Latent Mechanisms of Polarization Switching from In Situ Electron Microscopy Observations
ADVANCED FUNCTIONAL MATERIALS(2022)
摘要
In situ scanning transmission electron microscopy enables observation of the domain dynamics in ferroelectric materials as a function of externally applied bias and temperature. The resultant data sets contain a wealth of information on polarization switching and phase transition mechanisms. However, identification of these mechanisms from observational data sets has remained a problem due to a large variety of possible configurations, many of which are degenerate. Here, an approach based on a combination of deep learning-based semantic segmentation, rotationally invariant variational autoencoder (VAE), and non-negative matrix factorization to enable learning of a latent space representation of the data with multiple real-space rotationally equivalent variants mapped to the same latent space descriptors is introduced. By varying the size of training sub-images in the VAE, the degree of complexity in the structural descriptors is tuned from simple domain wall detection to the identification of switching pathways. This yields a powerful tool for the exploration of the dynamic data in mesoscopic electron, scanning probe, optical, and chemical imaging. Moreover, this work adds to the growing body of knowledge of incorporating physical constraints into the machine and deep-learning methods to improve learned descriptors of physical phenomena.
更多查看译文
关键词
deep learning, electron microscopy, ferroelectric materials, latent variable models, semantic segmentation
AI 理解论文
溯源树
样例
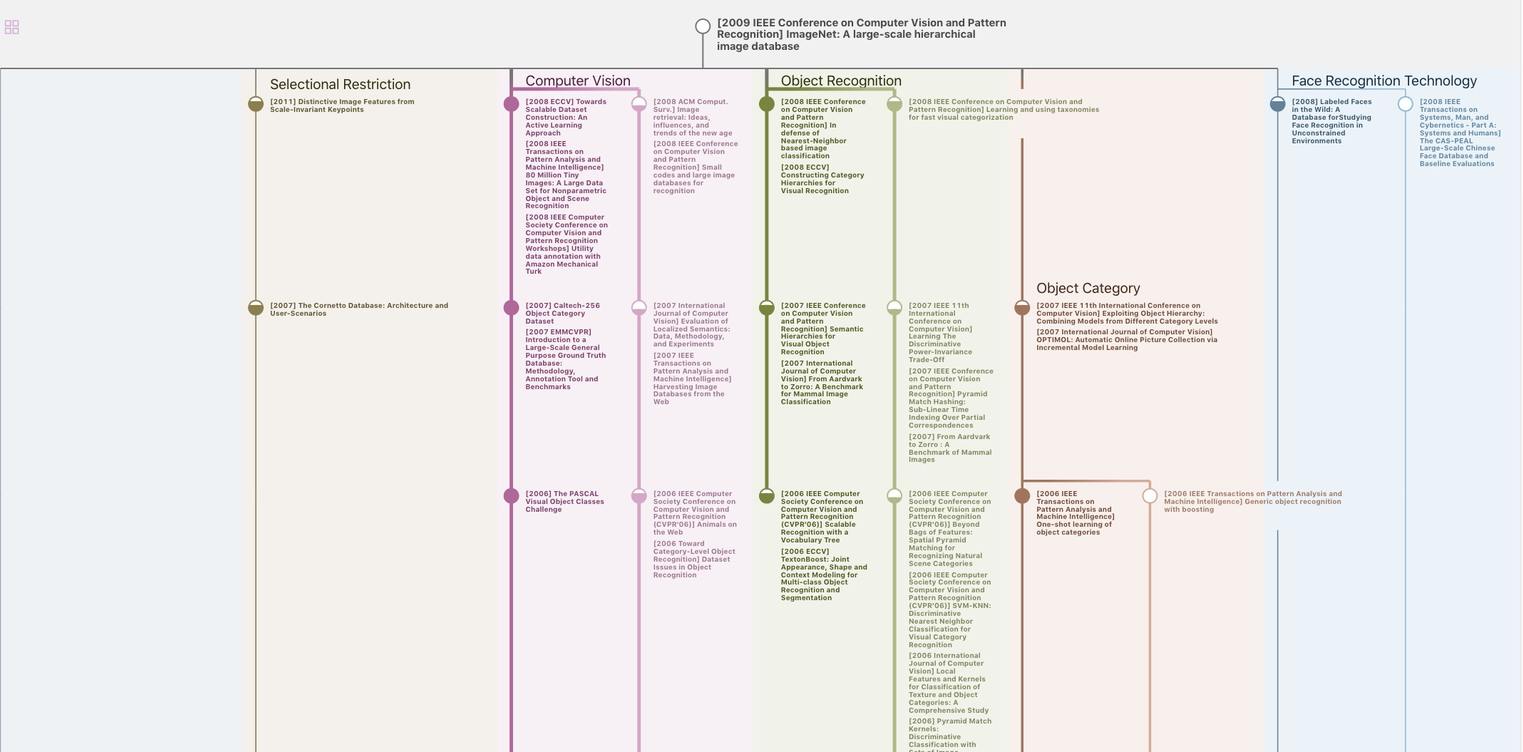
生成溯源树,研究论文发展脉络
Chat Paper
正在生成论文摘要