Denoising Non-Steady State Dynamic Pet Data Using A Feed-Forward Neural Network
PHYSICS IN MEDICINE AND BIOLOGY(2021)
摘要
The quality of reconstructed dynamic PET images, as well as the statistical reliability of the estimated pharmacokinetic parameters is often compromised by high levels of statistical noise, particularly at the voxel level. Many denoising strategies have been proposed, both in the temporal and spatial domain, which substantially improve the signal to noise ratio of the reconstructed dynamic images. However, although most filtering approaches are fairly successful in reducing the spatio-temporal inter-voxel variability, they may also average out or completely eradicate the critically important temporal signature of a transient neurotransmitter activation response that may be present in a non-steady state dynamic PET study. In this work, we explore an approach towards temporal denoising of non-steady state dynamic PET images using an artificial neural network, which was trained to identify the temporal profile of a time-activity curve, while preserving any potential activation response. We evaluated the performance of a feed-forward perceptron neural network to improve the signal to noise ratio of dynamic [C-11]raclopride activation studies and compared it with the widely used highly constrained back projection (HYPR) filter. Results on both simulated Geant4 Application for Tomographic Emission data of a realistic rat brain phantom and experimental animal data of a freely moving animal study showed that the proposed neural network can efficiently improve the noise characteristics of dynamic data in the temporal domain, while it can lead to a more reliable estimation of voxel-wise activation response in target region. In addition, improvements in signal-to-noise ratio achieved by denoising the dynamic data using the proposed neural network led to improved accuracy and precision of the estimated model parameters of the lp-ntPET model, compared to the HYPR filter. The performance of the proposed denoising approach strongly depends on the amount of noise in the dynamic PET data, with higher noise leading to substantially higher variability in the estimated parameters of the activation response. Overall, the feed-forward network led to a similar performance as the HYPR filter in terms of spatial denoising, but led to notable improvements in terms of temporal denoising, which in turn improved the estimation activation parameters.
更多查看译文
关键词
pharmacokinetic modelling, non-steady state kinetics, artificial neural networks, neurotransmitter release
AI 理解论文
溯源树
样例
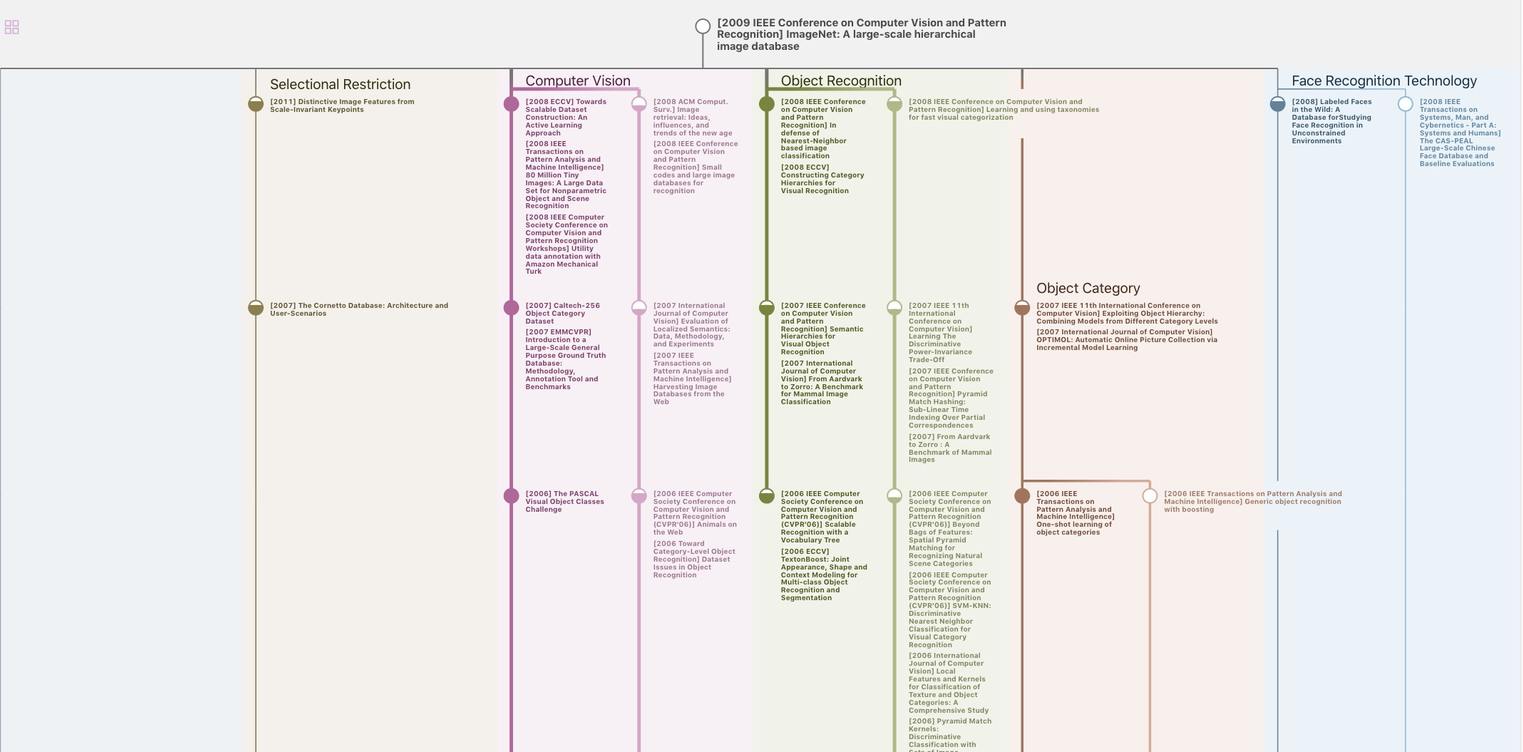
生成溯源树,研究论文发展脉络
Chat Paper
正在生成论文摘要