Right for the Right Concept: Revising Neuro-Symbolic Concepts by Interacting with their Explanations
2021 IEEE/CVF CONFERENCE ON COMPUTER VISION AND PATTERN RECOGNITION, CVPR 2021(2021)
摘要
Most explanation methods in deep learning map importance estimates for a model’s prediction back to the original input space. These "visual" explanations are often insufficient, as the model’s actual concept remains elusive. Moreover, without insights into the model’s semantic concept, it is difficult —if not impossible— to intervene on the model’s behavior via its explanations, called Explanatory Interactive Learning. Consequently, we propose to intervene on a Neuro-Symbolic scene representation, which allows one to revise the model on the semantic level, e.g. "never focus on the color to make your decision". We compiled a novel confounded visual scene data set, the CLEVR-Hans data set, capturing complex compositions of different objects. The results of our experiments on CLEVR-Hans demonstrate that our semantic explanations, i.e. compositional explanations at a per-object level, can identify confounders that are not identifiable using "visual" explanations only. More importantly, feedback on this semantic level makes it possible to revise the model from focusing on these factors.
更多查看译文
关键词
Explanatory Interactive Learning,original input space,deep learning map importance,Neuro-Symbolic concepts,semantic level,per-object level,compositional explanations,semantic explanations,CLEVR-Hans data,visual scene data set,Neuro-Symbolic scene representation,visual explanations
AI 理解论文
溯源树
样例
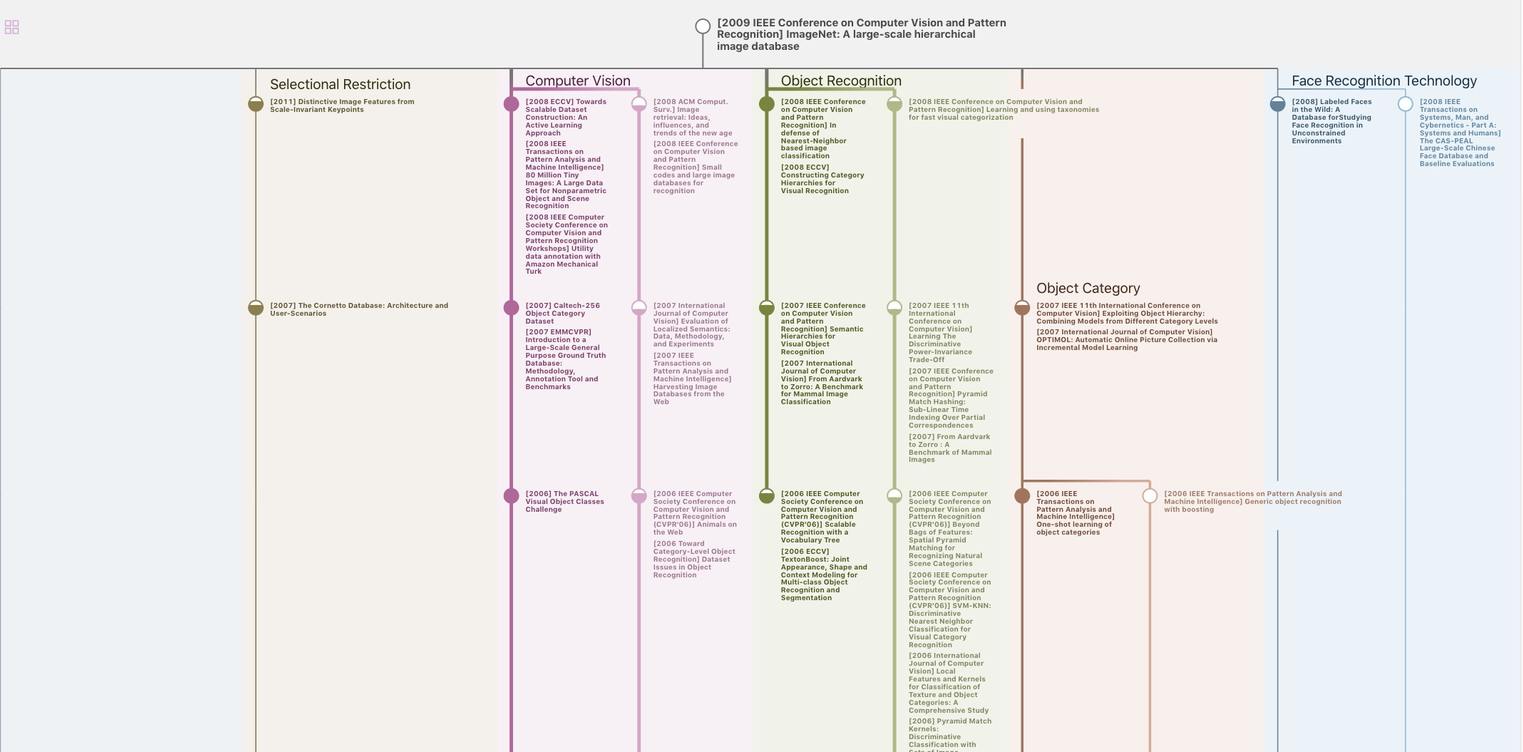
生成溯源树,研究论文发展脉络
Chat Paper
正在生成论文摘要