Image Inpainting with Contextual Reconstruction Loss
arxiv(2020)
摘要
Convolutional neural networks (CNNs) have been observed to be inefficient in propagating information across distant spatial positions in images. Recent studies in image inpainting attempt to overcome this issue by explicitly searching reference regions throughout the entire image to fill the features from reference regions in the missing regions. This operation can be implemented as contextual attention layer (CA layer) \cite{yu2018generative}, which has been widely used in many deep learning-based methods. However, it brings significant computational overhead as it computes the pair-wise similarity of feature patches at every spatial position. Also, it often fails to find proper reference regions due to the lack of supervision in terms of the correspondence between missing regions and known regions. We propose a novel contextual reconstruction loss (CR loss) to solve these problems. First, a criterion of searching reference region is designed based on minimizing reconstruction and adversarial losses corresponding to the searched reference and the ground-truth image. Second, unlike previous approaches which integrate the computationally heavy patch searching and replacement operation in the inpainting model, CR loss encourages a vanilla CNN to simulate this behavior during training, thus no extra computations are required during inference. Experimental results demonstrate that the proposed inpainting model with the CR loss compares favourably against the state-of-the-arts in terms of quantitative and visual performance. Code is available at \url{https://github.com/zengxianyu/crfill}.
更多查看译文
关键词
reconstruction,image
AI 理解论文
溯源树
样例
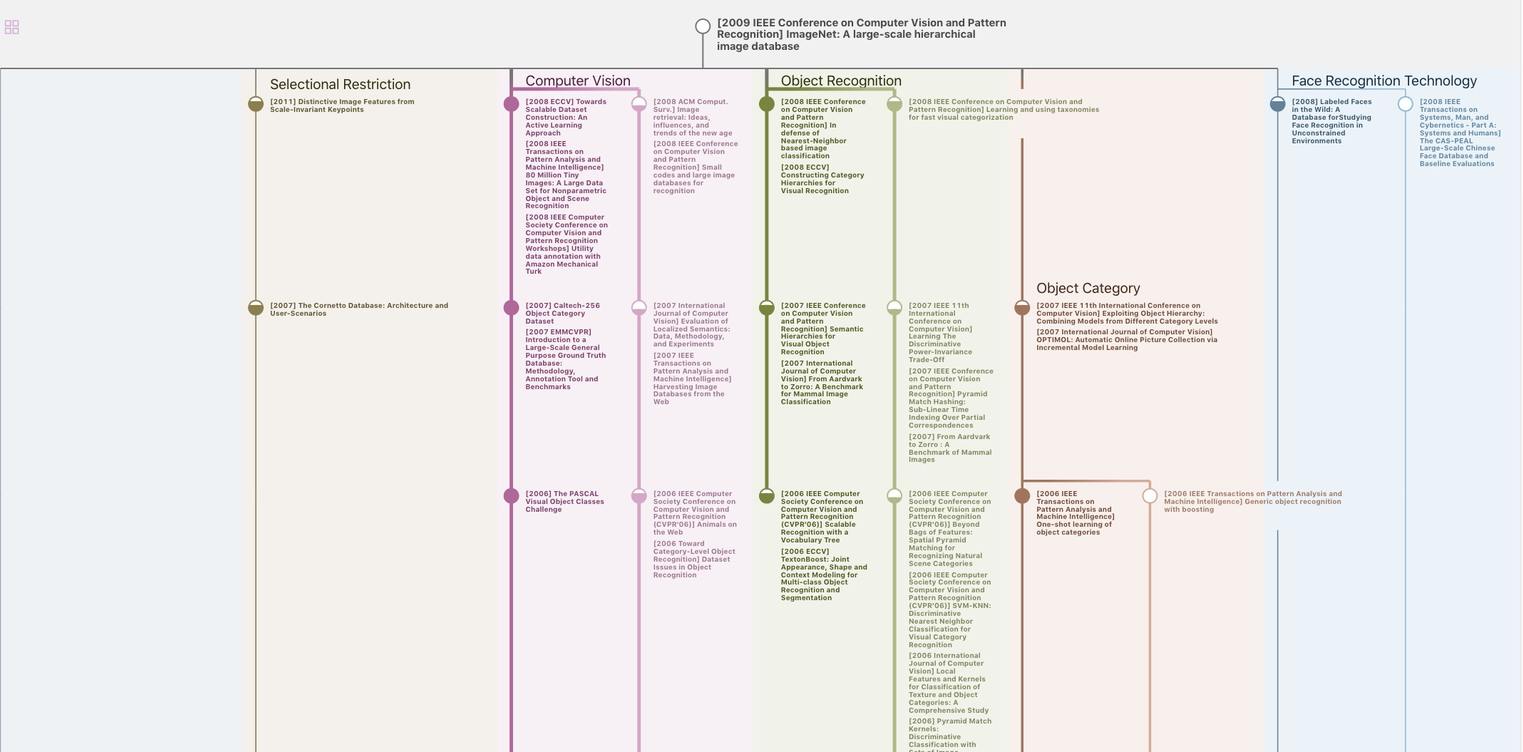
生成溯源树,研究论文发展脉络
Chat Paper
正在生成论文摘要