Unsupervised Part Representation by Flow Capsules
INTERNATIONAL CONFERENCE ON MACHINE LEARNING, VOL 139(2021)
摘要
Capsule networks aim to parse images into a hierarchy of objects, parts and relations. While promising, they remain limited by an inability to learn effective low level part descriptions. To address this issue we propose a way to learn primary capsule encoders that detect atomic parts from a single image. During training we exploit motion as a powerful perceptual cue for part definition, with an expressive decoder for part generation within a layered image model with occlusion. Experiments demonstrate robust part discovery in the presence of multiple objects, cluttered backgrounds, and occlusion. The part decoder infers the underlying shape masks, effectively filling in occluded regions of the detected shapes. We evaluate FlowCapsules on unsupervised part segmentation and unsupervised image classification.
更多查看译文
关键词
part representation,flow capsules
AI 理解论文
溯源树
样例
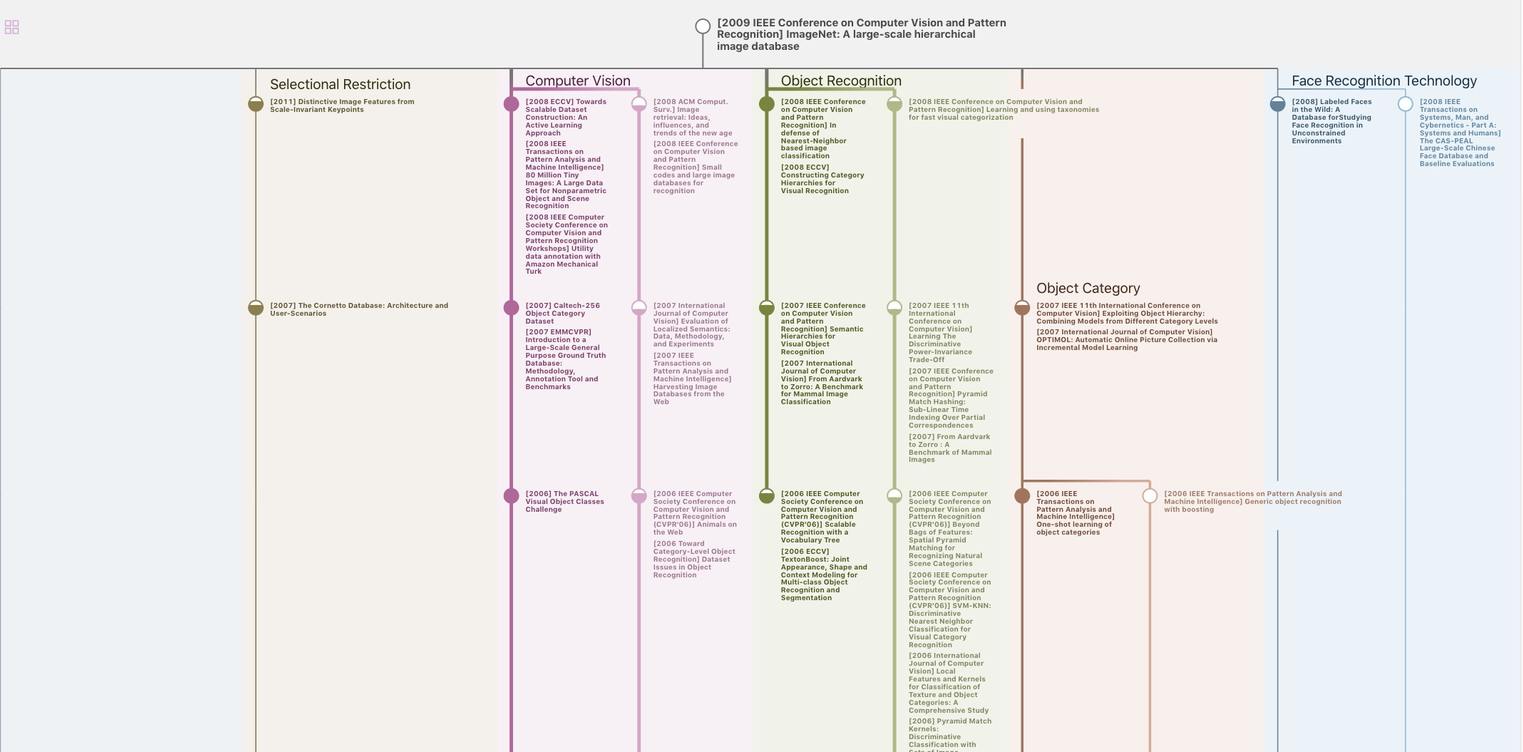
生成溯源树,研究论文发展脉络
Chat Paper
正在生成论文摘要