Remaining Useful Life Prognosis for Turbofan Engine Using Explainable Deep Neural Networks with Dimensionality Reduction.
SENSORS(2020)
摘要
This study prognoses the remaining useful life of a turbofan engine using a deep learning model, which is essential for the health management of an engine. The proposed deep learning model affords a significantly improved accuracy by organizing networks with a one-dimensional convolutional neural network, long short-term memory, and bidirectional long short-term memory. In particular, this paper investigates two practical and crucial issues in applying the deep learning model for system prognosis. The first is the requirement of numerous sensors for different components, i.e., the curse of dimensionality. Second, the deep neural network cannot identify the problematic component of the turbofan engine due to its "black box" property. This study thus employs dimensionality reduction and Shapley additive explanation (SHAP) techniques. Dimensionality reduction in the model reduces the complexity and prevents overfitting, while maintaining high accuracy. SHAP analyzes and visualizes the black box to identify the sensors. The experimental results demonstrate the high accuracy and efficiency of the proposed model with dimensionality reduction and show that SHAP enhances the explainability in a conventional deep learning model for system prognosis.
更多查看译文
关键词
deep neural network,dimensionality reduction,explainable artificial intelligence,feature selection,prognostics and health monitoring,turbofan engine
AI 理解论文
溯源树
样例
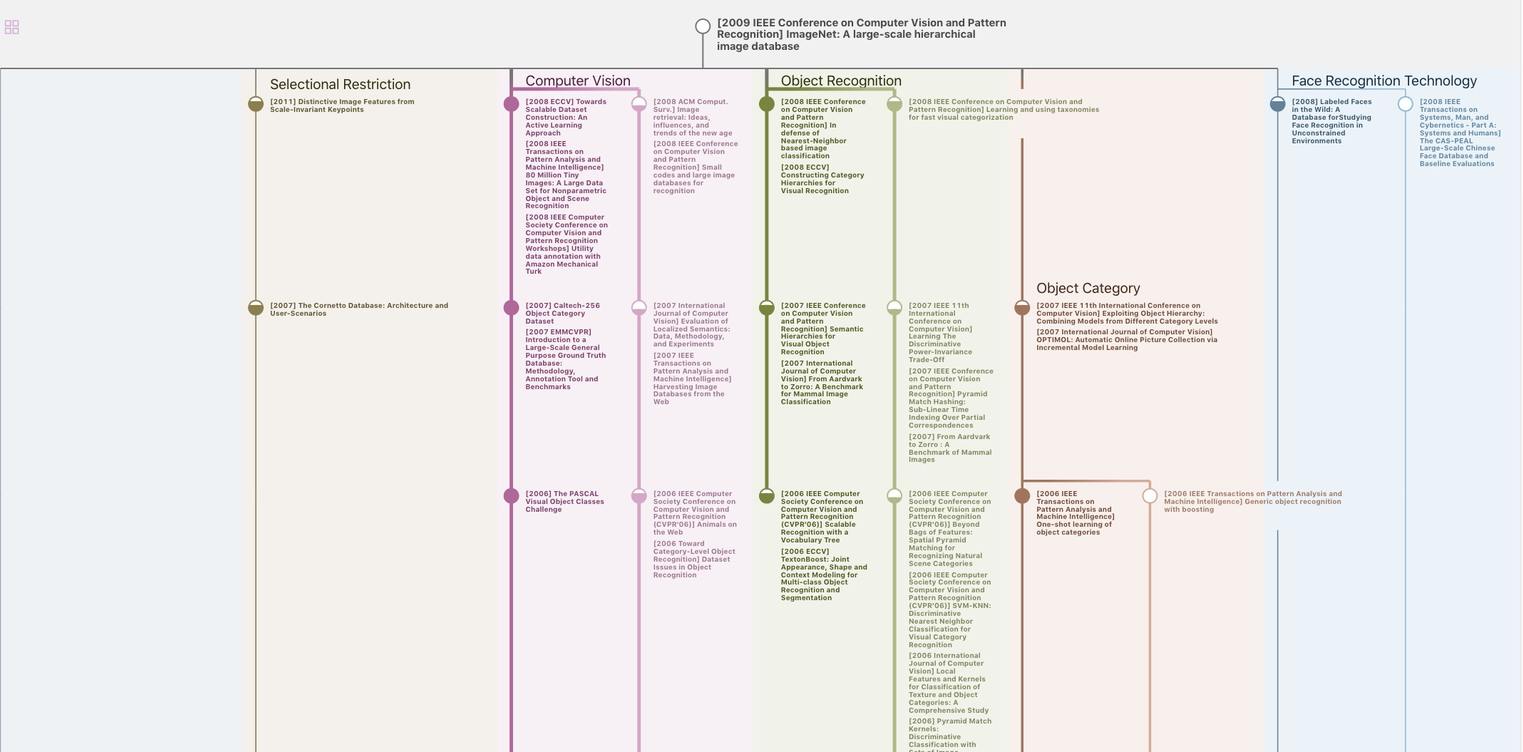
生成溯源树,研究论文发展脉络
Chat Paper
正在生成论文摘要