Augmented Lagrangian Adversarial Attacks.
ICCV(2021)
摘要
Adversarial attack algorithms are dominated by penalty methods, which are slow in practice, or more efficient distance-customized methods, which are heavily tailored to the properties of the considered distance. We propose a white-box attack algorithm to generate minimally perturbed adversarial examples based on Augmented Lagrangian principles. We bring several non-trivial algorithmic modifications, which have a crucial effect on performance. Our attack enjoys the generality of penalty methods and the computational efficiency of distance-customized algorithms, and can be readily used for a wide set of distances. We compare our attack to state-of-the-art methods on three datasets and several models, and consistently obtain competitive performances with similar or lower computational complexity.
更多查看译文
关键词
Adversarial learning,Optimization and learning methods
AI 理解论文
溯源树
样例
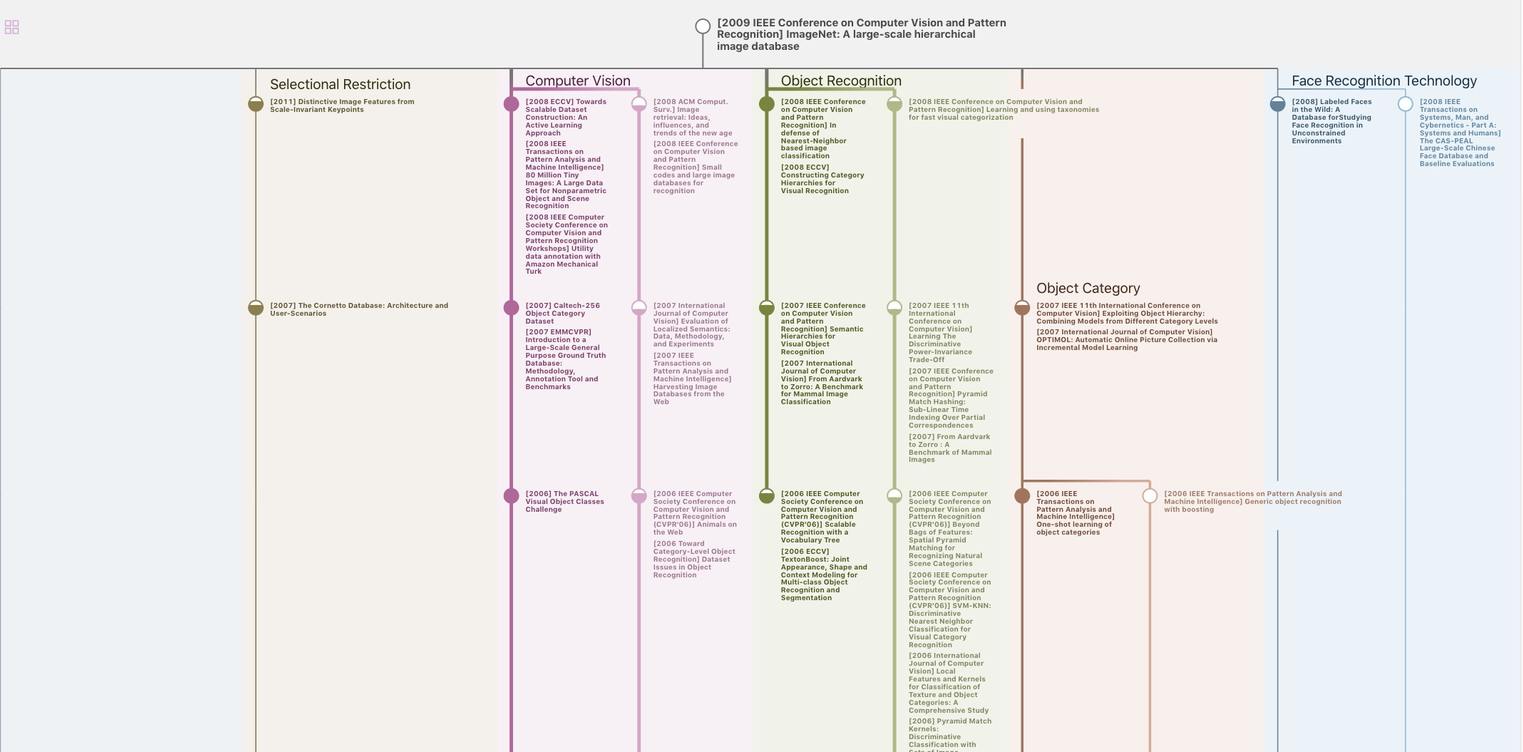
生成溯源树,研究论文发展脉络
Chat Paper
正在生成论文摘要