SLADE: A Self-Training Framework For Distance Metric Learning
2021 IEEE/CVF CONFERENCE ON COMPUTER VISION AND PATTERN RECOGNITION, CVPR 2021(2021)
摘要
Most existing distance metric learning approaches use fully labeled data to learn the sample similarities in an embedding space. We present a self-training framework, SLADE, to improve retrieval performance by leveraging additional unlabeled data. We first train a teacher model on the labeled data and use it to generate pseudo labels for the unlabeled data. We then train a student model on both labels and pseudo labels to generate final feature embeddings. We use self-supervised representation learning to initialize the teacher model. To better deal with noisy pseudo labels generated by the teacher network, we design a new feature basis learning component for the student network, which learns basis functions of feature representations for unlabeled data. The learned basis vectors better measure the pairwise similarity and are used to select high-confident samples for training the student network. We evaluate our method on standard retrieval benchmarks: CUB-200, Cars196 and In-shop. Experimental results demonstrate that with additional unlabeled data, our approach significantly improves the performance over the state-of-the-art methods.
更多查看译文
关键词
SLADE,self-training framework,distance metric learning approaches,fully labeled data,sample similarities,embedding space,retrieval performance,unlabeled data,teacher model,student model,feature embeddings,self-supervised representation,noisy pseudolabels,teacher network,feature basis learning component,student network,feature representations,learned basis vectors,pairwise similarity,standard retrieval benchmarks
AI 理解论文
溯源树
样例
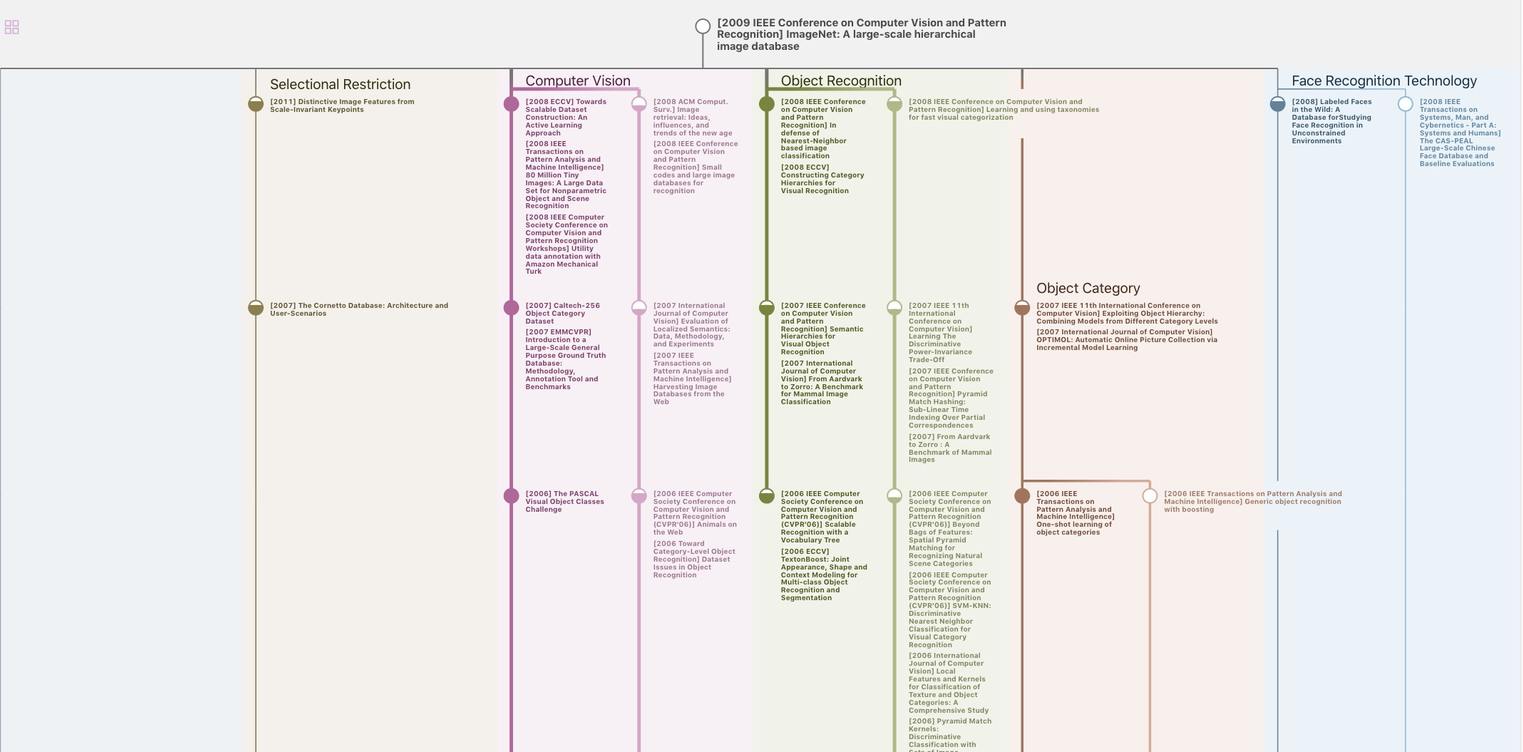
生成溯源树,研究论文发展脉络
Chat Paper
正在生成论文摘要