Semi-Supervised Gated Recurrent Neural Networks For Robotic Terrain Classification
IEEE ROBOTICS AND AUTOMATION LETTERS(2021)
摘要
Legged robots are popular candidates for missions in challenging terrains due to their versatile locomotion strategies. Terrain classification is a key enabling technology for autonomous legged robots, allowing them to harness their innate flexibility to adapt to the demands of their operating environment. We show how highly capable machine learning techniques, namely gated recurrent neural networks, allow our target legged robot to correctly classify the terrain it traverses in both supervised and semi-supervised fashions. Tests on a benchmark dataset shows that our time-domain classifiers are well capable of handling raw and variable-length data with small amount of labels and outperform frequency-domain classifiers. The classification results on our own extended dataset opens up a range of high-performance behaviours that are specific to those environments. Furthermore, we show how raw unlabelled data is used to improve significantly the classification results in a semi-supervised model.
更多查看译文
关键词
Deep learning methods, legged robots
AI 理解论文
溯源树
样例
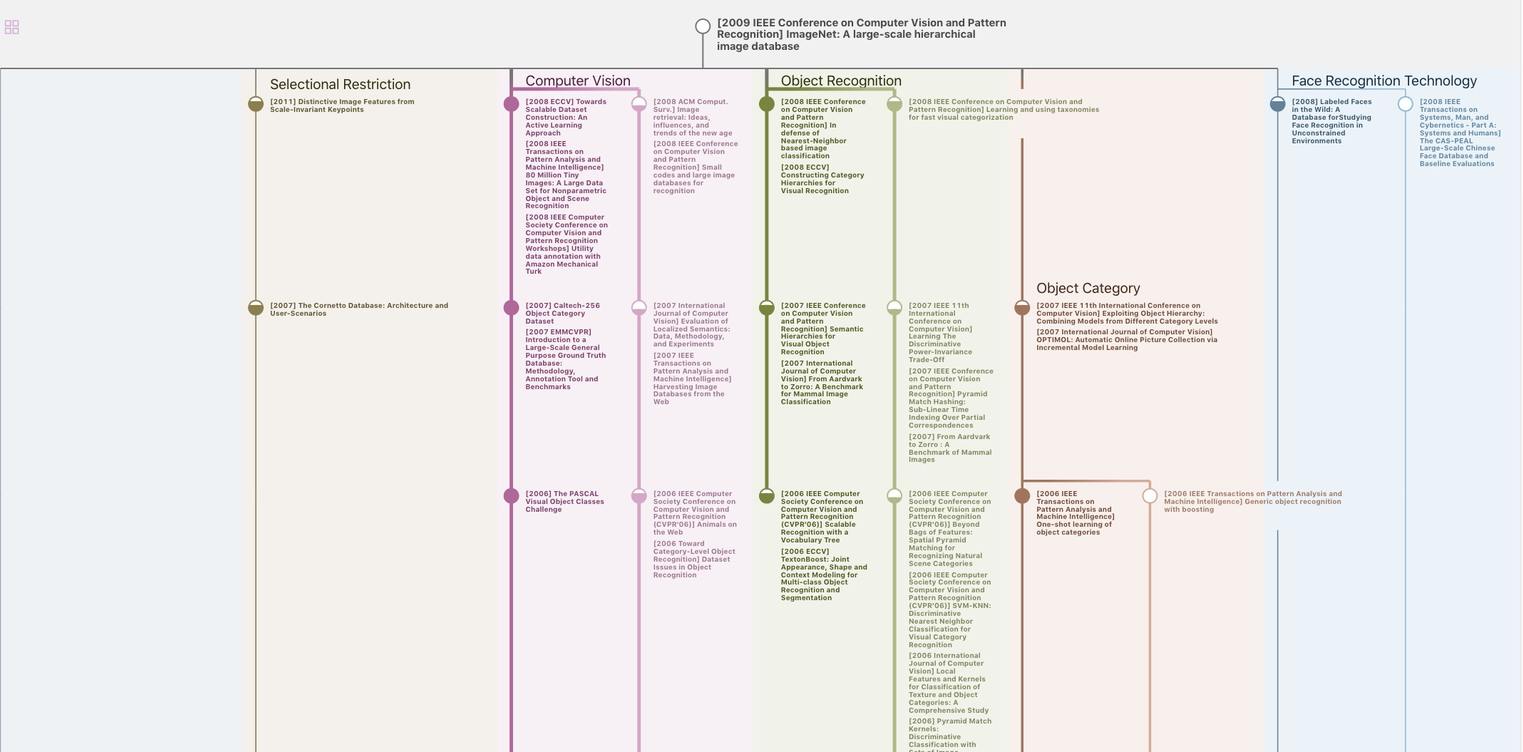
生成溯源树,研究论文发展脉络
Chat Paper
正在生成论文摘要