Combining Semantic Guidance and Deep Reinforcement Learning For Generating Human Level Paintings
2021 IEEE/CVF CONFERENCE ON COMPUTER VISION AND PATTERN RECOGNITION, CVPR 2021(2021)
摘要
Generation of stroke-based non-photorealistic imagery, is an important problem in the computer vision community. As an endeavor in this direction, substantial recent research efforts have been focused on teaching machines "how to paint", in a manner similar to a human painter. However, the applicability of previous methods has been limited to datasets with little variation in position, scale and saliency of the foreground object. As a consequence, we find that these methods struggle to cover the granularity and diversity possessed by real world images. To this end, we propose a Semantic Guidance pipeline with 1) a bi-level painting procedure for learning the distinction between foreground and background brush strokes at training time. 2) We also introduce invariance to the position and scale of the foreground object through a neural alignment model, which combines object localization and spatial transformer networks in an end to end manner, to zoom into a particular semantic instance. 3) The distinguishing features of the in-focus object are then amplified by maximizing a novel guided backpropagation based focus reward. The proposed agent does not require any supervision on human stroke-data and successfully handles variations in foreground object attributes, thus, producing much higher quality canvases for the CUB-200 Birds [29] and Stanford Cars-196 [17] datasets. Finally, we demonstrate the further efficacy of our method on complex datasets with multiple foreground object instances by evaluating an extension of our method on the challenging Virtual-KITTI [2] dataset. Source code and models are available at https://github.com/1jsingh/semantic-guidance.
更多查看译文
关键词
deep reinforcement learning,stroke-based nonphotorealistic imagery,computer vision community,teaching machines,human painter,saliency,granularity,Semantic Guidance pipeline,bi-level painting procedure,background brush strokes,training time,neural alignment model,object localization,spatial transformer networks,semantic instance,in-focus object,novel guided backpropagation based focus reward,human stroke-data,foreground object attributes,complex datasets,multiple foreground object instances,end to end manner,human level paintings
AI 理解论文
溯源树
样例
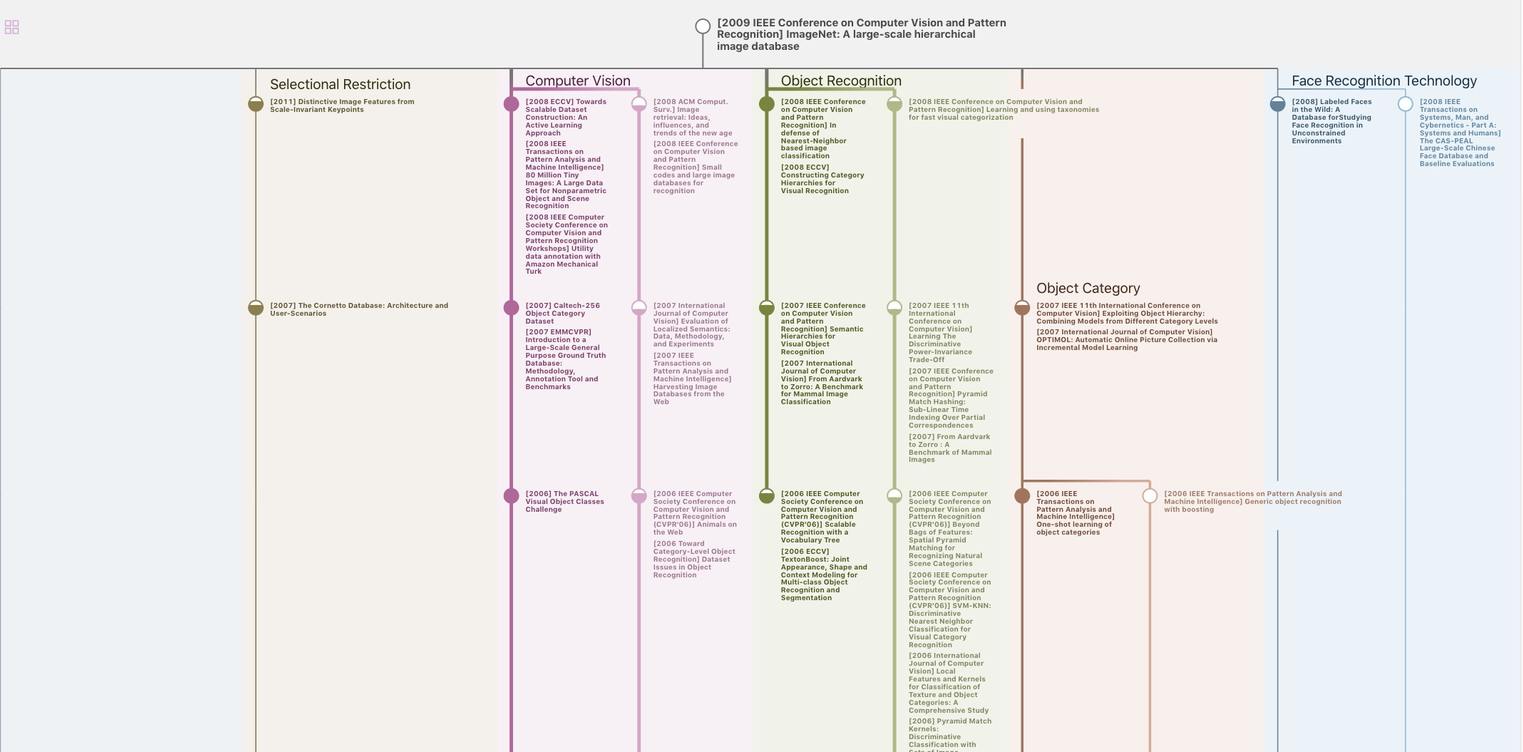
生成溯源树,研究论文发展脉络
Chat Paper
正在生成论文摘要