Deep-Learning-Assisted multivariate curve resolution
JOURNAL OF CHROMATOGRAPHY A(2021)
摘要
Gas chromatography-mass spectrometry (GC-MS) is one of the major platforms for analyzing volatile compounds in complex samples. However, automatic and accurate extraction of qualitative and quantitative information is still challenging when analyzing complex GC-MS data, especially for the components incompletely separated by chromatography. Deep-Learning-Assisted Multivariate Curve Resolution (DeepResolution) was proposed in this study. It essentially consists of convolutional neural networks (CNN) models to determine the number of components of each overlapped peak and the elution region of each compound. With the assistance of the predicted elution regions, the informative regions (such as selective region and zero-concentration region) of each compound can be located precisely. Then, full rank resolution (FRR), multivariate curve resolution-alternating least squares (MCR-ALS) or iterative target transformation factor analysis (ITTFA) can be chosen adaptively to resolve the overlapped components without manual intervention. The results showed that DeepResolution has superior compound identification capability and better quantitative performances when comparing with MS-DIAL, ADAP-GC and AMDIS. It was also found that baseline levels, interferents, component concentrations and peak tailing have little influences on resolution result. Besides, DeepResolution can be extended easily when encountering unknown component(s), due to the independence of each CNN model. All procedures of DeepResolution can be performed automatically, and adaptive selection of resolution methods ensures the balance between resolution power and consumed time. (C) 2020 Published by Elsevier B.V.
更多查看译文
关键词
Deep Learning,GC-MS,Multivariate Curve Resolution
AI 理解论文
溯源树
样例
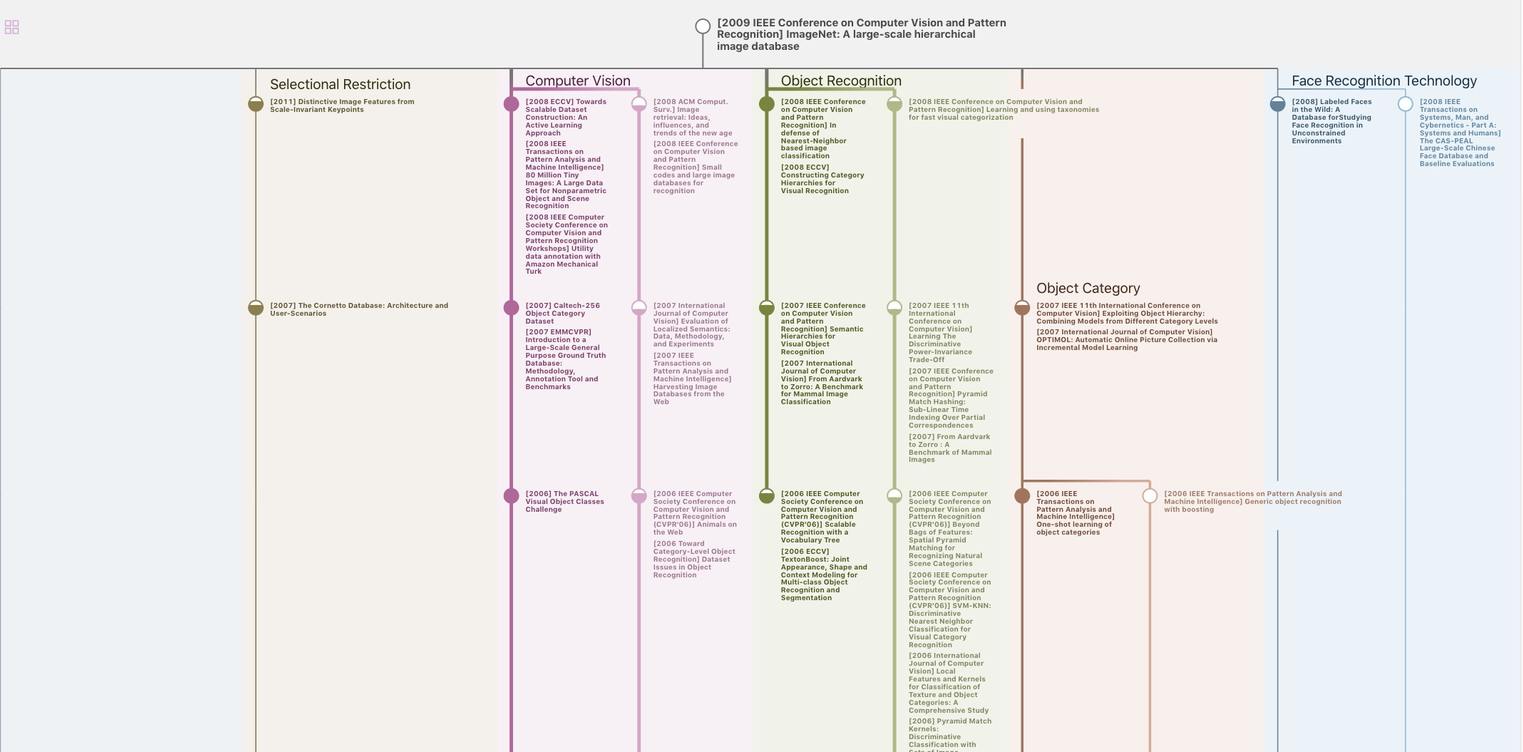
生成溯源树,研究论文发展脉络
Chat Paper
正在生成论文摘要