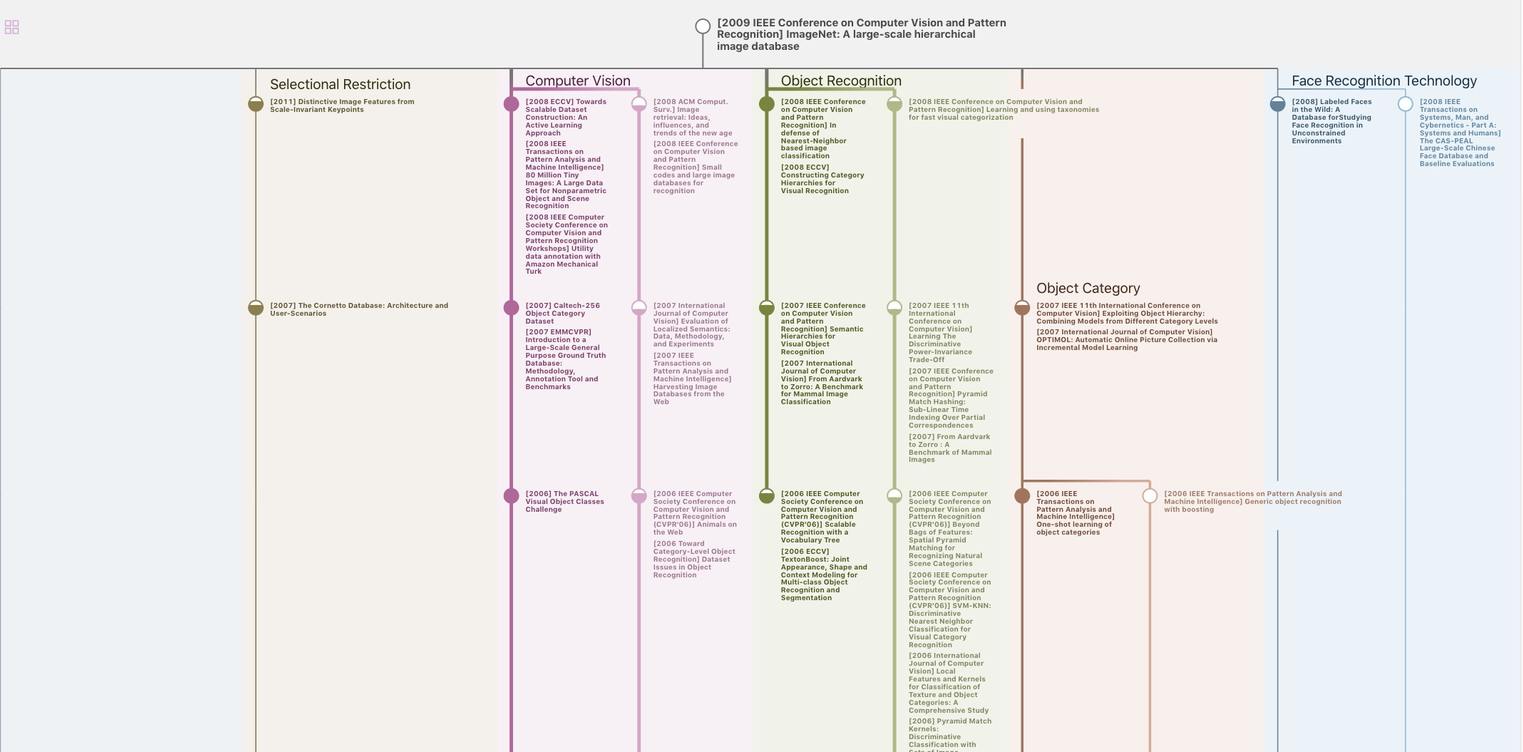
生成溯源树,研究论文发展脉络
Chat Paper
正在生成论文摘要