Reinforced optimal control
arxiv(2020)
摘要
Least squares Monte Carlo methods are a popular numerical approximation method for solving stochastic control problems. Based on dynamic programming, their key feature is the approximation of the conditional expectation of future rewards by linear least squares regression. Hence, the choice of basis functions is crucial for the accuracy of the method. Earlier work by some of us [Belomestny, Schoenmakers, Spokoiny, Zharkynbay. Commun.~Math.~Sci., 18(1):109-121, 2020] proposes to \emph{reinforce} the basis functions in the case of optimal stopping problems by already computed value functions for later times, thereby considerably improving the accuracy with limited additional computational cost. We extend the reinforced regression method to a general class of stochastic control problems, while considerably improving the method's efficiency, as demonstrated by substantial numerical examples as well as theoretical analysis.
更多查看译文
关键词
Monte Carlo,optimal control,regression,reinforcement learning
AI 理解论文
溯源树
样例
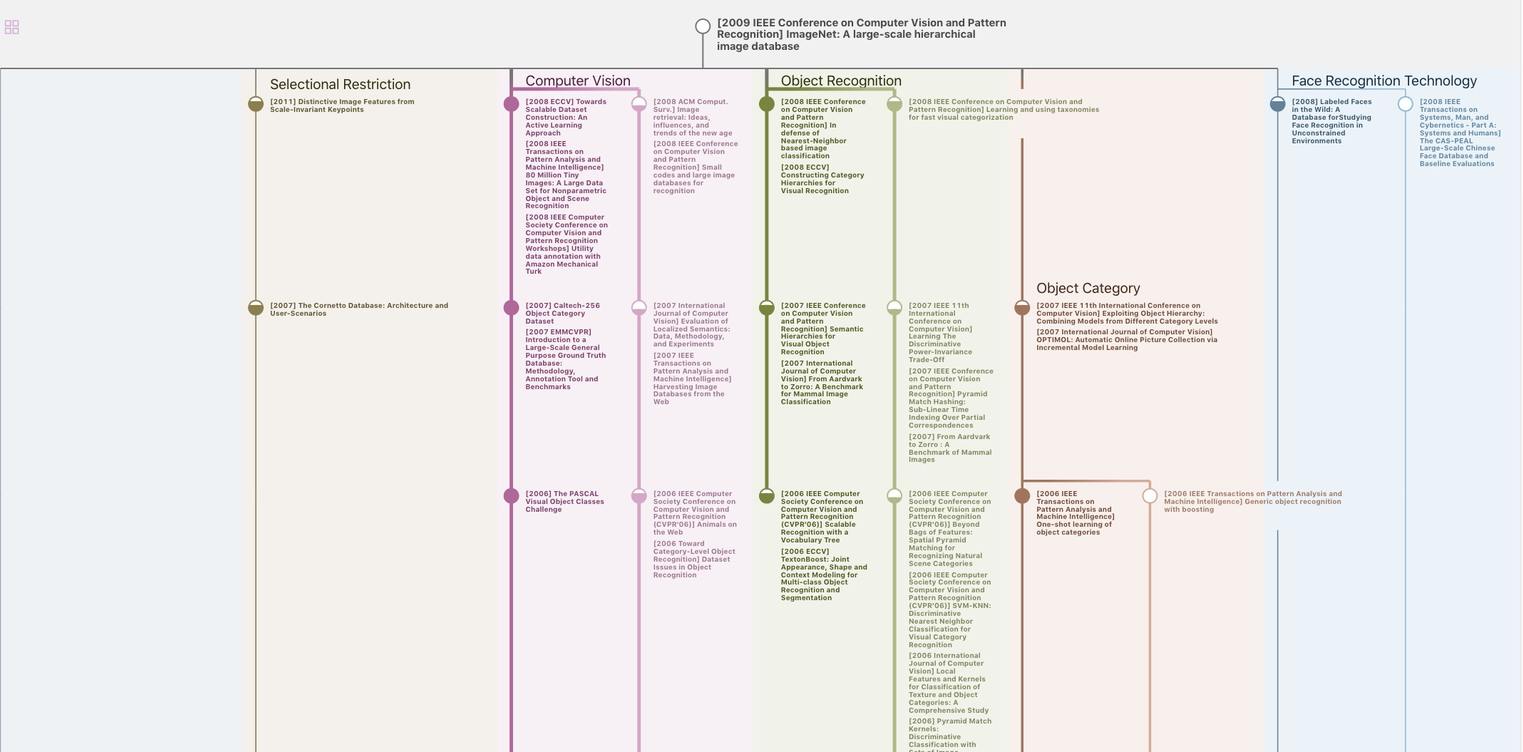
生成溯源树,研究论文发展脉络
Chat Paper
正在生成论文摘要