Graph Tikhonov Regularization and Interpolation Via Random Spanning Forests
IEEE Transactions on Signal and Information Processing over Networks(2021)
摘要
Novel Monte Carlo estimators are proposed to solve both the Tikhonov regularization (TR) and the interpolation problems on graphs. These estimators are based on random spanning forests (RSF), the theoretical properties of which enable to analyze the estimators' theoretical mean and variance. We also show how to perform hyperparameter tuning for these RSF-based estimators. TR is a component in many well-known algorithms, and we show how the proposed estimators can be easily adapted to avoid expensive intermediate steps in generalized semi-supervised learning, label propagation, Newton's method and iteratively reweighted least squares. In the experiments, we illustrate the proposed methods on several problems and provide observations on their run time.
更多查看译文
关键词
Graph signal processing,random spanning forests,smoothing,interpolation,semi-supervised learning,label propagation,Newton's method,iteratively reweighted least square
AI 理解论文
溯源树
样例
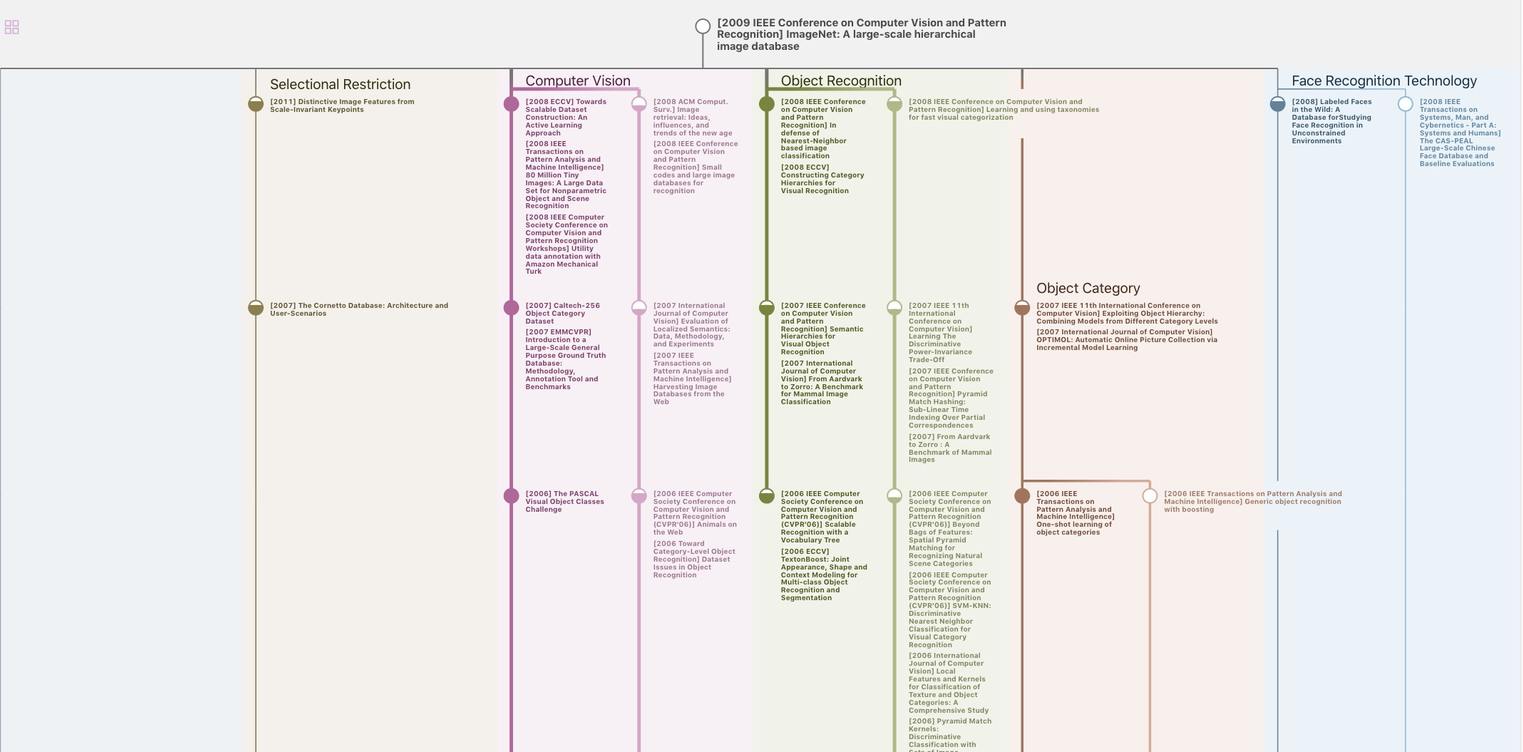
生成溯源树,研究论文发展脉络
Chat Paper
正在生成论文摘要