An Effective Anti-Aliasing Approach for Residual Networks
arxiv(2020)
摘要
Image pre-processing in the frequency domain has traditionally played a vital role in computer vision and was even part of the standard pipeline in the early days of deep learning. However, with the advent of large datasets, many practitioners concluded that this was unnecessary due to the belief that these priors can be learned from the data itself. Frequency aliasing is a phenomenon that may occur when sub-sampling any signal, such as an image or feature map, causing distortion in the sub-sampled output. We show that we can mitigate this effect by placing non-trainable blur filters and using smooth activation functions at key locations, particularly where networks lack the capacity to learn them. These simple architectural changes lead to substantial improvements in out-of-distribution generalization on both image classification under natural corruptions on ImageNet-C [10] and few-shot learning on Meta-Dataset [17], without introducing additional trainable parameters and using the default hyper-parameters of open source codebases.
更多查看译文
关键词
residual networks,anti-aliasing
AI 理解论文
溯源树
样例
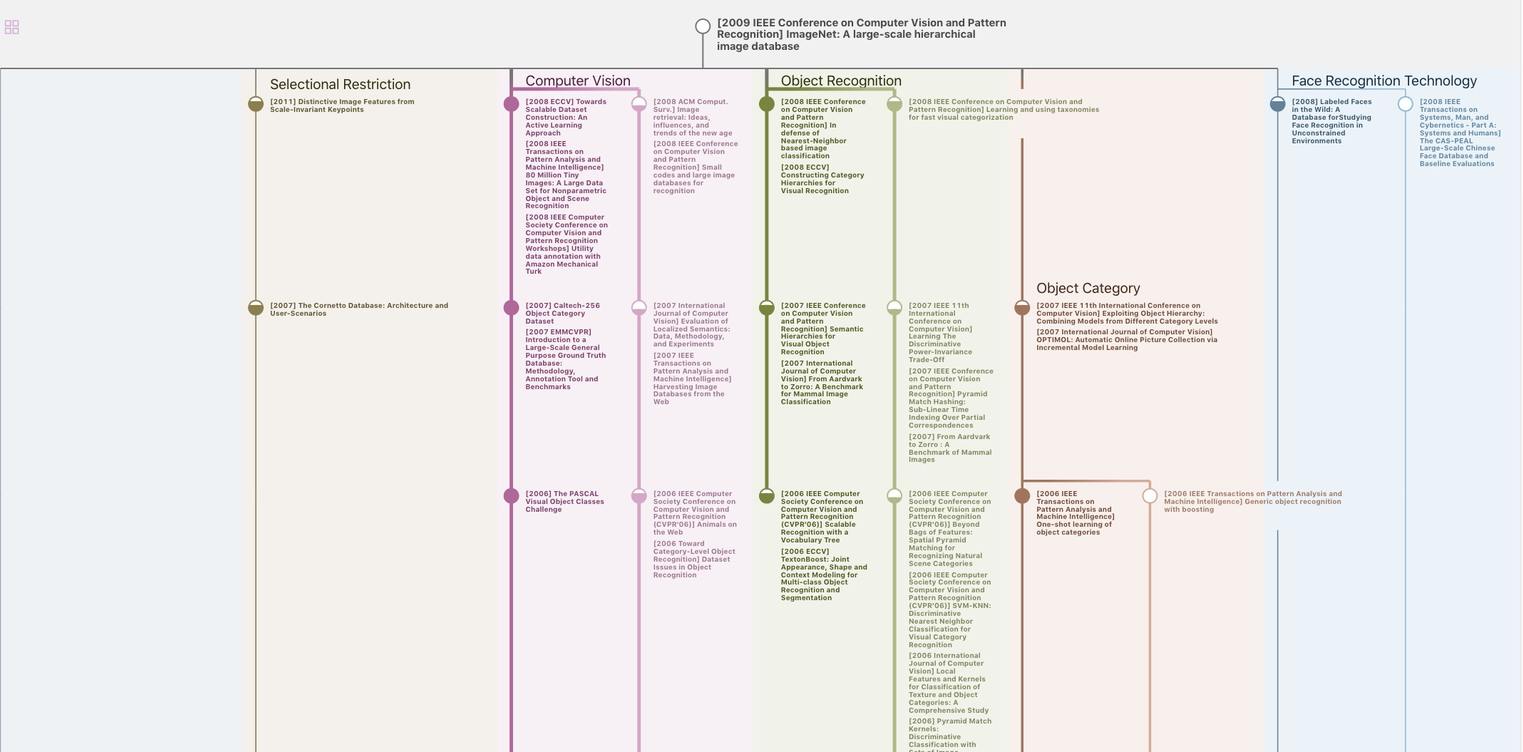
生成溯源树,研究论文发展脉络
Chat Paper
正在生成论文摘要