Cost-Effective Variational Active Entity Resolution
2021 IEEE 37TH INTERNATIONAL CONFERENCE ON DATA ENGINEERING (ICDE 2021)(2021)
摘要
Accurately identifying different representations of the same real-world entity is an integral part of data cleaning and many methods have been proposed to accomplish it. The challenges of this entity resolution task that demand so much research attention are often rooted in the task-specificity and user-dependence of the process. Adopting deep learning techniques has the potential to lessen these challenges. In this paper, we set out to devise an entity resolution method that builds on the robustness conferred by deep autoencoders to reduce human-involvement costs. Specifically, we reduce the cost of training deep entity resolution models by performing unsupervised representation learning. This unveils a transferability property of the resulting model that can further reduce the cost of applying the approach to new datasets by means of transfer learning. Finally, we reduce the cost of labeling training data through an active learning approach that builds on the properties conferred by the use of deep autoencoders. Empirical evaluation confirms the accomplishment of our cost-reduction desideratum, while achieving comparable effectiveness with state-of-the-art alternatives.
更多查看译文
关键词
cost-effective
AI 理解论文
溯源树
样例
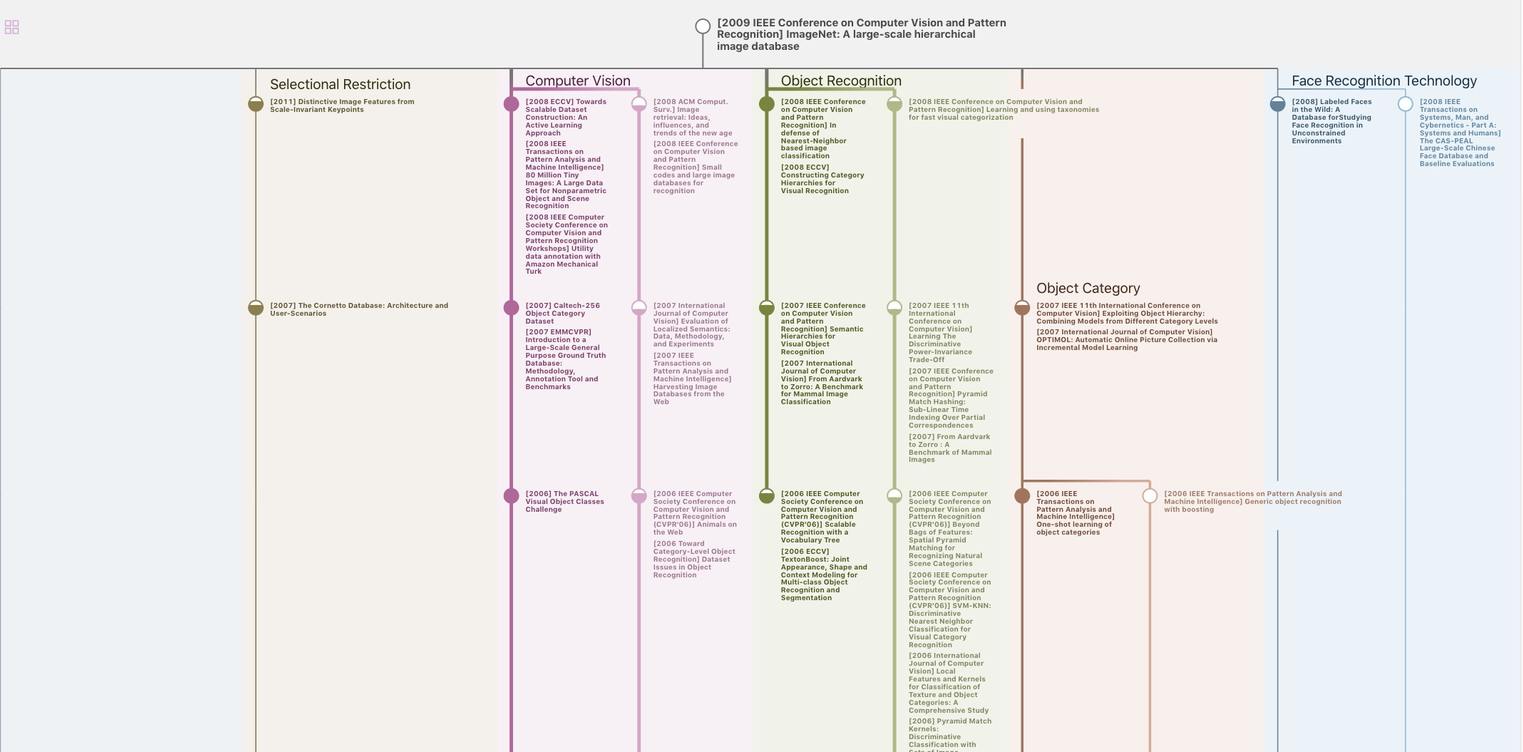
生成溯源树,研究论文发展脉络
Chat Paper
正在生成论文摘要